Repository: incubator-s2graph Updated Branches: refs/heads/master 33e3d267e -> f0f1081b1
add REAME.md to explaining movielens example. Project: http://git-wip-us.apache.org/repos/asf/incubator-s2graph/repo Commit: http://git-wip-us.apache.org/repos/asf/incubator-s2graph/commit/d587538d Tree: http://git-wip-us.apache.org/repos/asf/incubator-s2graph/tree/d587538d Diff: http://git-wip-us.apache.org/repos/asf/incubator-s2graph/diff/d587538d Branch: refs/heads/master Commit: d587538d61c2add23c241f832e39d6ca739ed979 Parents: 33e3d26 Author: DO YUNG YOON <steams...@apache.org> Authored: Tue May 15 16:07:07 2018 +0900 Committer: DO YUNG YOON <steams...@apache.org> Committed: Tue May 15 19:06:01 2018 +0900 ---------------------------------------------------------------------- example/movielens/README.md | 453 +++++++++++++++++++++++++++++++++++++++ 1 file changed, 453 insertions(+) ---------------------------------------------------------------------- http://git-wip-us.apache.org/repos/asf/incubator-s2graph/blob/d587538d/example/movielens/README.md ---------------------------------------------------------------------- diff --git a/example/movielens/README.md b/example/movielens/README.md new file mode 100644 index 0000000..04bfaf7 --- /dev/null +++ b/example/movielens/README.md @@ -0,0 +1,453 @@ +<!--- +/* + * Licensed to the Apache Software Foundation (ASF) under one + * or more contributor license agreements. See the NOTICE file + * distributed with this work for additional information + * regarding copyright ownership. The ASF licenses this file + * to you under the Apache License, Version 2.0 (the + * "License"); you may not use this file except in compliance + * with the License. You may obtain a copy of the License at + * + * http://www.apache.org/licenses/LICENSE-2.0 + * + * Unless required by applicable law or agreed to in writing, + * software distributed under the License is distributed on an + * "AS IS" BASIS, WITHOUT WARRANTIES OR CONDITIONS OF ANY + * KIND, either express or implied. See the License for the + * specific language governing permissions and limitations + * under the License. + */ +---> + +# Movie Recommendation with Apache S2Graph(incubating) And Spark MLLib + +We will briefly go through the example of building movie recommendation service using the public dataset from Movielens. + +There are plenty of materials on the collaborative filtering algorithm and process to build recommendation dataset, +so we will focus on how to integrate your trained machine learning model with property graph model. + + --------- + +## The technologies we'll use + ### [Apache S2Graph](https://s2graph.apache.org/) + +The graph database that stores all movielens dataset. Also, S2Graph provide S2GraphQL which is unified REST Interface for not only graph query, but also serving trained model. + +### [Apache Spark](https://spark.apache.org/) + We process movielens dataset with Apache Spark and most importantly, Apache Spark's MLLib is used to build the model by training movielens data. + +### [Annoy4s](https://github.com/annoy4s/annoy4s) + +After Spark build model by running ALS algorithm, use annoy4s to build the index to find approximate nearest neighbors. + +## The architecture + +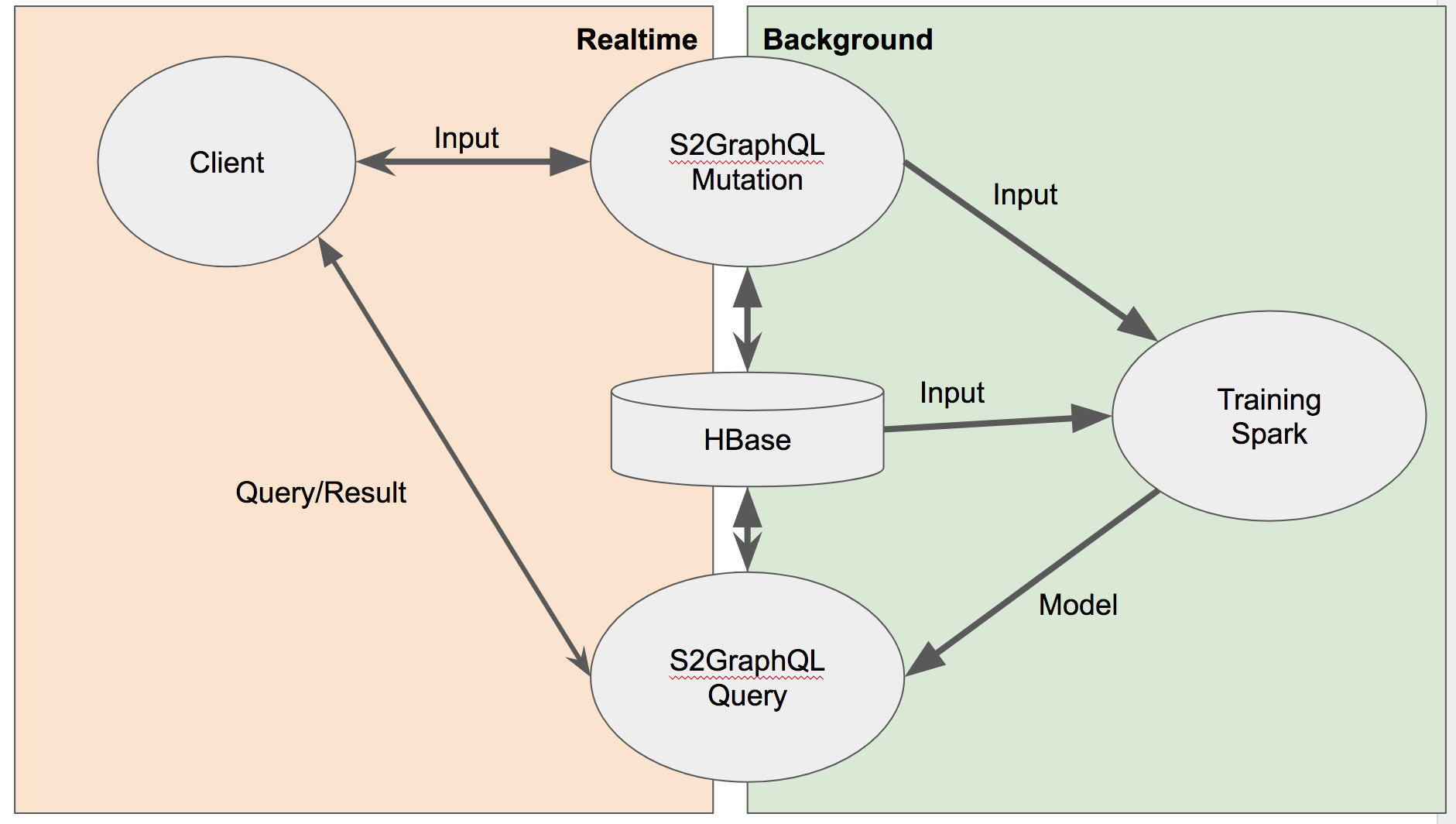 + + +This example will set up local HBase, local Spark, local S2GraphQL server as the environment, and use [graphiql](https://github.com/graphql/graphiql) as the client. + +## The abstraction + +Followings are the representation of movielens dataset as property graph model. + +### 1. Service + +Service represent namespace or database for this example. In this example, we will use movielens as service and all schema and data will be under this namespace. + +```graphql +mutation{ + Management{ + createService( + name:"movielens" + ){ + isSuccess + message + object{ + id + name + } + } + } +} + ``` + +### 2. Vertex Schema + +Represent Node in movielens dataset. Each Node can store multiple properties on it if properties are configured on vertex schema. +Schemas must be registered under service correctly to mutate and query actual vertex/edge from S2Graph. + +#### 2.1. Movie + +Data is under `movies.csv` file and followings are an example of data. + +``` +movieId,title,genres +1,Toy Story (1995),Adventure|Animation|Children|Comedy|Fantasy +2,Jumanji (1995),Adventure|Children|Fantasy +3,Grumpier Old Men (1995),Comedy|Romance +... +``` + +Following is mutation defined as S2GraphQL. + +```graphql +mutation{ + Management{ + createServiceColumn( + serviceName:movielens + columnName:"Movie" + columnType: long + props: [ + { + name: "title" + dataType: string + defaultValue: "" + storeInGlobalIndex: true + }, + { + name: "genres" + dataType: string + defaultValue: "" + storeInGlobalIndex: true + } + ] + ){ + isSuccess + message + object{ + id + name + } + } + } +} +``` + +Note that S2Graph use **user provided id**, which is usually primary key in RDBMS, as vertexId. +S2Graph guarantee the uniqueness of vertexId by using composite of (service, serviceColumn, vertexId). + +Also, note that "storeInGlobalIndex" which let S2Graph build the global index on "title" property. +When the user does not know vertexId in advance and still want to start graph query on vertices that meet certain search criteria, then this global index can be helpful. + +#### 2.2 User + +In the real world, User vertex can have various property, such as age, gender, occupation, location, etc, but in movielens dataset, userId is only available. + +```graphql +mutation{ + Management{ + createServiceColumn( + serviceName:movielens + columnName:"User" + columnType: long + ){ + isSuccess + message + object{ + id + name + } + } + } +} +``` + +### 3. Edge + +Once we create vertex schema for Movie and User, it is time to create edge schema to model the relation between User and Movie. + +#### 3.1. rated + +The data is under `ratings.csv` file and this data represent which user rated which movie. + +``` +userId,movieId,rating,timestamp +1,31,2.5,1260759144 +1,1029,3.0,1260759179 +1,1061,3.0,1260759182 +... +``` + +```graphql +mutation{ + Management{ + createLabel( + name:"rated" + sourceService: { + movielens: { + columnName: User + } + } + targetService: { + movielens: { + columnName: Movie + } + } + serviceName: movielens + consistencyLevel: strong + props:[ + { + name: "score" + dataType: double + defaultValue: "0.0" + storeInGlobalIndex: true + } + ] + indices:{ + name:"_PK" + propNames:["score"] + } + ) { + isSuccess + message + object{ + id + name + props{ + name + } + } + } + } +} +``` + +Since S2Graph support vertex-centric index, which is specific to a vertex, we create primary vertex-centric index "_PK" to be sorted by their score. + +#### 3.2. tagged + +`tags.csv` file contains following data. + +``` +userId,movieId,tag,timestamp +15,339,sandra 'boring' bullock,1138537770 +15,1955,dentist,1193435061 +... +``` + +```graphql +mutation{ + Management{ + createLabel( + name:"tagged" + sourceService: { + movielens: { + columnName: User + } + } + targetService: { + movielens: { + columnName: Movie + } + } + serviceName: movielens + consistencyLevel: weak + props:[ + { + name: "tag" + dataType: string + defaultValue: "" + storeInGlobalIndex: true + } + ] + ) { + isSuccess + message + object{ + id + name + props{ + name + } + } + } + } +} +``` + +#### 3.3. similar_movie +This represents similar movie relation, which actually not stored in S2Graph, but obtained by asking ALS model. +Since S2Graph provide pluggable interface how to fetch/mutate from storage, it is possible to provide the custom model implementation. +[S2GRAPH-206](https://issues.apache.org/jira/projects/S2GRAPH/issues/S2GRAPH-206?filter=allopenissues) issue contains few popular implementations on this interface, such as Annoy, FastText, TensorFlow. + +```graphql +mutation{ + Management{ + createLabel( + name:"similar_movie" + sourceService: { + movielens: { + columnName: Movie + } + } + targetService: { + movielens: { + columnName: Movie + } + } + serviceName: movielens + consistencyLevel: strong + props:[ + { + name: "score" + dataType: double + defaultValue: "0.0" + storeInGlobalIndex: false + } + ] + indices:{ + name:"_PK" + propNames:["score"] + } + ) { + isSuccess + message + object{ + id + name + props{ + name + } + } + } + } +} +``` + +Note that there are no actual edges exist in the S2Graph system, but S2Graph knows which model to ask when user query "similar_movie" edges. +Also note that instead of considering entire ALS model, we use Annoy to support k approximate nearest neighbor search to make prediction fast. + +### Schema Summary + + + +-------------- + +## Running this example + +### Setup + +1. checkout [apache s2graph master](https://github.com/apache/incubator-s2graph) on local. +2. install [apache spark](https://spark.apache.org/downloads.html)( >= v2.2.0) on local. +3. export **SPARK_HOME** to pointing to installed spark. +4. `cd example; sh run.sh` + +### Description + +#### 1. Prepare + +Prepare all pre-requisites to run this example. + +- S2GraphQL server start + - package S2Graph. + - start standalone hbase. + - start s2graphql server on localhost port 8000. + - conf located under `target/apache-s2graph-*-incubating-bin/conf/` + - s2graphql log located under `target/apache-s2graph-*-incubating-bin/log/` + +- S2Jobs jar build + - create fat jar using `sbt project/s2jobs assembly` + - fat jar located under `s2jobs/target/scala-2.11/` + +- check SPARK_HOME is setup correctly + + +#### 2. Create Schema + +- download movielens dataset(ml-latest-small.zip) under `example/movielens/input/` +- create all schema that explained above by sending mutation request to s2graphql server. + +#### 3. Import Data + +- load movielens data as vertices and edges into S2Graph. +- train ALS model on `ratings.csv`. +- build annoy index from dense matrix `itemFactors` in trained ALS model. + +#### 4. Post Process + +- Bind trained annoy index from 3 to "similar_movie" edge schema by update "similar_movie". + +#### 5. Have fun with GraphQL +- go to [graphiql](localhost:8000) and start traversing movielens graph. + +We provide few example queries that can show how to traverse not only graph data but also serving trained model. + +##### 5.1. Item Based Recommendation. + +This is the very basic kind of item-based collaborative filtering recommendation. +Recommendations are **similar movies to movies that each user rated**. + +Note that we ask our model to find k nearest neighbor on the trained model to get similar_movie. + +```graphql +query { + movielens { + User(id: 1) { + rated { + Movie { + title + similar_movie(limit: 5) { + Movie { + title + } + } + } + } + } + } +} +``` + +##### 5.2. Vertex Property Search. +This shows S2Graph's global index feature, which answer **"movies that contain Toy in their title".** +Note how intuitive the GraphQL syntax represent graph traversal. + +```graphql +query { + movielens { + Movie(search: "title: *Toy*", limit: 5) { + title + tagged(limit: 10) { + User { + id + rated(limit: 5) { + Movie { + title + } + } + } + } + } + } +} +``` + +We can mix **model serving and graph traversal** as follow. + +```graphql +query { + movielens { + Movie(search: "genres: *Comedy* AND title: *1995*", limit: 5) { + title + genres + similar_movie(limit: 5) { + Movie { + title + genres + } + } + } + } +} +``` + +Note that we only need the trained model to traverse "similar_movie" relation. + +## Summary + +We show how to serving not only graph data that is actually stored in the graph database but also data that can be obtained from the pre-trained model. + +In general, S2Graph abstract **the pre-trained model as an immutable graph that can produce vertices/edges** for input vertex. + +By using this abstraction, there is no distinction between model serving and graph data from client side. \ No newline at end of file