[GitHub] lebeg commented on issue #11148: [MXNET-679] Refactor handling BLAS libraries with cmake
lebeg commented on issue #11148: [MXNET-679] Refactor handling BLAS libraries with cmake URL: https://github.com/apache/incubator-mxnet/pull/11148#issuecomment-405467956 @szha Yes, of course. Actually the cake scripts are far from being ready for the switch. This change is just a step for resolving https://github.com/apache/incubator-mxnet/issues/8702 This is an automated message from the Apache Git Service. To respond to the message, please log on GitHub and use the URL above to go to the specific comment. For queries about this service, please contact Infrastructure at: us...@infra.apache.org With regards, Apache Git Services
svn commit: r28166 - /dev/incubator/mxnet/1.2.1/ /release/incubator/mxnet/1.2.1/
Author: anirudh2290 Date: Tue Jul 17 05:42:40 2018 New Revision: 28166 Log: 1.2.1 moving from dev to release repo Added: release/incubator/mxnet/1.2.1/ - copied from r28165, dev/incubator/mxnet/1.2.1/ Removed: dev/incubator/mxnet/1.2.1/
svn commit: r28165 - in /dev/incubator/mxnet/1.2.1: ./ apache-mxnet-src-1.2.1-incubating.tar.gz apache-mxnet-src-1.2.1-incubating.tar.gz.asc apache-mxnet-src-1.2.1-incubating.tar.gz.sha512
Author: anirudh2290 Date: Tue Jul 17 05:41:45 2018 New Revision: 28165 Log: Add 1.2.1 files Added: dev/incubator/mxnet/1.2.1/ dev/incubator/mxnet/1.2.1/apache-mxnet-src-1.2.1-incubating.tar.gz (with props) dev/incubator/mxnet/1.2.1/apache-mxnet-src-1.2.1-incubating.tar.gz.asc dev/incubator/mxnet/1.2.1/apache-mxnet-src-1.2.1-incubating.tar.gz.sha512 Added: dev/incubator/mxnet/1.2.1/apache-mxnet-src-1.2.1-incubating.tar.gz == Binary file - no diff available. Propchange: dev/incubator/mxnet/1.2.1/apache-mxnet-src-1.2.1-incubating.tar.gz -- svn:mime-type = application/octet-stream Added: dev/incubator/mxnet/1.2.1/apache-mxnet-src-1.2.1-incubating.tar.gz.asc == --- dev/incubator/mxnet/1.2.1/apache-mxnet-src-1.2.1-incubating.tar.gz.asc (added) +++ dev/incubator/mxnet/1.2.1/apache-mxnet-src-1.2.1-incubating.tar.gz.asc Tue Jul 17 05:41:45 2018 @@ -0,0 +1,17 @@ +-BEGIN PGP SIGNATURE- +Version: GnuPG v1 + +iQIcBAABAgAGBQJbTYA1AAoJEJBnzI6vVc1tmJMQAI7rD1dZ3Z/ZT4u7zDWDsDd1 +HpOCh7ZAgf4MjOkQyy2lhyaF2KYJdLtQ7BXpJJq3JLl3nzYAM9wRvsVZ+Laj8Snv +C9BGfle3LmbzuFr7Dnn1y34jQdweQPUpk6ns9MUTrpmnUReY1h8449JkBCp12U+h +w2LEOzw5APaDJlpuuEh60y+58Kd+i13QBGR/prQ8junojwhHnZdVGu6bdI5Vc0bU +F9FljywNPwZfxaaF/iBw9kKM83E3CPdD4gar3fulDIrwZ/aegz4g3s/L3TAuKMhh +OnY+4iDeeEr4DbOICMa96TFl0EKm2dFR83A5yzdFdqa+xAKW1ZFiEH2Mrt7CA02R +epl3kTALfw2MAdBcc1+sgT0fFfklef6uh9AyokzDo0FX/s6cv3eMjnwyYl2lLG8n +XBuO4mRfRXC7YHJafeyFIwq0h7t6wXdtkXtFvM9qvGrQ+TtW1hjJtvXXmfTgciaI +gxxWdjnwwA0gzWFcdApdlpn2VNy/GdVFXCfglafk3EVxQF4ndKFzaPaWhE4ZuQDn +iGlagmtbccKJ4RrYZxfnwh42U2tr/+HM2LX3LIrgeR9j9BA4I9/0/O0541xuuLkA +IV/6n9X++z4Py9k0RyTAA6saaYijcnE7K1l532BvSQee8bMr+N50JBBK6bGUXItu +8yTSC6z3xMPr4cfmh40r +=JItz +-END PGP SIGNATURE- Added: dev/incubator/mxnet/1.2.1/apache-mxnet-src-1.2.1-incubating.tar.gz.sha512 == --- dev/incubator/mxnet/1.2.1/apache-mxnet-src-1.2.1-incubating.tar.gz.sha512 (added) +++ dev/incubator/mxnet/1.2.1/apache-mxnet-src-1.2.1-incubating.tar.gz.sha512 Tue Jul 17 05:41:45 2018 @@ -0,0 +1 @@ +09eafecaf73284a938c03a0e72ac2b2cfb69f89c44301ca28268a0b01b3b4d7da3cf3a404535a64b9beef5116851815aee15bbfa106a4ed66186064df7d7f35b apache-mxnet-src-1.2.1-incubating.tar.gz
[GitHub] safrooze commented on a change in pull request #11651: Add logistic regression tutorial
safrooze commented on a change in pull request #11651: Add logistic regression tutorial URL: https://github.com/apache/incubator-mxnet/pull/11651#discussion_r202895654 ## File path: docs/tutorials/gluon/logistic_regression_explained.md ## @@ -0,0 +1,215 @@ + +# Logistic regression using Gluon API explained + +Logistic Regression is one of the first models newcomers to Deep Learning are implementing. In this tutorial I am going to focus on how to do logistic regression using Gluon API and provide some high level tips. + +Before anything else, let's import required packages for this tutorial. + + +```python +import numpy as np +import mxnet as mx +from mxnet import nd, autograd, gluon +from mxnet.gluon import nn, Trainer +from mxnet.gluon.data import DataLoader, ArrayDataset + +mx.random.seed(12345) # Added for reproducibility +``` + +In this tutorial we will use fake dataset, which contains 10 features drawn from a normal distribution with mean equals to 0 and standard deviation equals to 1, and a class label, which can be either 0 or 1. The length of the dataset is an arbitrary value. The function below helps us to generate a dataset. + + +```python +def get_random_data(size, ctx): +x = nd.normal(0, 1, shape=(size, 10), ctx=ctx) +# Class label is generated via non-random logic so the network would have a pattern to look for +# Number 3 is selected to make sure that number of positive examples smaller than negative, but not too small +y = x.sum(axis=1) > 3 +return x, y +``` + +Also, let's define a set of hyperparameters, that we are going to use later. Since our model is simple and dataset is small, we are going to use CPU for calculations. Feel free to change it to GPU for a more advanced scenario. + + +```python +ctx = mx.cpu() +train_data_size = 1000 +val_data_size = 100 +batch_size = 10 +``` + +## Working with data + +To work with data, Apache MXNet provides Dataset and DataLoader classes. The former is used to provide an indexed access to the data, the latter is used to shuffle and batchify the data. + +This separation is done because a source of Dataset can vary from a simple array of numbers to complex data structures like text and images. DataLoader doesn't need to be aware of the source of data as long as Dataset provides a way to get the number of records and to load a record by index. As an outcome, Dataset doesn't need to hold in memory all data at once. Needless to say, that one can implement its own versions of Dataset and DataLoader, but we are going to use existing implementation. + +Below we define 2 datasets: training dataset and validation dataset. It is a good practice to measure performance of a trained model on a data that the network hasn't seen before. That is why we are going to use training set for training the model and validation set to calculate model's accuracy. + + +```python +train_x, train_ground_truth_class = get_random_data(train_data_size, ctx) +train_dataset = ArrayDataset(train_x, train_ground_truth_class) +train_dataloader = DataLoader(train_dataset, batch_size=batch_size, shuffle=True) + +val_x, val_ground_truth_class = get_random_data(val_data_size, ctx) +val_dataset = ArrayDataset(val_x, val_ground_truth_class) +val_dataloader = DataLoader(val_dataset, batch_size=batch_size, shuffle=True) +``` + +## Defining and training the model + +In real application, model can be arbitrary complex. The only requirement for the logistic regression is that the last layer of the network must be a single neuron. Apache MXNet allows us to do so by using `Dense` layer and specifying the number of units to 1. + +Below, we define a model which has an input layer of 10 neurons, a couple inner layers of 10 neurons each, and output layer of 1 neuron, as it is required by logistic regression. We stack the layers using `HybridSequential` block and initialize parameters of the network using `Xavier` initialization. + + +```python +net = nn.HybridSequential() + +with net.name_scope(): +net.add(nn.Dense(units=10, activation='relu')) # input layer +net.add(nn.Dense(units=10, activation='relu')) # inner layer 1 +net.add(nn.Dense(units=10, activation='relu')) # inner layer 2 +net.add(nn.Dense(units=1)) # output layer: notice, it must have only 1 neuron + +net.initialize(mx.init.Xavier(magnitude=2.34)) +``` + +After defining the model, we need to define a few more thing: our loss, our trainer and our metric. + +Loss function is used to calculate how the output of the network different from the ground truth. In case of the logistic regression the ground truth are class labels, which can be either 0 or 1. Because of that, we are using `SigmoidBinaryCrossEntropyLoss`, which suites well for that scenario. + +Trainer object allows to specify the method of training to be used. There are various methods available, and for our tutorial we use a widely accepted method Stochastic Gradient Descent. We
[GitHub] safrooze commented on a change in pull request #11651: Add logistic regression tutorial
safrooze commented on a change in pull request #11651: Add logistic regression tutorial URL: https://github.com/apache/incubator-mxnet/pull/11651#discussion_r202895482 ## File path: docs/tutorials/gluon/logistic_regression_explained.md ## @@ -0,0 +1,215 @@ + +# Logistic regression using Gluon API explained + +Logistic Regression is one of the first models newcomers to Deep Learning are implementing. In this tutorial I am going to focus on how to do logistic regression using Gluon API and provide some high level tips. + +Before anything else, let's import required packages for this tutorial. + + +```python +import numpy as np +import mxnet as mx +from mxnet import nd, autograd, gluon +from mxnet.gluon import nn, Trainer +from mxnet.gluon.data import DataLoader, ArrayDataset + +mx.random.seed(12345) # Added for reproducibility +``` + +In this tutorial we will use fake dataset, which contains 10 features drawn from a normal distribution with mean equals to 0 and standard deviation equals to 1, and a class label, which can be either 0 or 1. The length of the dataset is an arbitrary value. The function below helps us to generate a dataset. + + +```python +def get_random_data(size, ctx): +x = nd.normal(0, 1, shape=(size, 10), ctx=ctx) +# Class label is generated via non-random logic so the network would have a pattern to look for +# Number 3 is selected to make sure that number of positive examples smaller than negative, but not too small +y = x.sum(axis=1) > 3 +return x, y +``` + +Also, let's define a set of hyperparameters, that we are going to use later. Since our model is simple and dataset is small, we are going to use CPU for calculations. Feel free to change it to GPU for a more advanced scenario. + + +```python +ctx = mx.cpu() +train_data_size = 1000 +val_data_size = 100 +batch_size = 10 +``` + +## Working with data + +To work with data, Apache MXNet provides Dataset and DataLoader classes. The former is used to provide an indexed access to the data, the latter is used to shuffle and batchify the data. + +This separation is done because a source of Dataset can vary from a simple array of numbers to complex data structures like text and images. DataLoader doesn't need to be aware of the source of data as long as Dataset provides a way to get the number of records and to load a record by index. As an outcome, Dataset doesn't need to hold in memory all data at once. Needless to say, that one can implement its own versions of Dataset and DataLoader, but we are going to use existing implementation. + +Below we define 2 datasets: training dataset and validation dataset. It is a good practice to measure performance of a trained model on a data that the network hasn't seen before. That is why we are going to use training set for training the model and validation set to calculate model's accuracy. + + +```python +train_x, train_ground_truth_class = get_random_data(train_data_size, ctx) +train_dataset = ArrayDataset(train_x, train_ground_truth_class) +train_dataloader = DataLoader(train_dataset, batch_size=batch_size, shuffle=True) + +val_x, val_ground_truth_class = get_random_data(val_data_size, ctx) +val_dataset = ArrayDataset(val_x, val_ground_truth_class) +val_dataloader = DataLoader(val_dataset, batch_size=batch_size, shuffle=True) +``` + +## Defining and training the model + +In real application, model can be arbitrary complex. The only requirement for the logistic regression is that the last layer of the network must be a single neuron. Apache MXNet allows us to do so by using `Dense` layer and specifying the number of units to 1. + +Below, we define a model which has an input layer of 10 neurons, a couple inner layers of 10 neurons each, and output layer of 1 neuron, as it is required by logistic regression. We stack the layers using `HybridSequential` block and initialize parameters of the network using `Xavier` initialization. + + +```python +net = nn.HybridSequential() + +with net.name_scope(): +net.add(nn.Dense(units=10, activation='relu')) # input layer +net.add(nn.Dense(units=10, activation='relu')) # inner layer 1 +net.add(nn.Dense(units=10, activation='relu')) # inner layer 2 +net.add(nn.Dense(units=1)) # output layer: notice, it must have only 1 neuron + +net.initialize(mx.init.Xavier(magnitude=2.34)) +``` + +After defining the model, we need to define a few more thing: our loss, our trainer and our metric. + +Loss function is used to calculate how the output of the network different from the ground truth. In case of the logistic regression the ground truth are class labels, which can be either 0 or 1. Because of that, we are using `SigmoidBinaryCrossEntropyLoss`, which suites well for that scenario. + +Trainer object allows to specify the method of training to be used. There are various methods available, and for our tutorial we use a widely accepted method Stochastic Gradient Descent. We
[GitHub] szha commented on a change in pull request #11528: New ecosystem website page
szha commented on a change in pull request #11528: New ecosystem website page URL: https://github.com/apache/incubator-mxnet/pull/11528#discussion_r202898732 ## File path: docs/community/ecosystem.md ## @@ -0,0 +1,72 @@ +# MXNet's Ecosystem + +Community contributions to MXNet have added many new valuable features and functionality to support use cases such as model serving & portability, easy and flexible APIs, and educational material like crash courses and online books. This ecosystem page lists the projects that use MXNet, teach MXNet, or augment MXNet in some way. + + +## Highlighted Project + +[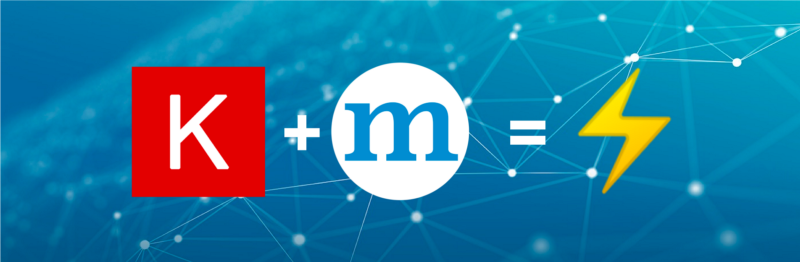](https://medium.com/apache-mxnet/announcing-keras-mxnet-v2-2-4b8404568e75) + [Keras-MXNet v2.2 released!](https://medium.com/apache-mxnet/announcing-keras-mxnet-v2-2-4b8404568e75) + + +## Contents + +* [Learning MXNet and other Educational Resources](#learning-mxnet-and-other-educational-resources) +* [MXNet APIs](#mxnet-apis) +* [Toolkits to Extend MXNet](#toolkits-to-extend-mxnet) +* [Debugging and Visualization](#debugging-and-visualization) +* [Model Serving](#model-serving) +* [Model Zoos](#model-zoos) +* [Contributions](#contributions) + + +## Learning MXNet and other Educational Resources + +* [Gluon 60 Minute Crash Course](https://gluon-crash-course.mxnet.io/) - deep learning practitioners can learn Gluon quickly with these six 10-minute tutorials. +- [YouTube Series](https://www.youtube.com/playlist?list=PLkEvNnRk8uVmVKRDgznk3o3LxmjFRaW7s) +* [The Straight Dope](https://gluon.mxnet.io/) - a series of notebooks designed to teach deep learning using the Gluon Python API for MXNet. + + +## MXNet APIs + +* [Clojure API](https://github.com/apache/incubator-mxnet/tree/master/contrib/clojure-package) - use MXNet with Clojure. +* [C++ API](../api/c++/index.html) - not be confused with the C++ backend, this API allows C++ programmers to train networks in C++. +* [Gluon Python Interface](../gluon/index.html) - train complex models imperatively and then deploy with a symbolic graph. +* [Julia API](../api/julia/index.html) *(Community Supported)* - train models with multiple GPUs using Julia. +* [Keras-MXNet](https://github.com/awslabs/keras-apache-mxnet) - design with Keras2 and train with MXNet as the backend for 2x or more speed improvement. +* [MinPy](https://github.com/dmlc/minpy) - Pure numpy practice with third party operator integration and MXNet as backend for GPU computing +* [Module Python API](../api/python/index.html) - backed by the Symbol API, you can define your network in a declarative fashion. +* [ONNX-MXnet API](../api/python/contrib/onnx.html) - train and use Open Neural Network eXchange (ONNX) model files. +* [Perl API](../api/perl/index.html) *(Community Supported)* - train models with multiple GPUs using Perl. +* [R API](https://mxnet.incubator.apache.org/api/r/index.html) *(Community Supported)* - train models with multiple GPUs using R. +* [Scala Infer API](../api/scala/infer.html) - model loading and inference functionality. +* [TensorFuse](https://github.com/dementrock/tensorfuse) - Common interface for Theano, CGT, TensorFlow, and MXNet (experimental) by [dementrock](https://github.com/dementrock) + + +## Toolkits to Extend MXNet + +* [NVIDIA DALI](https://github.com/NVIDIA/DALI/tree/master/examples/mxnet) - a collection of highly optimized building blocks and an execution engine to accelerate input data pre-processing for deep learning applications. Review comment: It's not specifically built for mxnet, even though it has examples for using in mxnet. Examples in the same line of argument is that we don't include numpy, skimage, PIL, or opencv here. This is an automated message from the Apache Git Service. To respond to the message, please log on GitHub and use the URL above to go to the specific comment. For queries about this service, please contact Infrastructure at: us...@infra.apache.org With regards, Apache Git Services
[GitHub] lupesko commented on a change in pull request #11528: New ecosystem website page
lupesko commented on a change in pull request #11528: New ecosystem website page URL: https://github.com/apache/incubator-mxnet/pull/11528#discussion_r202898348 ## File path: example/model-server/mms.md ## @@ -0,0 +1,115 @@ +# Model Server for Apache MXNet (incubating) Review comment: I tend to agree with @szha - isn't it enough to link to MMS repo readme (or to modelserver.io) from ecosystem.md? Duplicating docs means more effort to maintain, keep up to date, etc. This is an automated message from the Apache Git Service. To respond to the message, please log on GitHub and use the URL above to go to the specific comment. For queries about this service, please contact Infrastructure at: us...@infra.apache.org With regards, Apache Git Services
[GitHub] safrooze commented on a change in pull request #11651: Add logistic regression tutorial
safrooze commented on a change in pull request #11651: Add logistic regression tutorial URL: https://github.com/apache/incubator-mxnet/pull/11651#discussion_r202895708 ## File path: docs/tutorials/gluon/logistic_regression_explained.md ## @@ -0,0 +1,215 @@ + +# Logistic regression using Gluon API explained + +Logistic Regression is one of the first models newcomers to Deep Learning are implementing. In this tutorial I am going to focus on how to do logistic regression using Gluon API and provide some high level tips. + +Before anything else, let's import required packages for this tutorial. + + +```python +import numpy as np +import mxnet as mx +from mxnet import nd, autograd, gluon +from mxnet.gluon import nn, Trainer +from mxnet.gluon.data import DataLoader, ArrayDataset + +mx.random.seed(12345) # Added for reproducibility +``` + +In this tutorial we will use fake dataset, which contains 10 features drawn from a normal distribution with mean equals to 0 and standard deviation equals to 1, and a class label, which can be either 0 or 1. The length of the dataset is an arbitrary value. The function below helps us to generate a dataset. + + +```python +def get_random_data(size, ctx): +x = nd.normal(0, 1, shape=(size, 10), ctx=ctx) +# Class label is generated via non-random logic so the network would have a pattern to look for +# Number 3 is selected to make sure that number of positive examples smaller than negative, but not too small +y = x.sum(axis=1) > 3 +return x, y +``` + +Also, let's define a set of hyperparameters, that we are going to use later. Since our model is simple and dataset is small, we are going to use CPU for calculations. Feel free to change it to GPU for a more advanced scenario. + + +```python +ctx = mx.cpu() +train_data_size = 1000 +val_data_size = 100 +batch_size = 10 +``` + +## Working with data + +To work with data, Apache MXNet provides Dataset and DataLoader classes. The former is used to provide an indexed access to the data, the latter is used to shuffle and batchify the data. + +This separation is done because a source of Dataset can vary from a simple array of numbers to complex data structures like text and images. DataLoader doesn't need to be aware of the source of data as long as Dataset provides a way to get the number of records and to load a record by index. As an outcome, Dataset doesn't need to hold in memory all data at once. Needless to say, that one can implement its own versions of Dataset and DataLoader, but we are going to use existing implementation. + +Below we define 2 datasets: training dataset and validation dataset. It is a good practice to measure performance of a trained model on a data that the network hasn't seen before. That is why we are going to use training set for training the model and validation set to calculate model's accuracy. + + +```python +train_x, train_ground_truth_class = get_random_data(train_data_size, ctx) +train_dataset = ArrayDataset(train_x, train_ground_truth_class) +train_dataloader = DataLoader(train_dataset, batch_size=batch_size, shuffle=True) + +val_x, val_ground_truth_class = get_random_data(val_data_size, ctx) +val_dataset = ArrayDataset(val_x, val_ground_truth_class) +val_dataloader = DataLoader(val_dataset, batch_size=batch_size, shuffle=True) +``` + +## Defining and training the model + +In real application, model can be arbitrary complex. The only requirement for the logistic regression is that the last layer of the network must be a single neuron. Apache MXNet allows us to do so by using `Dense` layer and specifying the number of units to 1. + +Below, we define a model which has an input layer of 10 neurons, a couple inner layers of 10 neurons each, and output layer of 1 neuron, as it is required by logistic regression. We stack the layers using `HybridSequential` block and initialize parameters of the network using `Xavier` initialization. + + +```python +net = nn.HybridSequential() + +with net.name_scope(): +net.add(nn.Dense(units=10, activation='relu')) # input layer +net.add(nn.Dense(units=10, activation='relu')) # inner layer 1 +net.add(nn.Dense(units=10, activation='relu')) # inner layer 2 +net.add(nn.Dense(units=1)) # output layer: notice, it must have only 1 neuron + +net.initialize(mx.init.Xavier(magnitude=2.34)) +``` + +After defining the model, we need to define a few more thing: our loss, our trainer and our metric. + +Loss function is used to calculate how the output of the network different from the ground truth. In case of the logistic regression the ground truth are class labels, which can be either 0 or 1. Because of that, we are using `SigmoidBinaryCrossEntropyLoss`, which suites well for that scenario. + +Trainer object allows to specify the method of training to be used. There are various methods available, and for our tutorial we use a widely accepted method Stochastic Gradient Descent. We
[GitHub] safrooze commented on a change in pull request #11651: Add logistic regression tutorial
safrooze commented on a change in pull request #11651: Add logistic regression tutorial URL: https://github.com/apache/incubator-mxnet/pull/11651#discussion_r202897885 ## File path: docs/tutorials/gluon/logistic_regression_explained.md ## @@ -0,0 +1,215 @@ + +# Logistic regression using Gluon API explained + +Logistic Regression is one of the first models newcomers to Deep Learning are implementing. In this tutorial I am going to focus on how to do logistic regression using Gluon API and provide some high level tips. + +Before anything else, let's import required packages for this tutorial. + + +```python +import numpy as np +import mxnet as mx +from mxnet import nd, autograd, gluon +from mxnet.gluon import nn, Trainer +from mxnet.gluon.data import DataLoader, ArrayDataset + +mx.random.seed(12345) # Added for reproducibility +``` + +In this tutorial we will use fake dataset, which contains 10 features drawn from a normal distribution with mean equals to 0 and standard deviation equals to 1, and a class label, which can be either 0 or 1. The length of the dataset is an arbitrary value. The function below helps us to generate a dataset. + + +```python +def get_random_data(size, ctx): +x = nd.normal(0, 1, shape=(size, 10), ctx=ctx) +# Class label is generated via non-random logic so the network would have a pattern to look for +# Number 3 is selected to make sure that number of positive examples smaller than negative, but not too small +y = x.sum(axis=1) > 3 +return x, y +``` + +Also, let's define a set of hyperparameters, that we are going to use later. Since our model is simple and dataset is small, we are going to use CPU for calculations. Feel free to change it to GPU for a more advanced scenario. + + +```python +ctx = mx.cpu() +train_data_size = 1000 +val_data_size = 100 +batch_size = 10 +``` + +## Working with data + +To work with data, Apache MXNet provides Dataset and DataLoader classes. The former is used to provide an indexed access to the data, the latter is used to shuffle and batchify the data. + +This separation is done because a source of Dataset can vary from a simple array of numbers to complex data structures like text and images. DataLoader doesn't need to be aware of the source of data as long as Dataset provides a way to get the number of records and to load a record by index. As an outcome, Dataset doesn't need to hold in memory all data at once. Needless to say, that one can implement its own versions of Dataset and DataLoader, but we are going to use existing implementation. + +Below we define 2 datasets: training dataset and validation dataset. It is a good practice to measure performance of a trained model on a data that the network hasn't seen before. That is why we are going to use training set for training the model and validation set to calculate model's accuracy. + + +```python +train_x, train_ground_truth_class = get_random_data(train_data_size, ctx) +train_dataset = ArrayDataset(train_x, train_ground_truth_class) +train_dataloader = DataLoader(train_dataset, batch_size=batch_size, shuffle=True) + +val_x, val_ground_truth_class = get_random_data(val_data_size, ctx) +val_dataset = ArrayDataset(val_x, val_ground_truth_class) +val_dataloader = DataLoader(val_dataset, batch_size=batch_size, shuffle=True) +``` + +## Defining and training the model + +In real application, model can be arbitrary complex. The only requirement for the logistic regression is that the last layer of the network must be a single neuron. Apache MXNet allows us to do so by using `Dense` layer and specifying the number of units to 1. + +Below, we define a model which has an input layer of 10 neurons, a couple inner layers of 10 neurons each, and output layer of 1 neuron, as it is required by logistic regression. We stack the layers using `HybridSequential` block and initialize parameters of the network using `Xavier` initialization. + + +```python +net = nn.HybridSequential() + +with net.name_scope(): +net.add(nn.Dense(units=10, activation='relu')) # input layer +net.add(nn.Dense(units=10, activation='relu')) # inner layer 1 +net.add(nn.Dense(units=10, activation='relu')) # inner layer 2 +net.add(nn.Dense(units=1)) # output layer: notice, it must have only 1 neuron + +net.initialize(mx.init.Xavier(magnitude=2.34)) +``` + +After defining the model, we need to define a few more thing: our loss, our trainer and our metric. + +Loss function is used to calculate how the output of the network different from the ground truth. In case of the logistic regression the ground truth are class labels, which can be either 0 or 1. Because of that, we are using `SigmoidBinaryCrossEntropyLoss`, which suites well for that scenario. + +Trainer object allows to specify the method of training to be used. There are various methods available, and for our tutorial we use a widely accepted method Stochastic Gradient Descent. We
[GitHub] safrooze commented on a change in pull request #11651: Add logistic regression tutorial
safrooze commented on a change in pull request #11651: Add logistic regression tutorial URL: https://github.com/apache/incubator-mxnet/pull/11651#discussion_r202894812 ## File path: docs/tutorials/gluon/logistic_regression_explained.md ## @@ -0,0 +1,215 @@ + +# Logistic regression using Gluon API explained + +Logistic Regression is one of the first models newcomers to Deep Learning are implementing. In this tutorial I am going to focus on how to do logistic regression using Gluon API and provide some high level tips. + +Before anything else, let's import required packages for this tutorial. + + +```python +import numpy as np +import mxnet as mx +from mxnet import nd, autograd, gluon +from mxnet.gluon import nn, Trainer +from mxnet.gluon.data import DataLoader, ArrayDataset + +mx.random.seed(12345) # Added for reproducibility +``` + +In this tutorial we will use fake dataset, which contains 10 features drawn from a normal distribution with mean equals to 0 and standard deviation equals to 1, and a class label, which can be either 0 or 1. The length of the dataset is an arbitrary value. The function below helps us to generate a dataset. + + +```python +def get_random_data(size, ctx): +x = nd.normal(0, 1, shape=(size, 10), ctx=ctx) +# Class label is generated via non-random logic so the network would have a pattern to look for +# Number 3 is selected to make sure that number of positive examples smaller than negative, but not too small +y = x.sum(axis=1) > 3 +return x, y +``` + +Also, let's define a set of hyperparameters, that we are going to use later. Since our model is simple and dataset is small, we are going to use CPU for calculations. Feel free to change it to GPU for a more advanced scenario. + + +```python +ctx = mx.cpu() +train_data_size = 1000 +val_data_size = 100 +batch_size = 10 +``` + +## Working with data + +To work with data, Apache MXNet provides Dataset and DataLoader classes. The former is used to provide an indexed access to the data, the latter is used to shuffle and batchify the data. + +This separation is done because a source of Dataset can vary from a simple array of numbers to complex data structures like text and images. DataLoader doesn't need to be aware of the source of data as long as Dataset provides a way to get the number of records and to load a record by index. As an outcome, Dataset doesn't need to hold in memory all data at once. Needless to say, that one can implement its own versions of Dataset and DataLoader, but we are going to use existing implementation. + +Below we define 2 datasets: training dataset and validation dataset. It is a good practice to measure performance of a trained model on a data that the network hasn't seen before. That is why we are going to use training set for training the model and validation set to calculate model's accuracy. + + +```python +train_x, train_ground_truth_class = get_random_data(train_data_size, ctx) +train_dataset = ArrayDataset(train_x, train_ground_truth_class) +train_dataloader = DataLoader(train_dataset, batch_size=batch_size, shuffle=True) + +val_x, val_ground_truth_class = get_random_data(val_data_size, ctx) +val_dataset = ArrayDataset(val_x, val_ground_truth_class) +val_dataloader = DataLoader(val_dataset, batch_size=batch_size, shuffle=True) +``` + +## Defining and training the model + +In real application, model can be arbitrary complex. The only requirement for the logistic regression is that the last layer of the network must be a single neuron. Apache MXNet allows us to do so by using `Dense` layer and specifying the number of units to 1. + +Below, we define a model which has an input layer of 10 neurons, a couple inner layers of 10 neurons each, and output layer of 1 neuron, as it is required by logistic regression. We stack the layers using `HybridSequential` block and initialize parameters of the network using `Xavier` initialization. + + +```python +net = nn.HybridSequential() + +with net.name_scope(): +net.add(nn.Dense(units=10, activation='relu')) # input layer +net.add(nn.Dense(units=10, activation='relu')) # inner layer 1 +net.add(nn.Dense(units=10, activation='relu')) # inner layer 2 +net.add(nn.Dense(units=1)) # output layer: notice, it must have only 1 neuron + +net.initialize(mx.init.Xavier(magnitude=2.34)) +``` + +After defining the model, we need to define a few more thing: our loss, our trainer and our metric. + +Loss function is used to calculate how the output of the network different from the ground truth. In case of the logistic regression the ground truth are class labels, which can be either 0 or 1. Because of that, we are using `SigmoidBinaryCrossEntropyLoss`, which suites well for that scenario. Review comment: I think if instead of defining what a loss is, you'd define what `SigmoidBinaryCrossEntropyLoss` does, user may benefit more. Something that explains there is a
[GitHub] safrooze commented on a change in pull request #11651: Add logistic regression tutorial
safrooze commented on a change in pull request #11651: Add logistic regression tutorial URL: https://github.com/apache/incubator-mxnet/pull/11651#discussion_r202894975 ## File path: docs/tutorials/gluon/logistic_regression_explained.md ## @@ -0,0 +1,215 @@ + +# Logistic regression using Gluon API explained + +Logistic Regression is one of the first models newcomers to Deep Learning are implementing. In this tutorial I am going to focus on how to do logistic regression using Gluon API and provide some high level tips. + +Before anything else, let's import required packages for this tutorial. + + +```python +import numpy as np +import mxnet as mx +from mxnet import nd, autograd, gluon +from mxnet.gluon import nn, Trainer +from mxnet.gluon.data import DataLoader, ArrayDataset + +mx.random.seed(12345) # Added for reproducibility +``` + +In this tutorial we will use fake dataset, which contains 10 features drawn from a normal distribution with mean equals to 0 and standard deviation equals to 1, and a class label, which can be either 0 or 1. The length of the dataset is an arbitrary value. The function below helps us to generate a dataset. + + +```python +def get_random_data(size, ctx): +x = nd.normal(0, 1, shape=(size, 10), ctx=ctx) +# Class label is generated via non-random logic so the network would have a pattern to look for +# Number 3 is selected to make sure that number of positive examples smaller than negative, but not too small +y = x.sum(axis=1) > 3 +return x, y +``` + +Also, let's define a set of hyperparameters, that we are going to use later. Since our model is simple and dataset is small, we are going to use CPU for calculations. Feel free to change it to GPU for a more advanced scenario. + + +```python +ctx = mx.cpu() +train_data_size = 1000 +val_data_size = 100 +batch_size = 10 +``` + +## Working with data + +To work with data, Apache MXNet provides Dataset and DataLoader classes. The former is used to provide an indexed access to the data, the latter is used to shuffle and batchify the data. + +This separation is done because a source of Dataset can vary from a simple array of numbers to complex data structures like text and images. DataLoader doesn't need to be aware of the source of data as long as Dataset provides a way to get the number of records and to load a record by index. As an outcome, Dataset doesn't need to hold in memory all data at once. Needless to say, that one can implement its own versions of Dataset and DataLoader, but we are going to use existing implementation. + +Below we define 2 datasets: training dataset and validation dataset. It is a good practice to measure performance of a trained model on a data that the network hasn't seen before. That is why we are going to use training set for training the model and validation set to calculate model's accuracy. + + +```python +train_x, train_ground_truth_class = get_random_data(train_data_size, ctx) +train_dataset = ArrayDataset(train_x, train_ground_truth_class) +train_dataloader = DataLoader(train_dataset, batch_size=batch_size, shuffle=True) + +val_x, val_ground_truth_class = get_random_data(val_data_size, ctx) +val_dataset = ArrayDataset(val_x, val_ground_truth_class) +val_dataloader = DataLoader(val_dataset, batch_size=batch_size, shuffle=True) +``` + +## Defining and training the model + +In real application, model can be arbitrary complex. The only requirement for the logistic regression is that the last layer of the network must be a single neuron. Apache MXNet allows us to do so by using `Dense` layer and specifying the number of units to 1. + +Below, we define a model which has an input layer of 10 neurons, a couple inner layers of 10 neurons each, and output layer of 1 neuron, as it is required by logistic regression. We stack the layers using `HybridSequential` block and initialize parameters of the network using `Xavier` initialization. + + +```python +net = nn.HybridSequential() + +with net.name_scope(): +net.add(nn.Dense(units=10, activation='relu')) # input layer +net.add(nn.Dense(units=10, activation='relu')) # inner layer 1 +net.add(nn.Dense(units=10, activation='relu')) # inner layer 2 +net.add(nn.Dense(units=1)) # output layer: notice, it must have only 1 neuron + +net.initialize(mx.init.Xavier(magnitude=2.34)) +``` + +After defining the model, we need to define a few more thing: our loss, our trainer and our metric. + +Loss function is used to calculate how the output of the network different from the ground truth. In case of the logistic regression the ground truth are class labels, which can be either 0 or 1. Because of that, we are using `SigmoidBinaryCrossEntropyLoss`, which suites well for that scenario. + +Trainer object allows to specify the method of training to be used. There are various methods available, and for our tutorial we use a widely accepted method Stochastic Gradient Descent. We
[GitHub] safrooze commented on a change in pull request #11651: Add logistic regression tutorial
safrooze commented on a change in pull request #11651: Add logistic regression tutorial URL: https://github.com/apache/incubator-mxnet/pull/11651#discussion_r202895412 ## File path: docs/tutorials/gluon/logistic_regression_explained.md ## @@ -0,0 +1,215 @@ + +# Logistic regression using Gluon API explained + +Logistic Regression is one of the first models newcomers to Deep Learning are implementing. In this tutorial I am going to focus on how to do logistic regression using Gluon API and provide some high level tips. + +Before anything else, let's import required packages for this tutorial. + + +```python +import numpy as np +import mxnet as mx +from mxnet import nd, autograd, gluon +from mxnet.gluon import nn, Trainer +from mxnet.gluon.data import DataLoader, ArrayDataset + +mx.random.seed(12345) # Added for reproducibility +``` + +In this tutorial we will use fake dataset, which contains 10 features drawn from a normal distribution with mean equals to 0 and standard deviation equals to 1, and a class label, which can be either 0 or 1. The length of the dataset is an arbitrary value. The function below helps us to generate a dataset. + + +```python +def get_random_data(size, ctx): +x = nd.normal(0, 1, shape=(size, 10), ctx=ctx) +# Class label is generated via non-random logic so the network would have a pattern to look for +# Number 3 is selected to make sure that number of positive examples smaller than negative, but not too small +y = x.sum(axis=1) > 3 +return x, y +``` + +Also, let's define a set of hyperparameters, that we are going to use later. Since our model is simple and dataset is small, we are going to use CPU for calculations. Feel free to change it to GPU for a more advanced scenario. + + +```python +ctx = mx.cpu() +train_data_size = 1000 +val_data_size = 100 +batch_size = 10 +``` + +## Working with data + +To work with data, Apache MXNet provides Dataset and DataLoader classes. The former is used to provide an indexed access to the data, the latter is used to shuffle and batchify the data. + +This separation is done because a source of Dataset can vary from a simple array of numbers to complex data structures like text and images. DataLoader doesn't need to be aware of the source of data as long as Dataset provides a way to get the number of records and to load a record by index. As an outcome, Dataset doesn't need to hold in memory all data at once. Needless to say, that one can implement its own versions of Dataset and DataLoader, but we are going to use existing implementation. + +Below we define 2 datasets: training dataset and validation dataset. It is a good practice to measure performance of a trained model on a data that the network hasn't seen before. That is why we are going to use training set for training the model and validation set to calculate model's accuracy. + + +```python +train_x, train_ground_truth_class = get_random_data(train_data_size, ctx) +train_dataset = ArrayDataset(train_x, train_ground_truth_class) +train_dataloader = DataLoader(train_dataset, batch_size=batch_size, shuffle=True) + +val_x, val_ground_truth_class = get_random_data(val_data_size, ctx) +val_dataset = ArrayDataset(val_x, val_ground_truth_class) +val_dataloader = DataLoader(val_dataset, batch_size=batch_size, shuffle=True) +``` + +## Defining and training the model + +In real application, model can be arbitrary complex. The only requirement for the logistic regression is that the last layer of the network must be a single neuron. Apache MXNet allows us to do so by using `Dense` layer and specifying the number of units to 1. + +Below, we define a model which has an input layer of 10 neurons, a couple inner layers of 10 neurons each, and output layer of 1 neuron, as it is required by logistic regression. We stack the layers using `HybridSequential` block and initialize parameters of the network using `Xavier` initialization. + + +```python +net = nn.HybridSequential() + +with net.name_scope(): +net.add(nn.Dense(units=10, activation='relu')) # input layer +net.add(nn.Dense(units=10, activation='relu')) # inner layer 1 +net.add(nn.Dense(units=10, activation='relu')) # inner layer 2 +net.add(nn.Dense(units=1)) # output layer: notice, it must have only 1 neuron + +net.initialize(mx.init.Xavier(magnitude=2.34)) +``` + +After defining the model, we need to define a few more thing: our loss, our trainer and our metric. + +Loss function is used to calculate how the output of the network different from the ground truth. In case of the logistic regression the ground truth are class labels, which can be either 0 or 1. Because of that, we are using `SigmoidBinaryCrossEntropyLoss`, which suites well for that scenario. + +Trainer object allows to specify the method of training to be used. There are various methods available, and for our tutorial we use a widely accepted method Stochastic Gradient Descent. We
[GitHub] safrooze commented on a change in pull request #11651: Add logistic regression tutorial
safrooze commented on a change in pull request #11651: Add logistic regression tutorial URL: https://github.com/apache/incubator-mxnet/pull/11651#discussion_r202895201 ## File path: docs/tutorials/gluon/logistic_regression_explained.md ## @@ -0,0 +1,215 @@ + +# Logistic regression using Gluon API explained + +Logistic Regression is one of the first models newcomers to Deep Learning are implementing. In this tutorial I am going to focus on how to do logistic regression using Gluon API and provide some high level tips. + +Before anything else, let's import required packages for this tutorial. + + +```python +import numpy as np +import mxnet as mx +from mxnet import nd, autograd, gluon +from mxnet.gluon import nn, Trainer +from mxnet.gluon.data import DataLoader, ArrayDataset + +mx.random.seed(12345) # Added for reproducibility +``` + +In this tutorial we will use fake dataset, which contains 10 features drawn from a normal distribution with mean equals to 0 and standard deviation equals to 1, and a class label, which can be either 0 or 1. The length of the dataset is an arbitrary value. The function below helps us to generate a dataset. + + +```python +def get_random_data(size, ctx): +x = nd.normal(0, 1, shape=(size, 10), ctx=ctx) +# Class label is generated via non-random logic so the network would have a pattern to look for +# Number 3 is selected to make sure that number of positive examples smaller than negative, but not too small +y = x.sum(axis=1) > 3 +return x, y +``` + +Also, let's define a set of hyperparameters, that we are going to use later. Since our model is simple and dataset is small, we are going to use CPU for calculations. Feel free to change it to GPU for a more advanced scenario. + + +```python +ctx = mx.cpu() +train_data_size = 1000 +val_data_size = 100 +batch_size = 10 +``` + +## Working with data + +To work with data, Apache MXNet provides Dataset and DataLoader classes. The former is used to provide an indexed access to the data, the latter is used to shuffle and batchify the data. + +This separation is done because a source of Dataset can vary from a simple array of numbers to complex data structures like text and images. DataLoader doesn't need to be aware of the source of data as long as Dataset provides a way to get the number of records and to load a record by index. As an outcome, Dataset doesn't need to hold in memory all data at once. Needless to say, that one can implement its own versions of Dataset and DataLoader, but we are going to use existing implementation. + +Below we define 2 datasets: training dataset and validation dataset. It is a good practice to measure performance of a trained model on a data that the network hasn't seen before. That is why we are going to use training set for training the model and validation set to calculate model's accuracy. + + +```python +train_x, train_ground_truth_class = get_random_data(train_data_size, ctx) +train_dataset = ArrayDataset(train_x, train_ground_truth_class) +train_dataloader = DataLoader(train_dataset, batch_size=batch_size, shuffle=True) + +val_x, val_ground_truth_class = get_random_data(val_data_size, ctx) +val_dataset = ArrayDataset(val_x, val_ground_truth_class) +val_dataloader = DataLoader(val_dataset, batch_size=batch_size, shuffle=True) +``` + +## Defining and training the model + +In real application, model can be arbitrary complex. The only requirement for the logistic regression is that the last layer of the network must be a single neuron. Apache MXNet allows us to do so by using `Dense` layer and specifying the number of units to 1. + +Below, we define a model which has an input layer of 10 neurons, a couple inner layers of 10 neurons each, and output layer of 1 neuron, as it is required by logistic regression. We stack the layers using `HybridSequential` block and initialize parameters of the network using `Xavier` initialization. + + +```python +net = nn.HybridSequential() + +with net.name_scope(): +net.add(nn.Dense(units=10, activation='relu')) # input layer +net.add(nn.Dense(units=10, activation='relu')) # inner layer 1 +net.add(nn.Dense(units=10, activation='relu')) # inner layer 2 +net.add(nn.Dense(units=1)) # output layer: notice, it must have only 1 neuron + +net.initialize(mx.init.Xavier(magnitude=2.34)) +``` + +After defining the model, we need to define a few more thing: our loss, our trainer and our metric. + +Loss function is used to calculate how the output of the network different from the ground truth. In case of the logistic regression the ground truth are class labels, which can be either 0 or 1. Because of that, we are using `SigmoidBinaryCrossEntropyLoss`, which suites well for that scenario. + +Trainer object allows to specify the method of training to be used. There are various methods available, and for our tutorial we use a widely accepted method Stochastic Gradient Descent. We
[GitHub] safrooze commented on a change in pull request #11651: Add logistic regression tutorial
safrooze commented on a change in pull request #11651: Add logistic regression tutorial URL: https://github.com/apache/incubator-mxnet/pull/11651#discussion_r202893568 ## File path: docs/tutorials/gluon/logistic_regression_explained.md ## @@ -0,0 +1,215 @@ + +# Logistic regression using Gluon API explained + +Logistic Regression is one of the first models newcomers to Deep Learning are implementing. In this tutorial I am going to focus on how to do logistic regression using Gluon API and provide some high level tips. + +Before anything else, let's import required packages for this tutorial. + + +```python +import numpy as np +import mxnet as mx +from mxnet import nd, autograd, gluon +from mxnet.gluon import nn, Trainer +from mxnet.gluon.data import DataLoader, ArrayDataset + +mx.random.seed(12345) # Added for reproducibility +``` + +In this tutorial we will use fake dataset, which contains 10 features drawn from a normal distribution with mean equals to 0 and standard deviation equals to 1, and a class label, which can be either 0 or 1. The length of the dataset is an arbitrary value. The function below helps us to generate a dataset. + + +```python +def get_random_data(size, ctx): +x = nd.normal(0, 1, shape=(size, 10), ctx=ctx) +# Class label is generated via non-random logic so the network would have a pattern to look for +# Number 3 is selected to make sure that number of positive examples smaller than negative, but not too small +y = x.sum(axis=1) > 3 +return x, y +``` + +Also, let's define a set of hyperparameters, that we are going to use later. Since our model is simple and dataset is small, we are going to use CPU for calculations. Feel free to change it to GPU for a more advanced scenario. + + +```python +ctx = mx.cpu() +train_data_size = 1000 +val_data_size = 100 +batch_size = 10 +``` + +## Working with data + +To work with data, Apache MXNet provides Dataset and DataLoader classes. The former is used to provide an indexed access to the data, the latter is used to shuffle and batchify the data. + +This separation is done because a source of Dataset can vary from a simple array of numbers to complex data structures like text and images. DataLoader doesn't need to be aware of the source of data as long as Dataset provides a way to get the number of records and to load a record by index. As an outcome, Dataset doesn't need to hold in memory all data at once. Needless to say, that one can implement its own versions of Dataset and DataLoader, but we are going to use existing implementation. + +Below we define 2 datasets: training dataset and validation dataset. It is a good practice to measure performance of a trained model on a data that the network hasn't seen before. That is why we are going to use training set for training the model and validation set to calculate model's accuracy. + + +```python +train_x, train_ground_truth_class = get_random_data(train_data_size, ctx) +train_dataset = ArrayDataset(train_x, train_ground_truth_class) +train_dataloader = DataLoader(train_dataset, batch_size=batch_size, shuffle=True) + +val_x, val_ground_truth_class = get_random_data(val_data_size, ctx) +val_dataset = ArrayDataset(val_x, val_ground_truth_class) +val_dataloader = DataLoader(val_dataset, batch_size=batch_size, shuffle=True) +``` + +## Defining and training the model + +In real application, model can be arbitrary complex. The only requirement for the logistic regression is that the last layer of the network must be a single neuron. Apache MXNet allows us to do so by using `Dense` layer and specifying the number of units to 1. Review comment: Make references to functions a link to the API. This is an automated message from the Apache Git Service. To respond to the message, please log on GitHub and use the URL above to go to the specific comment. For queries about this service, please contact Infrastructure at: us...@infra.apache.org With regards, Apache Git Services
[GitHub] safrooze commented on a change in pull request #11651: Add logistic regression tutorial
safrooze commented on a change in pull request #11651: Add logistic regression tutorial URL: https://github.com/apache/incubator-mxnet/pull/11651#discussion_r202897660 ## File path: docs/tutorials/gluon/logistic_regression_explained.md ## @@ -0,0 +1,215 @@ + +# Logistic regression using Gluon API explained + +Logistic Regression is one of the first models newcomers to Deep Learning are implementing. In this tutorial I am going to focus on how to do logistic regression using Gluon API and provide some high level tips. + +Before anything else, let's import required packages for this tutorial. + + +```python +import numpy as np +import mxnet as mx +from mxnet import nd, autograd, gluon +from mxnet.gluon import nn, Trainer +from mxnet.gluon.data import DataLoader, ArrayDataset + +mx.random.seed(12345) # Added for reproducibility +``` + +In this tutorial we will use fake dataset, which contains 10 features drawn from a normal distribution with mean equals to 0 and standard deviation equals to 1, and a class label, which can be either 0 or 1. The length of the dataset is an arbitrary value. The function below helps us to generate a dataset. + + +```python +def get_random_data(size, ctx): +x = nd.normal(0, 1, shape=(size, 10), ctx=ctx) +# Class label is generated via non-random logic so the network would have a pattern to look for +# Number 3 is selected to make sure that number of positive examples smaller than negative, but not too small +y = x.sum(axis=1) > 3 +return x, y +``` + +Also, let's define a set of hyperparameters, that we are going to use later. Since our model is simple and dataset is small, we are going to use CPU for calculations. Feel free to change it to GPU for a more advanced scenario. + + +```python +ctx = mx.cpu() +train_data_size = 1000 +val_data_size = 100 +batch_size = 10 +``` + +## Working with data + +To work with data, Apache MXNet provides Dataset and DataLoader classes. The former is used to provide an indexed access to the data, the latter is used to shuffle and batchify the data. + +This separation is done because a source of Dataset can vary from a simple array of numbers to complex data structures like text and images. DataLoader doesn't need to be aware of the source of data as long as Dataset provides a way to get the number of records and to load a record by index. As an outcome, Dataset doesn't need to hold in memory all data at once. Needless to say, that one can implement its own versions of Dataset and DataLoader, but we are going to use existing implementation. + +Below we define 2 datasets: training dataset and validation dataset. It is a good practice to measure performance of a trained model on a data that the network hasn't seen before. That is why we are going to use training set for training the model and validation set to calculate model's accuracy. + + +```python +train_x, train_ground_truth_class = get_random_data(train_data_size, ctx) +train_dataset = ArrayDataset(train_x, train_ground_truth_class) +train_dataloader = DataLoader(train_dataset, batch_size=batch_size, shuffle=True) + +val_x, val_ground_truth_class = get_random_data(val_data_size, ctx) +val_dataset = ArrayDataset(val_x, val_ground_truth_class) +val_dataloader = DataLoader(val_dataset, batch_size=batch_size, shuffle=True) +``` + +## Defining and training the model + +In real application, model can be arbitrary complex. The only requirement for the logistic regression is that the last layer of the network must be a single neuron. Apache MXNet allows us to do so by using `Dense` layer and specifying the number of units to 1. + +Below, we define a model which has an input layer of 10 neurons, a couple inner layers of 10 neurons each, and output layer of 1 neuron, as it is required by logistic regression. We stack the layers using `HybridSequential` block and initialize parameters of the network using `Xavier` initialization. + + +```python +net = nn.HybridSequential() + +with net.name_scope(): +net.add(nn.Dense(units=10, activation='relu')) # input layer +net.add(nn.Dense(units=10, activation='relu')) # inner layer 1 +net.add(nn.Dense(units=10, activation='relu')) # inner layer 2 +net.add(nn.Dense(units=1)) # output layer: notice, it must have only 1 neuron + +net.initialize(mx.init.Xavier(magnitude=2.34)) +``` + +After defining the model, we need to define a few more thing: our loss, our trainer and our metric. + +Loss function is used to calculate how the output of the network different from the ground truth. In case of the logistic regression the ground truth are class labels, which can be either 0 or 1. Because of that, we are using `SigmoidBinaryCrossEntropyLoss`, which suites well for that scenario. + +Trainer object allows to specify the method of training to be used. There are various methods available, and for our tutorial we use a widely accepted method Stochastic Gradient Descent. We
[GitHub] safrooze commented on a change in pull request #11651: Add logistic regression tutorial
safrooze commented on a change in pull request #11651: Add logistic regression tutorial URL: https://github.com/apache/incubator-mxnet/pull/11651#discussion_r202896797 ## File path: docs/tutorials/gluon/logistic_regression_explained.md ## @@ -0,0 +1,215 @@ + +# Logistic regression using Gluon API explained + +Logistic Regression is one of the first models newcomers to Deep Learning are implementing. In this tutorial I am going to focus on how to do logistic regression using Gluon API and provide some high level tips. + +Before anything else, let's import required packages for this tutorial. + + +```python +import numpy as np +import mxnet as mx +from mxnet import nd, autograd, gluon +from mxnet.gluon import nn, Trainer +from mxnet.gluon.data import DataLoader, ArrayDataset + +mx.random.seed(12345) # Added for reproducibility +``` + +In this tutorial we will use fake dataset, which contains 10 features drawn from a normal distribution with mean equals to 0 and standard deviation equals to 1, and a class label, which can be either 0 or 1. The length of the dataset is an arbitrary value. The function below helps us to generate a dataset. + + +```python +def get_random_data(size, ctx): +x = nd.normal(0, 1, shape=(size, 10), ctx=ctx) +# Class label is generated via non-random logic so the network would have a pattern to look for +# Number 3 is selected to make sure that number of positive examples smaller than negative, but not too small +y = x.sum(axis=1) > 3 +return x, y +``` + +Also, let's define a set of hyperparameters, that we are going to use later. Since our model is simple and dataset is small, we are going to use CPU for calculations. Feel free to change it to GPU for a more advanced scenario. + + +```python +ctx = mx.cpu() +train_data_size = 1000 +val_data_size = 100 +batch_size = 10 +``` + +## Working with data + +To work with data, Apache MXNet provides Dataset and DataLoader classes. The former is used to provide an indexed access to the data, the latter is used to shuffle and batchify the data. + +This separation is done because a source of Dataset can vary from a simple array of numbers to complex data structures like text and images. DataLoader doesn't need to be aware of the source of data as long as Dataset provides a way to get the number of records and to load a record by index. As an outcome, Dataset doesn't need to hold in memory all data at once. Needless to say, that one can implement its own versions of Dataset and DataLoader, but we are going to use existing implementation. + +Below we define 2 datasets: training dataset and validation dataset. It is a good practice to measure performance of a trained model on a data that the network hasn't seen before. That is why we are going to use training set for training the model and validation set to calculate model's accuracy. + + +```python +train_x, train_ground_truth_class = get_random_data(train_data_size, ctx) +train_dataset = ArrayDataset(train_x, train_ground_truth_class) +train_dataloader = DataLoader(train_dataset, batch_size=batch_size, shuffle=True) + +val_x, val_ground_truth_class = get_random_data(val_data_size, ctx) +val_dataset = ArrayDataset(val_x, val_ground_truth_class) +val_dataloader = DataLoader(val_dataset, batch_size=batch_size, shuffle=True) +``` + +## Defining and training the model + +In real application, model can be arbitrary complex. The only requirement for the logistic regression is that the last layer of the network must be a single neuron. Apache MXNet allows us to do so by using `Dense` layer and specifying the number of units to 1. + +Below, we define a model which has an input layer of 10 neurons, a couple inner layers of 10 neurons each, and output layer of 1 neuron, as it is required by logistic regression. We stack the layers using `HybridSequential` block and initialize parameters of the network using `Xavier` initialization. + + +```python +net = nn.HybridSequential() + +with net.name_scope(): +net.add(nn.Dense(units=10, activation='relu')) # input layer +net.add(nn.Dense(units=10, activation='relu')) # inner layer 1 +net.add(nn.Dense(units=10, activation='relu')) # inner layer 2 +net.add(nn.Dense(units=1)) # output layer: notice, it must have only 1 neuron + +net.initialize(mx.init.Xavier(magnitude=2.34)) +``` + +After defining the model, we need to define a few more thing: our loss, our trainer and our metric. + +Loss function is used to calculate how the output of the network different from the ground truth. In case of the logistic regression the ground truth are class labels, which can be either 0 or 1. Because of that, we are using `SigmoidBinaryCrossEntropyLoss`, which suites well for that scenario. + +Trainer object allows to specify the method of training to be used. There are various methods available, and for our tutorial we use a widely accepted method Stochastic Gradient Descent. We
[GitHub] safrooze commented on a change in pull request #11651: Add logistic regression tutorial
safrooze commented on a change in pull request #11651: Add logistic regression tutorial URL: https://github.com/apache/incubator-mxnet/pull/11651#discussion_r202896471 ## File path: docs/tutorials/gluon/logistic_regression_explained.md ## @@ -0,0 +1,215 @@ + +# Logistic regression using Gluon API explained + +Logistic Regression is one of the first models newcomers to Deep Learning are implementing. In this tutorial I am going to focus on how to do logistic regression using Gluon API and provide some high level tips. + +Before anything else, let's import required packages for this tutorial. + + +```python +import numpy as np +import mxnet as mx +from mxnet import nd, autograd, gluon +from mxnet.gluon import nn, Trainer +from mxnet.gluon.data import DataLoader, ArrayDataset + +mx.random.seed(12345) # Added for reproducibility +``` + +In this tutorial we will use fake dataset, which contains 10 features drawn from a normal distribution with mean equals to 0 and standard deviation equals to 1, and a class label, which can be either 0 or 1. The length of the dataset is an arbitrary value. The function below helps us to generate a dataset. + + +```python +def get_random_data(size, ctx): +x = nd.normal(0, 1, shape=(size, 10), ctx=ctx) +# Class label is generated via non-random logic so the network would have a pattern to look for +# Number 3 is selected to make sure that number of positive examples smaller than negative, but not too small +y = x.sum(axis=1) > 3 +return x, y +``` + +Also, let's define a set of hyperparameters, that we are going to use later. Since our model is simple and dataset is small, we are going to use CPU for calculations. Feel free to change it to GPU for a more advanced scenario. + + +```python +ctx = mx.cpu() +train_data_size = 1000 +val_data_size = 100 +batch_size = 10 +``` + +## Working with data + +To work with data, Apache MXNet provides Dataset and DataLoader classes. The former is used to provide an indexed access to the data, the latter is used to shuffle and batchify the data. + +This separation is done because a source of Dataset can vary from a simple array of numbers to complex data structures like text and images. DataLoader doesn't need to be aware of the source of data as long as Dataset provides a way to get the number of records and to load a record by index. As an outcome, Dataset doesn't need to hold in memory all data at once. Needless to say, that one can implement its own versions of Dataset and DataLoader, but we are going to use existing implementation. + +Below we define 2 datasets: training dataset and validation dataset. It is a good practice to measure performance of a trained model on a data that the network hasn't seen before. That is why we are going to use training set for training the model and validation set to calculate model's accuracy. + + +```python +train_x, train_ground_truth_class = get_random_data(train_data_size, ctx) +train_dataset = ArrayDataset(train_x, train_ground_truth_class) +train_dataloader = DataLoader(train_dataset, batch_size=batch_size, shuffle=True) + +val_x, val_ground_truth_class = get_random_data(val_data_size, ctx) +val_dataset = ArrayDataset(val_x, val_ground_truth_class) +val_dataloader = DataLoader(val_dataset, batch_size=batch_size, shuffle=True) +``` + +## Defining and training the model + +In real application, model can be arbitrary complex. The only requirement for the logistic regression is that the last layer of the network must be a single neuron. Apache MXNet allows us to do so by using `Dense` layer and specifying the number of units to 1. + +Below, we define a model which has an input layer of 10 neurons, a couple inner layers of 10 neurons each, and output layer of 1 neuron, as it is required by logistic regression. We stack the layers using `HybridSequential` block and initialize parameters of the network using `Xavier` initialization. + + +```python +net = nn.HybridSequential() + +with net.name_scope(): +net.add(nn.Dense(units=10, activation='relu')) # input layer +net.add(nn.Dense(units=10, activation='relu')) # inner layer 1 +net.add(nn.Dense(units=10, activation='relu')) # inner layer 2 +net.add(nn.Dense(units=1)) # output layer: notice, it must have only 1 neuron + +net.initialize(mx.init.Xavier(magnitude=2.34)) +``` + +After defining the model, we need to define a few more thing: our loss, our trainer and our metric. + +Loss function is used to calculate how the output of the network different from the ground truth. In case of the logistic regression the ground truth are class labels, which can be either 0 or 1. Because of that, we are using `SigmoidBinaryCrossEntropyLoss`, which suites well for that scenario. + +Trainer object allows to specify the method of training to be used. There are various methods available, and for our tutorial we use a widely accepted method Stochastic Gradient Descent. We
[GitHub] safrooze commented on a change in pull request #11651: Add logistic regression tutorial
safrooze commented on a change in pull request #11651: Add logistic regression tutorial URL: https://github.com/apache/incubator-mxnet/pull/11651#discussion_r202897112 ## File path: docs/tutorials/gluon/logistic_regression_explained.md ## @@ -0,0 +1,215 @@ + +# Logistic regression using Gluon API explained + +Logistic Regression is one of the first models newcomers to Deep Learning are implementing. In this tutorial I am going to focus on how to do logistic regression using Gluon API and provide some high level tips. + +Before anything else, let's import required packages for this tutorial. + + +```python +import numpy as np +import mxnet as mx +from mxnet import nd, autograd, gluon +from mxnet.gluon import nn, Trainer +from mxnet.gluon.data import DataLoader, ArrayDataset + +mx.random.seed(12345) # Added for reproducibility +``` + +In this tutorial we will use fake dataset, which contains 10 features drawn from a normal distribution with mean equals to 0 and standard deviation equals to 1, and a class label, which can be either 0 or 1. The length of the dataset is an arbitrary value. The function below helps us to generate a dataset. + + +```python +def get_random_data(size, ctx): +x = nd.normal(0, 1, shape=(size, 10), ctx=ctx) +# Class label is generated via non-random logic so the network would have a pattern to look for +# Number 3 is selected to make sure that number of positive examples smaller than negative, but not too small +y = x.sum(axis=1) > 3 +return x, y +``` + +Also, let's define a set of hyperparameters, that we are going to use later. Since our model is simple and dataset is small, we are going to use CPU for calculations. Feel free to change it to GPU for a more advanced scenario. + + +```python +ctx = mx.cpu() +train_data_size = 1000 +val_data_size = 100 +batch_size = 10 +``` + +## Working with data + +To work with data, Apache MXNet provides Dataset and DataLoader classes. The former is used to provide an indexed access to the data, the latter is used to shuffle and batchify the data. + +This separation is done because a source of Dataset can vary from a simple array of numbers to complex data structures like text and images. DataLoader doesn't need to be aware of the source of data as long as Dataset provides a way to get the number of records and to load a record by index. As an outcome, Dataset doesn't need to hold in memory all data at once. Needless to say, that one can implement its own versions of Dataset and DataLoader, but we are going to use existing implementation. + +Below we define 2 datasets: training dataset and validation dataset. It is a good practice to measure performance of a trained model on a data that the network hasn't seen before. That is why we are going to use training set for training the model and validation set to calculate model's accuracy. + + +```python +train_x, train_ground_truth_class = get_random_data(train_data_size, ctx) +train_dataset = ArrayDataset(train_x, train_ground_truth_class) +train_dataloader = DataLoader(train_dataset, batch_size=batch_size, shuffle=True) + +val_x, val_ground_truth_class = get_random_data(val_data_size, ctx) +val_dataset = ArrayDataset(val_x, val_ground_truth_class) +val_dataloader = DataLoader(val_dataset, batch_size=batch_size, shuffle=True) +``` + +## Defining and training the model + +In real application, model can be arbitrary complex. The only requirement for the logistic regression is that the last layer of the network must be a single neuron. Apache MXNet allows us to do so by using `Dense` layer and specifying the number of units to 1. + +Below, we define a model which has an input layer of 10 neurons, a couple inner layers of 10 neurons each, and output layer of 1 neuron, as it is required by logistic regression. We stack the layers using `HybridSequential` block and initialize parameters of the network using `Xavier` initialization. + + +```python +net = nn.HybridSequential() + +with net.name_scope(): +net.add(nn.Dense(units=10, activation='relu')) # input layer +net.add(nn.Dense(units=10, activation='relu')) # inner layer 1 +net.add(nn.Dense(units=10, activation='relu')) # inner layer 2 +net.add(nn.Dense(units=1)) # output layer: notice, it must have only 1 neuron + +net.initialize(mx.init.Xavier(magnitude=2.34)) +``` + +After defining the model, we need to define a few more thing: our loss, our trainer and our metric. + +Loss function is used to calculate how the output of the network different from the ground truth. In case of the logistic regression the ground truth are class labels, which can be either 0 or 1. Because of that, we are using `SigmoidBinaryCrossEntropyLoss`, which suites well for that scenario. + +Trainer object allows to specify the method of training to be used. There are various methods available, and for our tutorial we use a widely accepted method Stochastic Gradient Descent. We
[GitHub] safrooze commented on a change in pull request #11651: Add logistic regression tutorial
safrooze commented on a change in pull request #11651: Add logistic regression tutorial URL: https://github.com/apache/incubator-mxnet/pull/11651#discussion_r202897762 ## File path: docs/tutorials/gluon/logistic_regression_explained.md ## @@ -0,0 +1,215 @@ + +# Logistic regression using Gluon API explained + +Logistic Regression is one of the first models newcomers to Deep Learning are implementing. In this tutorial I am going to focus on how to do logistic regression using Gluon API and provide some high level tips. + +Before anything else, let's import required packages for this tutorial. + + +```python +import numpy as np +import mxnet as mx +from mxnet import nd, autograd, gluon +from mxnet.gluon import nn, Trainer +from mxnet.gluon.data import DataLoader, ArrayDataset + +mx.random.seed(12345) # Added for reproducibility +``` + +In this tutorial we will use fake dataset, which contains 10 features drawn from a normal distribution with mean equals to 0 and standard deviation equals to 1, and a class label, which can be either 0 or 1. The length of the dataset is an arbitrary value. The function below helps us to generate a dataset. + + +```python +def get_random_data(size, ctx): +x = nd.normal(0, 1, shape=(size, 10), ctx=ctx) +# Class label is generated via non-random logic so the network would have a pattern to look for +# Number 3 is selected to make sure that number of positive examples smaller than negative, but not too small +y = x.sum(axis=1) > 3 +return x, y +``` + +Also, let's define a set of hyperparameters, that we are going to use later. Since our model is simple and dataset is small, we are going to use CPU for calculations. Feel free to change it to GPU for a more advanced scenario. + + +```python +ctx = mx.cpu() +train_data_size = 1000 +val_data_size = 100 +batch_size = 10 +``` + +## Working with data + +To work with data, Apache MXNet provides Dataset and DataLoader classes. The former is used to provide an indexed access to the data, the latter is used to shuffle and batchify the data. + +This separation is done because a source of Dataset can vary from a simple array of numbers to complex data structures like text and images. DataLoader doesn't need to be aware of the source of data as long as Dataset provides a way to get the number of records and to load a record by index. As an outcome, Dataset doesn't need to hold in memory all data at once. Needless to say, that one can implement its own versions of Dataset and DataLoader, but we are going to use existing implementation. + +Below we define 2 datasets: training dataset and validation dataset. It is a good practice to measure performance of a trained model on a data that the network hasn't seen before. That is why we are going to use training set for training the model and validation set to calculate model's accuracy. + + +```python +train_x, train_ground_truth_class = get_random_data(train_data_size, ctx) +train_dataset = ArrayDataset(train_x, train_ground_truth_class) +train_dataloader = DataLoader(train_dataset, batch_size=batch_size, shuffle=True) + +val_x, val_ground_truth_class = get_random_data(val_data_size, ctx) +val_dataset = ArrayDataset(val_x, val_ground_truth_class) +val_dataloader = DataLoader(val_dataset, batch_size=batch_size, shuffle=True) +``` + +## Defining and training the model + +In real application, model can be arbitrary complex. The only requirement for the logistic regression is that the last layer of the network must be a single neuron. Apache MXNet allows us to do so by using `Dense` layer and specifying the number of units to 1. + +Below, we define a model which has an input layer of 10 neurons, a couple inner layers of 10 neurons each, and output layer of 1 neuron, as it is required by logistic regression. We stack the layers using `HybridSequential` block and initialize parameters of the network using `Xavier` initialization. + + +```python +net = nn.HybridSequential() + +with net.name_scope(): +net.add(nn.Dense(units=10, activation='relu')) # input layer +net.add(nn.Dense(units=10, activation='relu')) # inner layer 1 +net.add(nn.Dense(units=10, activation='relu')) # inner layer 2 +net.add(nn.Dense(units=1)) # output layer: notice, it must have only 1 neuron + +net.initialize(mx.init.Xavier(magnitude=2.34)) +``` + +After defining the model, we need to define a few more thing: our loss, our trainer and our metric. + +Loss function is used to calculate how the output of the network different from the ground truth. In case of the logistic regression the ground truth are class labels, which can be either 0 or 1. Because of that, we are using `SigmoidBinaryCrossEntropyLoss`, which suites well for that scenario. + +Trainer object allows to specify the method of training to be used. There are various methods available, and for our tutorial we use a widely accepted method Stochastic Gradient Descent. We
[GitHub] safrooze commented on a change in pull request #11651: Add logistic regression tutorial
safrooze commented on a change in pull request #11651: Add logistic regression tutorial URL: https://github.com/apache/incubator-mxnet/pull/11651#discussion_r202893857 ## File path: docs/tutorials/gluon/logistic_regression_explained.md ## @@ -0,0 +1,215 @@ + +# Logistic regression using Gluon API explained + +Logistic Regression is one of the first models newcomers to Deep Learning are implementing. In this tutorial I am going to focus on how to do logistic regression using Gluon API and provide some high level tips. + +Before anything else, let's import required packages for this tutorial. + + +```python +import numpy as np +import mxnet as mx +from mxnet import nd, autograd, gluon +from mxnet.gluon import nn, Trainer +from mxnet.gluon.data import DataLoader, ArrayDataset + +mx.random.seed(12345) # Added for reproducibility +``` + +In this tutorial we will use fake dataset, which contains 10 features drawn from a normal distribution with mean equals to 0 and standard deviation equals to 1, and a class label, which can be either 0 or 1. The length of the dataset is an arbitrary value. The function below helps us to generate a dataset. + + +```python +def get_random_data(size, ctx): +x = nd.normal(0, 1, shape=(size, 10), ctx=ctx) +# Class label is generated via non-random logic so the network would have a pattern to look for +# Number 3 is selected to make sure that number of positive examples smaller than negative, but not too small +y = x.sum(axis=1) > 3 +return x, y +``` + +Also, let's define a set of hyperparameters, that we are going to use later. Since our model is simple and dataset is small, we are going to use CPU for calculations. Feel free to change it to GPU for a more advanced scenario. + + +```python +ctx = mx.cpu() +train_data_size = 1000 +val_data_size = 100 +batch_size = 10 +``` + +## Working with data + +To work with data, Apache MXNet provides Dataset and DataLoader classes. The former is used to provide an indexed access to the data, the latter is used to shuffle and batchify the data. + +This separation is done because a source of Dataset can vary from a simple array of numbers to complex data structures like text and images. DataLoader doesn't need to be aware of the source of data as long as Dataset provides a way to get the number of records and to load a record by index. As an outcome, Dataset doesn't need to hold in memory all data at once. Needless to say, that one can implement its own versions of Dataset and DataLoader, but we are going to use existing implementation. + +Below we define 2 datasets: training dataset and validation dataset. It is a good practice to measure performance of a trained model on a data that the network hasn't seen before. That is why we are going to use training set for training the model and validation set to calculate model's accuracy. + + +```python +train_x, train_ground_truth_class = get_random_data(train_data_size, ctx) +train_dataset = ArrayDataset(train_x, train_ground_truth_class) +train_dataloader = DataLoader(train_dataset, batch_size=batch_size, shuffle=True) + +val_x, val_ground_truth_class = get_random_data(val_data_size, ctx) +val_dataset = ArrayDataset(val_x, val_ground_truth_class) +val_dataloader = DataLoader(val_dataset, batch_size=batch_size, shuffle=True) +``` + +## Defining and training the model + +In real application, model can be arbitrary complex. The only requirement for the logistic regression is that the last layer of the network must be a single neuron. Apache MXNet allows us to do so by using `Dense` layer and specifying the number of units to 1. + +Below, we define a model which has an input layer of 10 neurons, a couple inner layers of 10 neurons each, and output layer of 1 neuron, as it is required by logistic regression. We stack the layers using `HybridSequential` block and initialize parameters of the network using `Xavier` initialization. + + +```python +net = nn.HybridSequential() + +with net.name_scope(): +net.add(nn.Dense(units=10, activation='relu')) # input layer +net.add(nn.Dense(units=10, activation='relu')) # inner layer 1 +net.add(nn.Dense(units=10, activation='relu')) # inner layer 2 +net.add(nn.Dense(units=1)) # output layer: notice, it must have only 1 neuron + +net.initialize(mx.init.Xavier(magnitude=2.34)) +``` + +After defining the model, we need to define a few more thing: our loss, our trainer and our metric. Review comment: ***"a few more things"***. This is an automated message from the Apache Git Service. To respond to the message, please log on GitHub and use the URL above to go to the specific comment. For queries about this service, please contact Infrastructure at: us...@infra.apache.org With regards, Apache Git Services
[GitHub] safrooze commented on a change in pull request #11651: Add logistic regression tutorial
safrooze commented on a change in pull request #11651: Add logistic regression tutorial URL: https://github.com/apache/incubator-mxnet/pull/11651#discussion_r202892204 ## File path: docs/tutorials/gluon/logistic_regression_explained.md ## @@ -0,0 +1,215 @@ + +# Logistic regression using Gluon API explained Review comment: This tutorial is very similar to [this Straight Dope tutorial](https://gluon.mxnet.io/chapter02_supervised-learning/logistic-regression-gluon.html). Did you consider focusing purely on the confusion around `Accuracy` and pointing user to Straight Dope for further reading? This is an automated message from the Apache Git Service. To respond to the message, please log on GitHub and use the URL above to go to the specific comment. For queries about this service, please contact Infrastructure at: us...@infra.apache.org With regards, Apache Git Services
[GitHub] safrooze commented on a change in pull request #11651: Add logistic regression tutorial
safrooze commented on a change in pull request #11651: Add logistic regression tutorial URL: https://github.com/apache/incubator-mxnet/pull/11651#discussion_r202892495 ## File path: docs/tutorials/gluon/logistic_regression_explained.md ## @@ -0,0 +1,215 @@ + +# Logistic regression using Gluon API explained + +Logistic Regression is one of the first models newcomers to Deep Learning are implementing. In this tutorial I am going to focus on how to do logistic regression using Gluon API and provide some high level tips. + +Before anything else, let's import required packages for this tutorial. + + +```python +import numpy as np +import mxnet as mx +from mxnet import nd, autograd, gluon +from mxnet.gluon import nn, Trainer +from mxnet.gluon.data import DataLoader, ArrayDataset + +mx.random.seed(12345) # Added for reproducibility +``` + +In this tutorial we will use fake dataset, which contains 10 features drawn from a normal distribution with mean equals to 0 and standard deviation equals to 1, and a class label, which can be either 0 or 1. The length of the dataset is an arbitrary value. The function below helps us to generate a dataset. Review comment: The logistic regression tutorial in straight dope uses the Adult dataset. Did you consider using similar dataset (or perhaps simply pointing the user to that tutorial)? This is an automated message from the Apache Git Service. To respond to the message, please log on GitHub and use the URL above to go to the specific comment. For queries about this service, please contact Infrastructure at: us...@infra.apache.org With regards, Apache Git Services
[GitHub] safrooze commented on a change in pull request #11651: Add logistic regression tutorial
safrooze commented on a change in pull request #11651: Add logistic regression tutorial URL: https://github.com/apache/incubator-mxnet/pull/11651#discussion_r202895819 ## File path: docs/tutorials/gluon/logistic_regression_explained.md ## @@ -0,0 +1,215 @@ + +# Logistic regression using Gluon API explained + +Logistic Regression is one of the first models newcomers to Deep Learning are implementing. In this tutorial I am going to focus on how to do logistic regression using Gluon API and provide some high level tips. + +Before anything else, let's import required packages for this tutorial. + + +```python +import numpy as np +import mxnet as mx +from mxnet import nd, autograd, gluon +from mxnet.gluon import nn, Trainer +from mxnet.gluon.data import DataLoader, ArrayDataset + +mx.random.seed(12345) # Added for reproducibility +``` + +In this tutorial we will use fake dataset, which contains 10 features drawn from a normal distribution with mean equals to 0 and standard deviation equals to 1, and a class label, which can be either 0 or 1. The length of the dataset is an arbitrary value. The function below helps us to generate a dataset. + + +```python +def get_random_data(size, ctx): +x = nd.normal(0, 1, shape=(size, 10), ctx=ctx) +# Class label is generated via non-random logic so the network would have a pattern to look for +# Number 3 is selected to make sure that number of positive examples smaller than negative, but not too small +y = x.sum(axis=1) > 3 +return x, y +``` + +Also, let's define a set of hyperparameters, that we are going to use later. Since our model is simple and dataset is small, we are going to use CPU for calculations. Feel free to change it to GPU for a more advanced scenario. + + +```python +ctx = mx.cpu() +train_data_size = 1000 +val_data_size = 100 +batch_size = 10 +``` + +## Working with data + +To work with data, Apache MXNet provides Dataset and DataLoader classes. The former is used to provide an indexed access to the data, the latter is used to shuffle and batchify the data. + +This separation is done because a source of Dataset can vary from a simple array of numbers to complex data structures like text and images. DataLoader doesn't need to be aware of the source of data as long as Dataset provides a way to get the number of records and to load a record by index. As an outcome, Dataset doesn't need to hold in memory all data at once. Needless to say, that one can implement its own versions of Dataset and DataLoader, but we are going to use existing implementation. + +Below we define 2 datasets: training dataset and validation dataset. It is a good practice to measure performance of a trained model on a data that the network hasn't seen before. That is why we are going to use training set for training the model and validation set to calculate model's accuracy. + + +```python +train_x, train_ground_truth_class = get_random_data(train_data_size, ctx) +train_dataset = ArrayDataset(train_x, train_ground_truth_class) +train_dataloader = DataLoader(train_dataset, batch_size=batch_size, shuffle=True) + +val_x, val_ground_truth_class = get_random_data(val_data_size, ctx) +val_dataset = ArrayDataset(val_x, val_ground_truth_class) +val_dataloader = DataLoader(val_dataset, batch_size=batch_size, shuffle=True) +``` + +## Defining and training the model + +In real application, model can be arbitrary complex. The only requirement for the logistic regression is that the last layer of the network must be a single neuron. Apache MXNet allows us to do so by using `Dense` layer and specifying the number of units to 1. + +Below, we define a model which has an input layer of 10 neurons, a couple inner layers of 10 neurons each, and output layer of 1 neuron, as it is required by logistic regression. We stack the layers using `HybridSequential` block and initialize parameters of the network using `Xavier` initialization. + + +```python +net = nn.HybridSequential() + +with net.name_scope(): +net.add(nn.Dense(units=10, activation='relu')) # input layer +net.add(nn.Dense(units=10, activation='relu')) # inner layer 1 +net.add(nn.Dense(units=10, activation='relu')) # inner layer 2 +net.add(nn.Dense(units=1)) # output layer: notice, it must have only 1 neuron + +net.initialize(mx.init.Xavier(magnitude=2.34)) +``` + +After defining the model, we need to define a few more thing: our loss, our trainer and our metric. + +Loss function is used to calculate how the output of the network different from the ground truth. In case of the logistic regression the ground truth are class labels, which can be either 0 or 1. Because of that, we are using `SigmoidBinaryCrossEntropyLoss`, which suites well for that scenario. + +Trainer object allows to specify the method of training to be used. There are various methods available, and for our tutorial we use a widely accepted method Stochastic Gradient Descent. We
[GitHub] safrooze commented on a change in pull request #11651: Add logistic regression tutorial
safrooze commented on a change in pull request #11651: Add logistic regression tutorial URL: https://github.com/apache/incubator-mxnet/pull/11651#discussion_r202897546 ## File path: docs/tutorials/gluon/logistic_regression_explained.md ## @@ -0,0 +1,215 @@ + +# Logistic regression using Gluon API explained + +Logistic Regression is one of the first models newcomers to Deep Learning are implementing. In this tutorial I am going to focus on how to do logistic regression using Gluon API and provide some high level tips. + +Before anything else, let's import required packages for this tutorial. + + +```python +import numpy as np +import mxnet as mx +from mxnet import nd, autograd, gluon +from mxnet.gluon import nn, Trainer +from mxnet.gluon.data import DataLoader, ArrayDataset + +mx.random.seed(12345) # Added for reproducibility +``` + +In this tutorial we will use fake dataset, which contains 10 features drawn from a normal distribution with mean equals to 0 and standard deviation equals to 1, and a class label, which can be either 0 or 1. The length of the dataset is an arbitrary value. The function below helps us to generate a dataset. + + +```python +def get_random_data(size, ctx): +x = nd.normal(0, 1, shape=(size, 10), ctx=ctx) +# Class label is generated via non-random logic so the network would have a pattern to look for +# Number 3 is selected to make sure that number of positive examples smaller than negative, but not too small +y = x.sum(axis=1) > 3 +return x, y +``` + +Also, let's define a set of hyperparameters, that we are going to use later. Since our model is simple and dataset is small, we are going to use CPU for calculations. Feel free to change it to GPU for a more advanced scenario. + + +```python +ctx = mx.cpu() +train_data_size = 1000 +val_data_size = 100 +batch_size = 10 +``` + +## Working with data + +To work with data, Apache MXNet provides Dataset and DataLoader classes. The former is used to provide an indexed access to the data, the latter is used to shuffle and batchify the data. + +This separation is done because a source of Dataset can vary from a simple array of numbers to complex data structures like text and images. DataLoader doesn't need to be aware of the source of data as long as Dataset provides a way to get the number of records and to load a record by index. As an outcome, Dataset doesn't need to hold in memory all data at once. Needless to say, that one can implement its own versions of Dataset and DataLoader, but we are going to use existing implementation. + +Below we define 2 datasets: training dataset and validation dataset. It is a good practice to measure performance of a trained model on a data that the network hasn't seen before. That is why we are going to use training set for training the model and validation set to calculate model's accuracy. + + +```python +train_x, train_ground_truth_class = get_random_data(train_data_size, ctx) +train_dataset = ArrayDataset(train_x, train_ground_truth_class) +train_dataloader = DataLoader(train_dataset, batch_size=batch_size, shuffle=True) + +val_x, val_ground_truth_class = get_random_data(val_data_size, ctx) +val_dataset = ArrayDataset(val_x, val_ground_truth_class) +val_dataloader = DataLoader(val_dataset, batch_size=batch_size, shuffle=True) +``` + +## Defining and training the model + +In real application, model can be arbitrary complex. The only requirement for the logistic regression is that the last layer of the network must be a single neuron. Apache MXNet allows us to do so by using `Dense` layer and specifying the number of units to 1. + +Below, we define a model which has an input layer of 10 neurons, a couple inner layers of 10 neurons each, and output layer of 1 neuron, as it is required by logistic regression. We stack the layers using `HybridSequential` block and initialize parameters of the network using `Xavier` initialization. + + +```python +net = nn.HybridSequential() + +with net.name_scope(): +net.add(nn.Dense(units=10, activation='relu')) # input layer +net.add(nn.Dense(units=10, activation='relu')) # inner layer 1 +net.add(nn.Dense(units=10, activation='relu')) # inner layer 2 +net.add(nn.Dense(units=1)) # output layer: notice, it must have only 1 neuron + +net.initialize(mx.init.Xavier(magnitude=2.34)) +``` + +After defining the model, we need to define a few more thing: our loss, our trainer and our metric. + +Loss function is used to calculate how the output of the network different from the ground truth. In case of the logistic regression the ground truth are class labels, which can be either 0 or 1. Because of that, we are using `SigmoidBinaryCrossEntropyLoss`, which suites well for that scenario. + +Trainer object allows to specify the method of training to be used. There are various methods available, and for our tutorial we use a widely accepted method Stochastic Gradient Descent. We
[GitHub] safrooze commented on a change in pull request #11651: Add logistic regression tutorial
safrooze commented on a change in pull request #11651: Add logistic regression tutorial URL: https://github.com/apache/incubator-mxnet/pull/11651#discussion_r202895356 ## File path: docs/tutorials/gluon/logistic_regression_explained.md ## @@ -0,0 +1,215 @@ + +# Logistic regression using Gluon API explained + +Logistic Regression is one of the first models newcomers to Deep Learning are implementing. In this tutorial I am going to focus on how to do logistic regression using Gluon API and provide some high level tips. + +Before anything else, let's import required packages for this tutorial. + + +```python +import numpy as np +import mxnet as mx +from mxnet import nd, autograd, gluon +from mxnet.gluon import nn, Trainer +from mxnet.gluon.data import DataLoader, ArrayDataset + +mx.random.seed(12345) # Added for reproducibility +``` + +In this tutorial we will use fake dataset, which contains 10 features drawn from a normal distribution with mean equals to 0 and standard deviation equals to 1, and a class label, which can be either 0 or 1. The length of the dataset is an arbitrary value. The function below helps us to generate a dataset. + + +```python +def get_random_data(size, ctx): +x = nd.normal(0, 1, shape=(size, 10), ctx=ctx) +# Class label is generated via non-random logic so the network would have a pattern to look for +# Number 3 is selected to make sure that number of positive examples smaller than negative, but not too small +y = x.sum(axis=1) > 3 +return x, y +``` + +Also, let's define a set of hyperparameters, that we are going to use later. Since our model is simple and dataset is small, we are going to use CPU for calculations. Feel free to change it to GPU for a more advanced scenario. + + +```python +ctx = mx.cpu() +train_data_size = 1000 +val_data_size = 100 +batch_size = 10 +``` + +## Working with data + +To work with data, Apache MXNet provides Dataset and DataLoader classes. The former is used to provide an indexed access to the data, the latter is used to shuffle and batchify the data. + +This separation is done because a source of Dataset can vary from a simple array of numbers to complex data structures like text and images. DataLoader doesn't need to be aware of the source of data as long as Dataset provides a way to get the number of records and to load a record by index. As an outcome, Dataset doesn't need to hold in memory all data at once. Needless to say, that one can implement its own versions of Dataset and DataLoader, but we are going to use existing implementation. + +Below we define 2 datasets: training dataset and validation dataset. It is a good practice to measure performance of a trained model on a data that the network hasn't seen before. That is why we are going to use training set for training the model and validation set to calculate model's accuracy. + + +```python +train_x, train_ground_truth_class = get_random_data(train_data_size, ctx) +train_dataset = ArrayDataset(train_x, train_ground_truth_class) +train_dataloader = DataLoader(train_dataset, batch_size=batch_size, shuffle=True) + +val_x, val_ground_truth_class = get_random_data(val_data_size, ctx) +val_dataset = ArrayDataset(val_x, val_ground_truth_class) +val_dataloader = DataLoader(val_dataset, batch_size=batch_size, shuffle=True) +``` + +## Defining and training the model + +In real application, model can be arbitrary complex. The only requirement for the logistic regression is that the last layer of the network must be a single neuron. Apache MXNet allows us to do so by using `Dense` layer and specifying the number of units to 1. + +Below, we define a model which has an input layer of 10 neurons, a couple inner layers of 10 neurons each, and output layer of 1 neuron, as it is required by logistic regression. We stack the layers using `HybridSequential` block and initialize parameters of the network using `Xavier` initialization. + + +```python +net = nn.HybridSequential() + +with net.name_scope(): +net.add(nn.Dense(units=10, activation='relu')) # input layer +net.add(nn.Dense(units=10, activation='relu')) # inner layer 1 +net.add(nn.Dense(units=10, activation='relu')) # inner layer 2 +net.add(nn.Dense(units=1)) # output layer: notice, it must have only 1 neuron + +net.initialize(mx.init.Xavier(magnitude=2.34)) +``` + +After defining the model, we need to define a few more thing: our loss, our trainer and our metric. + +Loss function is used to calculate how the output of the network different from the ground truth. In case of the logistic regression the ground truth are class labels, which can be either 0 or 1. Because of that, we are using `SigmoidBinaryCrossEntropyLoss`, which suites well for that scenario. + +Trainer object allows to specify the method of training to be used. There are various methods available, and for our tutorial we use a widely accepted method Stochastic Gradient Descent. We
[GitHub] safrooze commented on a change in pull request #11651: Add logistic regression tutorial
safrooze commented on a change in pull request #11651: Add logistic regression tutorial URL: https://github.com/apache/incubator-mxnet/pull/11651#discussion_r202896734 ## File path: docs/tutorials/gluon/logistic_regression_explained.md ## @@ -0,0 +1,215 @@ + +# Logistic regression using Gluon API explained + +Logistic Regression is one of the first models newcomers to Deep Learning are implementing. In this tutorial I am going to focus on how to do logistic regression using Gluon API and provide some high level tips. + +Before anything else, let's import required packages for this tutorial. + + +```python +import numpy as np +import mxnet as mx +from mxnet import nd, autograd, gluon +from mxnet.gluon import nn, Trainer +from mxnet.gluon.data import DataLoader, ArrayDataset + +mx.random.seed(12345) # Added for reproducibility +``` + +In this tutorial we will use fake dataset, which contains 10 features drawn from a normal distribution with mean equals to 0 and standard deviation equals to 1, and a class label, which can be either 0 or 1. The length of the dataset is an arbitrary value. The function below helps us to generate a dataset. + + +```python +def get_random_data(size, ctx): +x = nd.normal(0, 1, shape=(size, 10), ctx=ctx) +# Class label is generated via non-random logic so the network would have a pattern to look for +# Number 3 is selected to make sure that number of positive examples smaller than negative, but not too small +y = x.sum(axis=1) > 3 +return x, y +``` + +Also, let's define a set of hyperparameters, that we are going to use later. Since our model is simple and dataset is small, we are going to use CPU for calculations. Feel free to change it to GPU for a more advanced scenario. + + +```python +ctx = mx.cpu() +train_data_size = 1000 +val_data_size = 100 +batch_size = 10 +``` + +## Working with data + +To work with data, Apache MXNet provides Dataset and DataLoader classes. The former is used to provide an indexed access to the data, the latter is used to shuffle and batchify the data. + +This separation is done because a source of Dataset can vary from a simple array of numbers to complex data structures like text and images. DataLoader doesn't need to be aware of the source of data as long as Dataset provides a way to get the number of records and to load a record by index. As an outcome, Dataset doesn't need to hold in memory all data at once. Needless to say, that one can implement its own versions of Dataset and DataLoader, but we are going to use existing implementation. + +Below we define 2 datasets: training dataset and validation dataset. It is a good practice to measure performance of a trained model on a data that the network hasn't seen before. That is why we are going to use training set for training the model and validation set to calculate model's accuracy. + + +```python +train_x, train_ground_truth_class = get_random_data(train_data_size, ctx) +train_dataset = ArrayDataset(train_x, train_ground_truth_class) +train_dataloader = DataLoader(train_dataset, batch_size=batch_size, shuffle=True) + +val_x, val_ground_truth_class = get_random_data(val_data_size, ctx) +val_dataset = ArrayDataset(val_x, val_ground_truth_class) +val_dataloader = DataLoader(val_dataset, batch_size=batch_size, shuffle=True) +``` + +## Defining and training the model + +In real application, model can be arbitrary complex. The only requirement for the logistic regression is that the last layer of the network must be a single neuron. Apache MXNet allows us to do so by using `Dense` layer and specifying the number of units to 1. + +Below, we define a model which has an input layer of 10 neurons, a couple inner layers of 10 neurons each, and output layer of 1 neuron, as it is required by logistic regression. We stack the layers using `HybridSequential` block and initialize parameters of the network using `Xavier` initialization. + + +```python +net = nn.HybridSequential() + +with net.name_scope(): +net.add(nn.Dense(units=10, activation='relu')) # input layer +net.add(nn.Dense(units=10, activation='relu')) # inner layer 1 +net.add(nn.Dense(units=10, activation='relu')) # inner layer 2 +net.add(nn.Dense(units=1)) # output layer: notice, it must have only 1 neuron + +net.initialize(mx.init.Xavier(magnitude=2.34)) +``` + +After defining the model, we need to define a few more thing: our loss, our trainer and our metric. + +Loss function is used to calculate how the output of the network different from the ground truth. In case of the logistic regression the ground truth are class labels, which can be either 0 or 1. Because of that, we are using `SigmoidBinaryCrossEntropyLoss`, which suites well for that scenario. + +Trainer object allows to specify the method of training to be used. There are various methods available, and for our tutorial we use a widely accepted method Stochastic Gradient Descent. We
[GitHub] safrooze commented on a change in pull request #11651: Add logistic regression tutorial
safrooze commented on a change in pull request #11651: Add logistic regression tutorial URL: https://github.com/apache/incubator-mxnet/pull/11651#discussion_r202893582 ## File path: docs/tutorials/gluon/logistic_regression_explained.md ## @@ -0,0 +1,215 @@ + +# Logistic regression using Gluon API explained + +Logistic Regression is one of the first models newcomers to Deep Learning are implementing. In this tutorial I am going to focus on how to do logistic regression using Gluon API and provide some high level tips. + +Before anything else, let's import required packages for this tutorial. + + +```python +import numpy as np +import mxnet as mx +from mxnet import nd, autograd, gluon +from mxnet.gluon import nn, Trainer +from mxnet.gluon.data import DataLoader, ArrayDataset + +mx.random.seed(12345) # Added for reproducibility +``` + +In this tutorial we will use fake dataset, which contains 10 features drawn from a normal distribution with mean equals to 0 and standard deviation equals to 1, and a class label, which can be either 0 or 1. The length of the dataset is an arbitrary value. The function below helps us to generate a dataset. + + +```python +def get_random_data(size, ctx): +x = nd.normal(0, 1, shape=(size, 10), ctx=ctx) +# Class label is generated via non-random logic so the network would have a pattern to look for +# Number 3 is selected to make sure that number of positive examples smaller than negative, but not too small +y = x.sum(axis=1) > 3 +return x, y +``` + +Also, let's define a set of hyperparameters, that we are going to use later. Since our model is simple and dataset is small, we are going to use CPU for calculations. Feel free to change it to GPU for a more advanced scenario. + + +```python +ctx = mx.cpu() +train_data_size = 1000 +val_data_size = 100 +batch_size = 10 +``` + +## Working with data + +To work with data, Apache MXNet provides Dataset and DataLoader classes. The former is used to provide an indexed access to the data, the latter is used to shuffle and batchify the data. + +This separation is done because a source of Dataset can vary from a simple array of numbers to complex data structures like text and images. DataLoader doesn't need to be aware of the source of data as long as Dataset provides a way to get the number of records and to load a record by index. As an outcome, Dataset doesn't need to hold in memory all data at once. Needless to say, that one can implement its own versions of Dataset and DataLoader, but we are going to use existing implementation. + +Below we define 2 datasets: training dataset and validation dataset. It is a good practice to measure performance of a trained model on a data that the network hasn't seen before. That is why we are going to use training set for training the model and validation set to calculate model's accuracy. + + +```python +train_x, train_ground_truth_class = get_random_data(train_data_size, ctx) +train_dataset = ArrayDataset(train_x, train_ground_truth_class) +train_dataloader = DataLoader(train_dataset, batch_size=batch_size, shuffle=True) + +val_x, val_ground_truth_class = get_random_data(val_data_size, ctx) +val_dataset = ArrayDataset(val_x, val_ground_truth_class) +val_dataloader = DataLoader(val_dataset, batch_size=batch_size, shuffle=True) +``` + +## Defining and training the model + +In real application, model can be arbitrary complex. The only requirement for the logistic regression is that the last layer of the network must be a single neuron. Apache MXNet allows us to do so by using `Dense` layer and specifying the number of units to 1. + +Below, we define a model which has an input layer of 10 neurons, a couple inner layers of 10 neurons each, and output layer of 1 neuron, as it is required by logistic regression. We stack the layers using `HybridSequential` block and initialize parameters of the network using `Xavier` initialization. Review comment: Link to API. Also, "a couple ***of*** inner layers" This is an automated message from the Apache Git Service. To respond to the message, please log on GitHub and use the URL above to go to the specific comment. For queries about this service, please contact Infrastructure at: us...@infra.apache.org With regards, Apache Git Services
[GitHub] safrooze commented on a change in pull request #11651: Add logistic regression tutorial
safrooze commented on a change in pull request #11651: Add logistic regression tutorial URL: https://github.com/apache/incubator-mxnet/pull/11651#discussion_r202894728 ## File path: docs/tutorials/gluon/logistic_regression_explained.md ## @@ -0,0 +1,215 @@ + +# Logistic regression using Gluon API explained + +Logistic Regression is one of the first models newcomers to Deep Learning are implementing. In this tutorial I am going to focus on how to do logistic regression using Gluon API and provide some high level tips. + +Before anything else, let's import required packages for this tutorial. + + +```python +import numpy as np +import mxnet as mx +from mxnet import nd, autograd, gluon +from mxnet.gluon import nn, Trainer +from mxnet.gluon.data import DataLoader, ArrayDataset + +mx.random.seed(12345) # Added for reproducibility +``` + +In this tutorial we will use fake dataset, which contains 10 features drawn from a normal distribution with mean equals to 0 and standard deviation equals to 1, and a class label, which can be either 0 or 1. The length of the dataset is an arbitrary value. The function below helps us to generate a dataset. + + +```python +def get_random_data(size, ctx): +x = nd.normal(0, 1, shape=(size, 10), ctx=ctx) +# Class label is generated via non-random logic so the network would have a pattern to look for +# Number 3 is selected to make sure that number of positive examples smaller than negative, but not too small +y = x.sum(axis=1) > 3 +return x, y +``` + +Also, let's define a set of hyperparameters, that we are going to use later. Since our model is simple and dataset is small, we are going to use CPU for calculations. Feel free to change it to GPU for a more advanced scenario. + + +```python +ctx = mx.cpu() +train_data_size = 1000 +val_data_size = 100 +batch_size = 10 +``` + +## Working with data + +To work with data, Apache MXNet provides Dataset and DataLoader classes. The former is used to provide an indexed access to the data, the latter is used to shuffle and batchify the data. + +This separation is done because a source of Dataset can vary from a simple array of numbers to complex data structures like text and images. DataLoader doesn't need to be aware of the source of data as long as Dataset provides a way to get the number of records and to load a record by index. As an outcome, Dataset doesn't need to hold in memory all data at once. Needless to say, that one can implement its own versions of Dataset and DataLoader, but we are going to use existing implementation. + +Below we define 2 datasets: training dataset and validation dataset. It is a good practice to measure performance of a trained model on a data that the network hasn't seen before. That is why we are going to use training set for training the model and validation set to calculate model's accuracy. + + +```python +train_x, train_ground_truth_class = get_random_data(train_data_size, ctx) +train_dataset = ArrayDataset(train_x, train_ground_truth_class) +train_dataloader = DataLoader(train_dataset, batch_size=batch_size, shuffle=True) + +val_x, val_ground_truth_class = get_random_data(val_data_size, ctx) +val_dataset = ArrayDataset(val_x, val_ground_truth_class) +val_dataloader = DataLoader(val_dataset, batch_size=batch_size, shuffle=True) +``` + +## Defining and training the model + +In real application, model can be arbitrary complex. The only requirement for the logistic regression is that the last layer of the network must be a single neuron. Apache MXNet allows us to do so by using `Dense` layer and specifying the number of units to 1. + +Below, we define a model which has an input layer of 10 neurons, a couple inner layers of 10 neurons each, and output layer of 1 neuron, as it is required by logistic regression. We stack the layers using `HybridSequential` block and initialize parameters of the network using `Xavier` initialization. + + +```python +net = nn.HybridSequential() + +with net.name_scope(): +net.add(nn.Dense(units=10, activation='relu')) # input layer +net.add(nn.Dense(units=10, activation='relu')) # inner layer 1 +net.add(nn.Dense(units=10, activation='relu')) # inner layer 2 +net.add(nn.Dense(units=1)) # output layer: notice, it must have only 1 neuron + +net.initialize(mx.init.Xavier(magnitude=2.34)) +``` + +After defining the model, we need to define a few more thing: our loss, our trainer and our metric. + +Loss function is used to calculate how the output of the network different from the ground truth. In case of the logistic regression the ground truth are class labels, which can be either 0 or 1. Because of that, we are using `SigmoidBinaryCrossEntropyLoss`, which suites well for that scenario. + +Trainer object allows to specify the method of training to be used. There are various methods available, and for our tutorial we use a widely accepted method Stochastic Gradient Descent. We
[GitHub] safrooze commented on a change in pull request #11651: Add logistic regression tutorial
safrooze commented on a change in pull request #11651: Add logistic regression tutorial URL: https://github.com/apache/incubator-mxnet/pull/11651#discussion_r202895749 ## File path: docs/tutorials/gluon/logistic_regression_explained.md ## @@ -0,0 +1,215 @@ + +# Logistic regression using Gluon API explained + +Logistic Regression is one of the first models newcomers to Deep Learning are implementing. In this tutorial I am going to focus on how to do logistic regression using Gluon API and provide some high level tips. + +Before anything else, let's import required packages for this tutorial. + + +```python +import numpy as np +import mxnet as mx +from mxnet import nd, autograd, gluon +from mxnet.gluon import nn, Trainer +from mxnet.gluon.data import DataLoader, ArrayDataset + +mx.random.seed(12345) # Added for reproducibility +``` + +In this tutorial we will use fake dataset, which contains 10 features drawn from a normal distribution with mean equals to 0 and standard deviation equals to 1, and a class label, which can be either 0 or 1. The length of the dataset is an arbitrary value. The function below helps us to generate a dataset. + + +```python +def get_random_data(size, ctx): +x = nd.normal(0, 1, shape=(size, 10), ctx=ctx) +# Class label is generated via non-random logic so the network would have a pattern to look for +# Number 3 is selected to make sure that number of positive examples smaller than negative, but not too small +y = x.sum(axis=1) > 3 +return x, y +``` + +Also, let's define a set of hyperparameters, that we are going to use later. Since our model is simple and dataset is small, we are going to use CPU for calculations. Feel free to change it to GPU for a more advanced scenario. + + +```python +ctx = mx.cpu() +train_data_size = 1000 +val_data_size = 100 +batch_size = 10 +``` + +## Working with data + +To work with data, Apache MXNet provides Dataset and DataLoader classes. The former is used to provide an indexed access to the data, the latter is used to shuffle and batchify the data. + +This separation is done because a source of Dataset can vary from a simple array of numbers to complex data structures like text and images. DataLoader doesn't need to be aware of the source of data as long as Dataset provides a way to get the number of records and to load a record by index. As an outcome, Dataset doesn't need to hold in memory all data at once. Needless to say, that one can implement its own versions of Dataset and DataLoader, but we are going to use existing implementation. + +Below we define 2 datasets: training dataset and validation dataset. It is a good practice to measure performance of a trained model on a data that the network hasn't seen before. That is why we are going to use training set for training the model and validation set to calculate model's accuracy. + + +```python +train_x, train_ground_truth_class = get_random_data(train_data_size, ctx) +train_dataset = ArrayDataset(train_x, train_ground_truth_class) +train_dataloader = DataLoader(train_dataset, batch_size=batch_size, shuffle=True) + +val_x, val_ground_truth_class = get_random_data(val_data_size, ctx) +val_dataset = ArrayDataset(val_x, val_ground_truth_class) +val_dataloader = DataLoader(val_dataset, batch_size=batch_size, shuffle=True) +``` + +## Defining and training the model + +In real application, model can be arbitrary complex. The only requirement for the logistic regression is that the last layer of the network must be a single neuron. Apache MXNet allows us to do so by using `Dense` layer and specifying the number of units to 1. + +Below, we define a model which has an input layer of 10 neurons, a couple inner layers of 10 neurons each, and output layer of 1 neuron, as it is required by logistic regression. We stack the layers using `HybridSequential` block and initialize parameters of the network using `Xavier` initialization. + + +```python +net = nn.HybridSequential() + +with net.name_scope(): +net.add(nn.Dense(units=10, activation='relu')) # input layer +net.add(nn.Dense(units=10, activation='relu')) # inner layer 1 +net.add(nn.Dense(units=10, activation='relu')) # inner layer 2 +net.add(nn.Dense(units=1)) # output layer: notice, it must have only 1 neuron + +net.initialize(mx.init.Xavier(magnitude=2.34)) +``` + +After defining the model, we need to define a few more thing: our loss, our trainer and our metric. + +Loss function is used to calculate how the output of the network different from the ground truth. In case of the logistic regression the ground truth are class labels, which can be either 0 or 1. Because of that, we are using `SigmoidBinaryCrossEntropyLoss`, which suites well for that scenario. + +Trainer object allows to specify the method of training to be used. There are various methods available, and for our tutorial we use a widely accepted method Stochastic Gradient Descent. We
[GitHub] safrooze commented on a change in pull request #11651: Add logistic regression tutorial
safrooze commented on a change in pull request #11651: Add logistic regression tutorial URL: https://github.com/apache/incubator-mxnet/pull/11651#discussion_r202897215 ## File path: docs/tutorials/gluon/logistic_regression_explained.md ## @@ -0,0 +1,215 @@ + +# Logistic regression using Gluon API explained + +Logistic Regression is one of the first models newcomers to Deep Learning are implementing. In this tutorial I am going to focus on how to do logistic regression using Gluon API and provide some high level tips. + +Before anything else, let's import required packages for this tutorial. + + +```python +import numpy as np +import mxnet as mx +from mxnet import nd, autograd, gluon +from mxnet.gluon import nn, Trainer +from mxnet.gluon.data import DataLoader, ArrayDataset + +mx.random.seed(12345) # Added for reproducibility +``` + +In this tutorial we will use fake dataset, which contains 10 features drawn from a normal distribution with mean equals to 0 and standard deviation equals to 1, and a class label, which can be either 0 or 1. The length of the dataset is an arbitrary value. The function below helps us to generate a dataset. + + +```python +def get_random_data(size, ctx): +x = nd.normal(0, 1, shape=(size, 10), ctx=ctx) +# Class label is generated via non-random logic so the network would have a pattern to look for +# Number 3 is selected to make sure that number of positive examples smaller than negative, but not too small +y = x.sum(axis=1) > 3 +return x, y +``` + +Also, let's define a set of hyperparameters, that we are going to use later. Since our model is simple and dataset is small, we are going to use CPU for calculations. Feel free to change it to GPU for a more advanced scenario. + + +```python +ctx = mx.cpu() +train_data_size = 1000 +val_data_size = 100 +batch_size = 10 +``` + +## Working with data + +To work with data, Apache MXNet provides Dataset and DataLoader classes. The former is used to provide an indexed access to the data, the latter is used to shuffle and batchify the data. + +This separation is done because a source of Dataset can vary from a simple array of numbers to complex data structures like text and images. DataLoader doesn't need to be aware of the source of data as long as Dataset provides a way to get the number of records and to load a record by index. As an outcome, Dataset doesn't need to hold in memory all data at once. Needless to say, that one can implement its own versions of Dataset and DataLoader, but we are going to use existing implementation. + +Below we define 2 datasets: training dataset and validation dataset. It is a good practice to measure performance of a trained model on a data that the network hasn't seen before. That is why we are going to use training set for training the model and validation set to calculate model's accuracy. + + +```python +train_x, train_ground_truth_class = get_random_data(train_data_size, ctx) +train_dataset = ArrayDataset(train_x, train_ground_truth_class) +train_dataloader = DataLoader(train_dataset, batch_size=batch_size, shuffle=True) + +val_x, val_ground_truth_class = get_random_data(val_data_size, ctx) +val_dataset = ArrayDataset(val_x, val_ground_truth_class) +val_dataloader = DataLoader(val_dataset, batch_size=batch_size, shuffle=True) +``` + +## Defining and training the model + +In real application, model can be arbitrary complex. The only requirement for the logistic regression is that the last layer of the network must be a single neuron. Apache MXNet allows us to do so by using `Dense` layer and specifying the number of units to 1. + +Below, we define a model which has an input layer of 10 neurons, a couple inner layers of 10 neurons each, and output layer of 1 neuron, as it is required by logistic regression. We stack the layers using `HybridSequential` block and initialize parameters of the network using `Xavier` initialization. + + +```python +net = nn.HybridSequential() + +with net.name_scope(): +net.add(nn.Dense(units=10, activation='relu')) # input layer +net.add(nn.Dense(units=10, activation='relu')) # inner layer 1 +net.add(nn.Dense(units=10, activation='relu')) # inner layer 2 +net.add(nn.Dense(units=1)) # output layer: notice, it must have only 1 neuron + +net.initialize(mx.init.Xavier(magnitude=2.34)) +``` + +After defining the model, we need to define a few more thing: our loss, our trainer and our metric. + +Loss function is used to calculate how the output of the network different from the ground truth. In case of the logistic regression the ground truth are class labels, which can be either 0 or 1. Because of that, we are using `SigmoidBinaryCrossEntropyLoss`, which suites well for that scenario. + +Trainer object allows to specify the method of training to be used. There are various methods available, and for our tutorial we use a widely accepted method Stochastic Gradient Descent. We
[GitHub] safrooze commented on a change in pull request #11651: Add logistic regression tutorial
safrooze commented on a change in pull request #11651: Add logistic regression tutorial URL: https://github.com/apache/incubator-mxnet/pull/11651#discussion_r202893081 ## File path: docs/tutorials/gluon/logistic_regression_explained.md ## @@ -0,0 +1,215 @@ + +# Logistic regression using Gluon API explained + +Logistic Regression is one of the first models newcomers to Deep Learning are implementing. In this tutorial I am going to focus on how to do logistic regression using Gluon API and provide some high level tips. + +Before anything else, let's import required packages for this tutorial. + + +```python +import numpy as np +import mxnet as mx +from mxnet import nd, autograd, gluon +from mxnet.gluon import nn, Trainer +from mxnet.gluon.data import DataLoader, ArrayDataset + +mx.random.seed(12345) # Added for reproducibility +``` + +In this tutorial we will use fake dataset, which contains 10 features drawn from a normal distribution with mean equals to 0 and standard deviation equals to 1, and a class label, which can be either 0 or 1. The length of the dataset is an arbitrary value. The function below helps us to generate a dataset. + + +```python +def get_random_data(size, ctx): +x = nd.normal(0, 1, shape=(size, 10), ctx=ctx) +# Class label is generated via non-random logic so the network would have a pattern to look for +# Number 3 is selected to make sure that number of positive examples smaller than negative, but not too small +y = x.sum(axis=1) > 3 +return x, y +``` + +Also, let's define a set of hyperparameters, that we are going to use later. Since our model is simple and dataset is small, we are going to use CPU for calculations. Feel free to change it to GPU for a more advanced scenario. + + +```python +ctx = mx.cpu() +train_data_size = 1000 +val_data_size = 100 +batch_size = 10 +``` + +## Working with data + +To work with data, Apache MXNet provides Dataset and DataLoader classes. The former is used to provide an indexed access to the data, the latter is used to shuffle and batchify the data. Review comment: Point user to links to API doc and tutorials for further reading and perhaps skip explanation of dataset/dataloader. This is an automated message from the Apache Git Service. To respond to the message, please log on GitHub and use the URL above to go to the specific comment. For queries about this service, please contact Infrastructure at: us...@infra.apache.org With regards, Apache Git Services
[GitHub] safrooze commented on a change in pull request #11651: Add logistic regression tutorial
safrooze commented on a change in pull request #11651: Add logistic regression tutorial URL: https://github.com/apache/incubator-mxnet/pull/11651#discussion_r202895055 ## File path: docs/tutorials/gluon/logistic_regression_explained.md ## @@ -0,0 +1,215 @@ + +# Logistic regression using Gluon API explained + +Logistic Regression is one of the first models newcomers to Deep Learning are implementing. In this tutorial I am going to focus on how to do logistic regression using Gluon API and provide some high level tips. + +Before anything else, let's import required packages for this tutorial. + + +```python +import numpy as np +import mxnet as mx +from mxnet import nd, autograd, gluon +from mxnet.gluon import nn, Trainer +from mxnet.gluon.data import DataLoader, ArrayDataset + +mx.random.seed(12345) # Added for reproducibility +``` + +In this tutorial we will use fake dataset, which contains 10 features drawn from a normal distribution with mean equals to 0 and standard deviation equals to 1, and a class label, which can be either 0 or 1. The length of the dataset is an arbitrary value. The function below helps us to generate a dataset. + + +```python +def get_random_data(size, ctx): +x = nd.normal(0, 1, shape=(size, 10), ctx=ctx) +# Class label is generated via non-random logic so the network would have a pattern to look for +# Number 3 is selected to make sure that number of positive examples smaller than negative, but not too small +y = x.sum(axis=1) > 3 +return x, y +``` + +Also, let's define a set of hyperparameters, that we are going to use later. Since our model is simple and dataset is small, we are going to use CPU for calculations. Feel free to change it to GPU for a more advanced scenario. + + +```python +ctx = mx.cpu() +train_data_size = 1000 +val_data_size = 100 +batch_size = 10 +``` + +## Working with data + +To work with data, Apache MXNet provides Dataset and DataLoader classes. The former is used to provide an indexed access to the data, the latter is used to shuffle and batchify the data. + +This separation is done because a source of Dataset can vary from a simple array of numbers to complex data structures like text and images. DataLoader doesn't need to be aware of the source of data as long as Dataset provides a way to get the number of records and to load a record by index. As an outcome, Dataset doesn't need to hold in memory all data at once. Needless to say, that one can implement its own versions of Dataset and DataLoader, but we are going to use existing implementation. + +Below we define 2 datasets: training dataset and validation dataset. It is a good practice to measure performance of a trained model on a data that the network hasn't seen before. That is why we are going to use training set for training the model and validation set to calculate model's accuracy. + + +```python +train_x, train_ground_truth_class = get_random_data(train_data_size, ctx) +train_dataset = ArrayDataset(train_x, train_ground_truth_class) +train_dataloader = DataLoader(train_dataset, batch_size=batch_size, shuffle=True) + +val_x, val_ground_truth_class = get_random_data(val_data_size, ctx) +val_dataset = ArrayDataset(val_x, val_ground_truth_class) +val_dataloader = DataLoader(val_dataset, batch_size=batch_size, shuffle=True) +``` + +## Defining and training the model + +In real application, model can be arbitrary complex. The only requirement for the logistic regression is that the last layer of the network must be a single neuron. Apache MXNet allows us to do so by using `Dense` layer and specifying the number of units to 1. + +Below, we define a model which has an input layer of 10 neurons, a couple inner layers of 10 neurons each, and output layer of 1 neuron, as it is required by logistic regression. We stack the layers using `HybridSequential` block and initialize parameters of the network using `Xavier` initialization. + + +```python +net = nn.HybridSequential() + +with net.name_scope(): +net.add(nn.Dense(units=10, activation='relu')) # input layer +net.add(nn.Dense(units=10, activation='relu')) # inner layer 1 +net.add(nn.Dense(units=10, activation='relu')) # inner layer 2 +net.add(nn.Dense(units=1)) # output layer: notice, it must have only 1 neuron + +net.initialize(mx.init.Xavier(magnitude=2.34)) +``` + +After defining the model, we need to define a few more thing: our loss, our trainer and our metric. + +Loss function is used to calculate how the output of the network different from the ground truth. In case of the logistic regression the ground truth are class labels, which can be either 0 or 1. Because of that, we are using `SigmoidBinaryCrossEntropyLoss`, which suites well for that scenario. + +Trainer object allows to specify the method of training to be used. There are various methods available, and for our tutorial we use a widely accepted method Stochastic Gradient Descent. We
[GitHub] safrooze commented on a change in pull request #11651: Add logistic regression tutorial
safrooze commented on a change in pull request #11651: Add logistic regression tutorial URL: https://github.com/apache/incubator-mxnet/pull/11651#discussion_r202895767 ## File path: docs/tutorials/gluon/logistic_regression_explained.md ## @@ -0,0 +1,215 @@ + +# Logistic regression using Gluon API explained + +Logistic Regression is one of the first models newcomers to Deep Learning are implementing. In this tutorial I am going to focus on how to do logistic regression using Gluon API and provide some high level tips. + +Before anything else, let's import required packages for this tutorial. + + +```python +import numpy as np +import mxnet as mx +from mxnet import nd, autograd, gluon +from mxnet.gluon import nn, Trainer +from mxnet.gluon.data import DataLoader, ArrayDataset + +mx.random.seed(12345) # Added for reproducibility +``` + +In this tutorial we will use fake dataset, which contains 10 features drawn from a normal distribution with mean equals to 0 and standard deviation equals to 1, and a class label, which can be either 0 or 1. The length of the dataset is an arbitrary value. The function below helps us to generate a dataset. + + +```python +def get_random_data(size, ctx): +x = nd.normal(0, 1, shape=(size, 10), ctx=ctx) +# Class label is generated via non-random logic so the network would have a pattern to look for +# Number 3 is selected to make sure that number of positive examples smaller than negative, but not too small +y = x.sum(axis=1) > 3 +return x, y +``` + +Also, let's define a set of hyperparameters, that we are going to use later. Since our model is simple and dataset is small, we are going to use CPU for calculations. Feel free to change it to GPU for a more advanced scenario. + + +```python +ctx = mx.cpu() +train_data_size = 1000 +val_data_size = 100 +batch_size = 10 +``` + +## Working with data + +To work with data, Apache MXNet provides Dataset and DataLoader classes. The former is used to provide an indexed access to the data, the latter is used to shuffle and batchify the data. + +This separation is done because a source of Dataset can vary from a simple array of numbers to complex data structures like text and images. DataLoader doesn't need to be aware of the source of data as long as Dataset provides a way to get the number of records and to load a record by index. As an outcome, Dataset doesn't need to hold in memory all data at once. Needless to say, that one can implement its own versions of Dataset and DataLoader, but we are going to use existing implementation. + +Below we define 2 datasets: training dataset and validation dataset. It is a good practice to measure performance of a trained model on a data that the network hasn't seen before. That is why we are going to use training set for training the model and validation set to calculate model's accuracy. + + +```python +train_x, train_ground_truth_class = get_random_data(train_data_size, ctx) +train_dataset = ArrayDataset(train_x, train_ground_truth_class) +train_dataloader = DataLoader(train_dataset, batch_size=batch_size, shuffle=True) + +val_x, val_ground_truth_class = get_random_data(val_data_size, ctx) +val_dataset = ArrayDataset(val_x, val_ground_truth_class) +val_dataloader = DataLoader(val_dataset, batch_size=batch_size, shuffle=True) +``` + +## Defining and training the model + +In real application, model can be arbitrary complex. The only requirement for the logistic regression is that the last layer of the network must be a single neuron. Apache MXNet allows us to do so by using `Dense` layer and specifying the number of units to 1. + +Below, we define a model which has an input layer of 10 neurons, a couple inner layers of 10 neurons each, and output layer of 1 neuron, as it is required by logistic regression. We stack the layers using `HybridSequential` block and initialize parameters of the network using `Xavier` initialization. + + +```python +net = nn.HybridSequential() + +with net.name_scope(): +net.add(nn.Dense(units=10, activation='relu')) # input layer +net.add(nn.Dense(units=10, activation='relu')) # inner layer 1 +net.add(nn.Dense(units=10, activation='relu')) # inner layer 2 +net.add(nn.Dense(units=1)) # output layer: notice, it must have only 1 neuron + +net.initialize(mx.init.Xavier(magnitude=2.34)) +``` + +After defining the model, we need to define a few more thing: our loss, our trainer and our metric. + +Loss function is used to calculate how the output of the network different from the ground truth. In case of the logistic regression the ground truth are class labels, which can be either 0 or 1. Because of that, we are using `SigmoidBinaryCrossEntropyLoss`, which suites well for that scenario. + +Trainer object allows to specify the method of training to be used. There are various methods available, and for our tutorial we use a widely accepted method Stochastic Gradient Descent. We
[GitHub] safrooze commented on a change in pull request #11651: Add logistic regression tutorial
safrooze commented on a change in pull request #11651: Add logistic regression tutorial URL: https://github.com/apache/incubator-mxnet/pull/11651#discussion_r202895806 ## File path: docs/tutorials/gluon/logistic_regression_explained.md ## @@ -0,0 +1,215 @@ + +# Logistic regression using Gluon API explained + +Logistic Regression is one of the first models newcomers to Deep Learning are implementing. In this tutorial I am going to focus on how to do logistic regression using Gluon API and provide some high level tips. + +Before anything else, let's import required packages for this tutorial. + + +```python +import numpy as np +import mxnet as mx +from mxnet import nd, autograd, gluon +from mxnet.gluon import nn, Trainer +from mxnet.gluon.data import DataLoader, ArrayDataset + +mx.random.seed(12345) # Added for reproducibility +``` + +In this tutorial we will use fake dataset, which contains 10 features drawn from a normal distribution with mean equals to 0 and standard deviation equals to 1, and a class label, which can be either 0 or 1. The length of the dataset is an arbitrary value. The function below helps us to generate a dataset. + + +```python +def get_random_data(size, ctx): +x = nd.normal(0, 1, shape=(size, 10), ctx=ctx) +# Class label is generated via non-random logic so the network would have a pattern to look for +# Number 3 is selected to make sure that number of positive examples smaller than negative, but not too small +y = x.sum(axis=1) > 3 +return x, y +``` + +Also, let's define a set of hyperparameters, that we are going to use later. Since our model is simple and dataset is small, we are going to use CPU for calculations. Feel free to change it to GPU for a more advanced scenario. + + +```python +ctx = mx.cpu() +train_data_size = 1000 +val_data_size = 100 +batch_size = 10 +``` + +## Working with data + +To work with data, Apache MXNet provides Dataset and DataLoader classes. The former is used to provide an indexed access to the data, the latter is used to shuffle and batchify the data. + +This separation is done because a source of Dataset can vary from a simple array of numbers to complex data structures like text and images. DataLoader doesn't need to be aware of the source of data as long as Dataset provides a way to get the number of records and to load a record by index. As an outcome, Dataset doesn't need to hold in memory all data at once. Needless to say, that one can implement its own versions of Dataset and DataLoader, but we are going to use existing implementation. + +Below we define 2 datasets: training dataset and validation dataset. It is a good practice to measure performance of a trained model on a data that the network hasn't seen before. That is why we are going to use training set for training the model and validation set to calculate model's accuracy. + + +```python +train_x, train_ground_truth_class = get_random_data(train_data_size, ctx) +train_dataset = ArrayDataset(train_x, train_ground_truth_class) +train_dataloader = DataLoader(train_dataset, batch_size=batch_size, shuffle=True) + +val_x, val_ground_truth_class = get_random_data(val_data_size, ctx) +val_dataset = ArrayDataset(val_x, val_ground_truth_class) +val_dataloader = DataLoader(val_dataset, batch_size=batch_size, shuffle=True) +``` + +## Defining and training the model + +In real application, model can be arbitrary complex. The only requirement for the logistic regression is that the last layer of the network must be a single neuron. Apache MXNet allows us to do so by using `Dense` layer and specifying the number of units to 1. + +Below, we define a model which has an input layer of 10 neurons, a couple inner layers of 10 neurons each, and output layer of 1 neuron, as it is required by logistic regression. We stack the layers using `HybridSequential` block and initialize parameters of the network using `Xavier` initialization. + + +```python +net = nn.HybridSequential() + +with net.name_scope(): +net.add(nn.Dense(units=10, activation='relu')) # input layer +net.add(nn.Dense(units=10, activation='relu')) # inner layer 1 +net.add(nn.Dense(units=10, activation='relu')) # inner layer 2 +net.add(nn.Dense(units=1)) # output layer: notice, it must have only 1 neuron + +net.initialize(mx.init.Xavier(magnitude=2.34)) +``` + +After defining the model, we need to define a few more thing: our loss, our trainer and our metric. + +Loss function is used to calculate how the output of the network different from the ground truth. In case of the logistic regression the ground truth are class labels, which can be either 0 or 1. Because of that, we are using `SigmoidBinaryCrossEntropyLoss`, which suites well for that scenario. + +Trainer object allows to specify the method of training to be used. There are various methods available, and for our tutorial we use a widely accepted method Stochastic Gradient Descent. We
[GitHub] lupesko commented on a change in pull request #11528: New ecosystem website page
lupesko commented on a change in pull request #11528: New ecosystem website page URL: https://github.com/apache/incubator-mxnet/pull/11528#discussion_r202897939 ## File path: docs/community/ecosystem.md ## @@ -0,0 +1,72 @@ +# MXNet's Ecosystem + +Community contributions to MXNet have added many new valuable features and functionality to support use cases such as model serving & portability, easy and flexible APIs, and educational material like crash courses and online books. This ecosystem page lists the projects that use MXNet, teach MXNet, or augment MXNet in some way. + + +## Highlighted Project + +[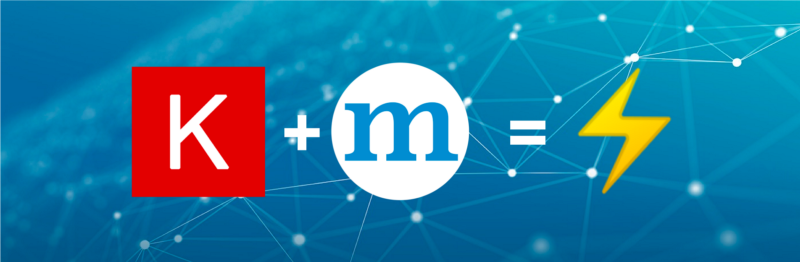](https://medium.com/apache-mxnet/announcing-keras-mxnet-v2-2-4b8404568e75) + [Keras-MXNet v2.2 released!](https://medium.com/apache-mxnet/announcing-keras-mxnet-v2-2-4b8404568e75) + + +## Contents + +* [Learning MXNet and other Educational Resources](#learning-mxnet-and-other-educational-resources) +* [MXNet APIs](#mxnet-apis) +* [Toolkits to Extend MXNet](#toolkits-to-extend-mxnet) +* [Debugging and Visualization](#debugging-and-visualization) +* [Model Serving](#model-serving) +* [Model Zoos](#model-zoos) +* [Contributions](#contributions) + + +## Learning MXNet and other Educational Resources + +* [Gluon 60 Minute Crash Course](https://gluon-crash-course.mxnet.io/) - deep learning practitioners can learn Gluon quickly with these six 10-minute tutorials. +- [YouTube Series](https://www.youtube.com/playlist?list=PLkEvNnRk8uVmVKRDgznk3o3LxmjFRaW7s) +* [The Straight Dope](https://gluon.mxnet.io/) - a series of notebooks designed to teach deep learning using the Gluon Python API for MXNet. + + +## MXNet APIs + +* [Clojure API](https://github.com/apache/incubator-mxnet/tree/master/contrib/clojure-package) - use MXNet with Clojure. +* [C++ API](../api/c++/index.html) - not be confused with the C++ backend, this API allows C++ programmers to train networks in C++. +* [Gluon Python Interface](../gluon/index.html) - train complex models imperatively and then deploy with a symbolic graph. +* [Julia API](../api/julia/index.html) *(Community Supported)* - train models with multiple GPUs using Julia. +* [Keras-MXNet](https://github.com/awslabs/keras-apache-mxnet) - design with Keras2 and train with MXNet as the backend for 2x or more speed improvement. +* [MinPy](https://github.com/dmlc/minpy) - Pure numpy practice with third party operator integration and MXNet as backend for GPU computing +* [Module Python API](../api/python/index.html) - backed by the Symbol API, you can define your network in a declarative fashion. +* [ONNX-MXnet API](../api/python/contrib/onnx.html) - train and use Open Neural Network eXchange (ONNX) model files. +* [Perl API](../api/perl/index.html) *(Community Supported)* - train models with multiple GPUs using Perl. +* [R API](https://mxnet.incubator.apache.org/api/r/index.html) *(Community Supported)* - train models with multiple GPUs using R. +* [Scala Infer API](../api/scala/infer.html) - model loading and inference functionality. +* [TensorFuse](https://github.com/dementrock/tensorfuse) - Common interface for Theano, CGT, TensorFlow, and MXNet (experimental) by [dementrock](https://github.com/dementrock) + + +## Toolkits to Extend MXNet + +* [NVIDIA DALI](https://github.com/NVIDIA/DALI/tree/master/examples/mxnet) - a collection of highly optimized building blocks and an execution engine to accelerate input data pre-processing for deep learning applications. Review comment: @aaronmarkham the link is broken; this one works: https://github.com/NVIDIA/DALI/blob/master/docs/examples/mxnet/gluon.ipynb @szha can you clarify why you would like to delete? seems like it may be a useful and performant toolkit for MXNet users. This is an automated message from the Apache Git Service. To respond to the message, please log on GitHub and use the URL above to go to the specific comment. For queries about this service, please contact Infrastructure at: us...@infra.apache.org With regards, Apache Git Services
[GitHub] lupesko commented on a change in pull request #11528: New ecosystem website page
lupesko commented on a change in pull request #11528: New ecosystem website page URL: https://github.com/apache/incubator-mxnet/pull/11528#discussion_r202897124 ## File path: docs/community/ecosystem.md ## @@ -0,0 +1,72 @@ +# MXNet's Ecosystem + +Community contributions to MXNet have added many new valuable features and functionality to support use cases such as model serving & portability, easy and flexible APIs, and educational material like crash courses and online books. This ecosystem page lists the projects that use MXNet, teach MXNet, or augment MXNet in some way. + + +## Highlighted Project + +[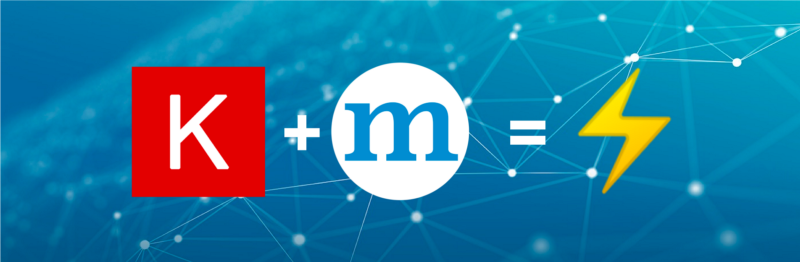](https://medium.com/apache-mxnet/announcing-keras-mxnet-v2-2-4b8404568e75) + [Keras-MXNet v2.2 released!](https://medium.com/apache-mxnet/announcing-keras-mxnet-v2-2-4b8404568e75) + + +## Contents + +* [Learning MXNet and other Educational Resources](#learning-mxnet-and-other-educational-resources) +* [MXNet APIs](#mxnet-apis) +* [Toolkits to Extend MXNet](#toolkits-to-extend-mxnet) +* [Debugging and Visualization](#debugging-and-visualization) +* [Model Serving](#model-serving) +* [Model Zoos](#model-zoos) +* [Contributions](#contributions) + + +## Learning MXNet and other Educational Resources + +* [Gluon 60 Minute Crash Course](https://gluon-crash-course.mxnet.io/) - deep learning practitioners can learn Gluon quickly with these six 10-minute tutorials. +- [YouTube Series](https://www.youtube.com/playlist?list=PLkEvNnRk8uVmVKRDgznk3o3LxmjFRaW7s) +* [The Straight Dope](https://gluon.mxnet.io/) - a series of notebooks designed to teach deep learning using the Gluon Python API for MXNet. + + +## MXNet APIs + +* [Clojure API](https://github.com/apache/incubator-mxnet/tree/master/contrib/clojure-package) - use MXNet with Clojure. +* [C++ API](../api/c++/index.html) - not be confused with the C++ backend, this API allows C++ programmers to train networks in C++. +* [Gluon Python Interface](../gluon/index.html) - train complex models imperatively and then deploy with a symbolic graph. +* [Julia API](../api/julia/index.html) *(Community Supported)* - train models with multiple GPUs using Julia. +* [Keras-MXNet](https://github.com/awslabs/keras-apache-mxnet) - design with Keras2 and train with MXNet as the backend for 2x or more speed improvement. +* [MinPy](https://github.com/dmlc/minpy) - Pure numpy practice with third party operator integration and MXNet as backend for GPU computing +* [Module Python API](../api/python/index.html) - backed by the Symbol API, you can define your network in a declarative fashion. +* [ONNX-MXnet API](../api/python/contrib/onnx.html) - train and use Open Neural Network eXchange (ONNX) model files. +* [Perl API](../api/perl/index.html) *(Community Supported)* - train models with multiple GPUs using Perl. +* [R API](https://mxnet.incubator.apache.org/api/r/index.html) *(Community Supported)* - train models with multiple GPUs using R. +* [Scala Infer API](../api/scala/infer.html) - model loading and inference functionality. +* [TensorFuse](https://github.com/dementrock/tensorfuse) - Common interface for Theano, CGT, TensorFlow, and MXNet (experimental) by [dementrock](https://github.com/dementrock) + + +## Toolkits to Extend MXNet + +* [NVIDIA DALI](https://github.com/NVIDIA/DALI/tree/master/examples/mxnet) - a collection of highly optimized building blocks and an execution engine to accelerate input data pre-processing for deep learning applications. +* [Gluon CV](https://gluon-cv.mxnet.io/) - state-of-the-art deep learning algorithms in computer vision. +* [Gluon NLP](https://gluon-nlp.mxnet.io/) - state-of-the-art deep learning models in natural language processing. +* [Sockeye](https://github.com/awslabs/sockeye) - a sequence-to-sequence framework for Neural Machine Translation + + +## Debugging and Visualization + +* [MXBoard](https://github.com/awslabs/mxboard) - lets you to visually inspect and interpret your MXNet runs and graphs using the TensorBoard software. + + +## Model Serving + +* [MXNet Model Server (MMS)](https://github.com/apache/incubator-mxnet/tree/master/example/model-server/mms.md) - simple yet scalable solution for model inference. + + +## Model Zoos + +* [Gluon Model Zoo](https://mxnet.incubator.apache.org/api/python/gluon/model_zoo.html) - models trained in Gluon and available through Gluon's model zoo API. +* [ONNX Model Zoo](https://github.com/onnx/model-zoo) - ONNX models from a variety of ONNX-supported frameworks. Review comment: Good catch @szha - @abhinavs95 recently moved ONNX model zoo into onnx/models. This is an automated message from the Apache Git Service. To respond to the message, please log on GitHub and use the URL above to go to the specific comment. For queries about this service, please contact Infrastructure at:
[GitHub] lupesko commented on issue #11775: NPE in BucketingModule when providedLabel of DataBatch is not set
lupesko commented on issue #11775: NPE in BucketingModule when providedLabel of DataBatch is not set URL: https://github.com/apache/incubator-mxnet/issues/11775#issuecomment-405458150 @nswamy please label as "Scala" "Bug" @lanking520 @andrewfayres @ddavydenko who are working on the Scala API This is an automated message from the Apache Git Service. To respond to the message, please log on GitHub and use the URL above to go to the specific comment. For queries about this service, please contact Infrastructure at: us...@infra.apache.org With regards, Apache Git Services
[GitHub] lupesko commented on issue #11775: NPE in BucketingModule when providedLabel of DataBatch is not set
lupesko commented on issue #11775: NPE in BucketingModule when providedLabel of DataBatch is not set URL: https://github.com/apache/incubator-mxnet/issues/11775#issuecomment-405457908 Thanks for reporting the issue @milandesai To help the community look into it, please provide more information as asked below (usually the new issue template asks to fill all of that in) - providing complete information in the most concise form is the best way to get help. - Package used (Python/R/Scala/Julia): - For Scala user, please provide: 1. Java version: (`java -version`) 2. Maven version: (`mvn -version`) 3. Scala runtime if applicable: (`scala -version`) - Build info (Required if built from source) 1. Compiler (gcc/clang/mingw/visual studio): 2. MXNet commit hash (Paste the output of `git rev-parse HEAD` here.) 3. Build config: (Paste the content of config.mk, or the build command.) - Minimum reproducible example (If you are using your own code, please provide a short script that reproduces the error. Otherwise, please provide link to the existing example.) Thanks! This is an automated message from the Apache Git Service. To respond to the message, please log on GitHub and use the URL above to go to the specific comment. For queries about this service, please contact Infrastructure at: us...@infra.apache.org With regards, Apache Git Services
[GitHub] pracheer commented on issue #11773: Update PyPI version number
pracheer commented on issue #11773: Update PyPI version number URL: https://github.com/apache/incubator-mxnet/pull/11773#issuecomment-405456204 Looks good to me! Thanks @apeforest This is an automated message from the Apache Git Service. To respond to the message, please log on GitHub and use the URL above to go to the specific comment. For queries about this service, please contact Infrastructure at: us...@infra.apache.org With regards, Apache Git Services
[GitHub] yzhliu commented on a change in pull request #11751: [MXNET-600][Scala] NDArray auto-collector
yzhliu commented on a change in pull request #11751: [MXNET-600][Scala] NDArray auto-collector URL: https://github.com/apache/incubator-mxnet/pull/11751#discussion_r202891346 ## File path: scala-package/core/src/main/scala/org/apache/mxnet/NDArrayCollector.scala ## @@ -0,0 +1,155 @@ +/* + * Licensed to the Apache Software Foundation (ASF) under one or more + * contributor license agreements. See the NOTICE file distributed with + * this work for additional information regarding copyright ownership. + * The ASF licenses this file to You under the Apache License, Version 2.0 + * (the "License"); you may not use this file except in compliance with + * the License. You may obtain a copy of the License at + * + *http://www.apache.org/licenses/LICENSE-2.0 + * + * Unless required by applicable law or agreed to in writing, software + * distributed under the License is distributed on an "AS IS" BASIS, + * WITHOUT WARRANTIES OR CONDITIONS OF ANY KIND, either express or implied. + * See the License for the specific language governing permissions and + * limitations under the License. + */ + +package org.apache.mxnet + +import org.slf4j.LoggerFactory + +import scala.annotation.varargs +import scala.collection.mutable + +/** + * A collector to store NDArrays. + * It provides a scope, NDArrays allocated in the scope can either + * - be disposed automatically when the code block finishes, or + * - simply be collected for future usage. + * + * If the return type of scope is NDArray or NDArrayFuncReturn, + * the collector is smart enough NOT to collect or dispose the returned NDArray. + * However in other cases, it is users' responsibility NOT to leak allocated NDArrays outside, + * (e.g., store to a global variable and use later, pass to another thread, etc.) + * Usage Example: + * + * val a = NDArray.array(Array(-1f, 0f, 1f, 2f, 3f, 4f), shape = Shape(2, 3)) + * val res = NDArrayCollector.auto().withScope { + *(NDArray.relu(a) + a).toArray + * } + * + * In the case above, the intermediate NDArrays + * (created by NDArray.relu and +) will be disposed automatically. + * User can also decide to dispose the collected NDArrays later: + * + * val collector = NDArrayCollector.manual() + * val res = collector.withScope { + *(NDArray.relu(a) + a).toArray + * } + * collector.foreach(_.dispose()) + * + * For Java users: + * + * NDArray a = NDArray.array(new float[]{-1f, 0f, 1f, 2f, 3f, 4f}, + *Shape.create(2, 3), Context.cpu(0)); + * float[] sliced = NDArrayCollector.auto().withScope( + *new scala.runtime.AbstractFunction0() { + *@Override + *public float[] apply() { + * a.slice(0, 1).toArray(); + *} + * }); + * + */ +object NDArrayCollector { + private val logger = LoggerFactory.getLogger(classOf[NDArrayCollector]) + + private val currCollector = new ThreadLocal[NDArrayCollector] { +override def initialValue = new NDArrayCollector(false, false) + } + + /** + * Create a collector which will dispose the collected NDArrays automatically. + * @return an auto-disposable collector. + */ + def auto(): NDArrayCollector = new NDArrayCollector(true) + + /** + * Create a collector allows users to later dispose the collected NDArray manually. + * @return a manually-disposable collector. + */ + def manual(): NDArrayCollector = new NDArrayCollector(false) + + /** + * Collect the NDArrays into the collector of the current thread. + * @param ndArray NDArrays need to be collected. + */ + @varargs def collect(ndArray: NDArray*): Unit = { +currCollector.get().add(ndArray: _*) + } +} + +class NDArrayCollector private(private val autoDispose: Boolean = true, + private val doCollect: Boolean = true) { + private val arrays = mutable.HashMap.empty[Long, NDArray] + + private def add(nd: NDArray*): Unit = { +if (doCollect) nd.foreach(arr => arrays.put(arr.handle, arr)) + } + + /** + * Clear the collector. + */ + def clear(): Unit = { +arrays.clear() + } + + /** + * Iterate over the collected NDArrays and apply the user-defined function to each NDArray. + * @param f the function that is applied for its side-effect to every NDArray. + * The result of function f is discarded. + */ + def foreach(f: NDArray => Unit): Unit = { +arrays.values.foreach(f(_)) + } + + /** + * @return how many unique NDArrays are collected. + */ + def size: Int = arrays.size + + /** + * Create a code scope, NDArrays allocated within this scope will be collected. + * The collected NDArrays will be either + * - disposed automatically when the code blcok finishes (when using auto) or + * - stored for later access (when using manual) + * If the return type of scope is NDArray or NDArrayFuncReturn, + * it is smart enough NOT to collect or dispose the returned NDArray. + * However in other cases, it is users'
[GitHub] yzhliu commented on a change in pull request #11751: [MXNET-600][Scala] NDArray auto-collector
yzhliu commented on a change in pull request #11751: [MXNET-600][Scala] NDArray auto-collector URL: https://github.com/apache/incubator-mxnet/pull/11751#discussion_r202891252 ## File path: scala-package/core/src/main/scala/org/apache/mxnet/NDArrayCollector.scala ## @@ -0,0 +1,155 @@ +/* + * Licensed to the Apache Software Foundation (ASF) under one or more + * contributor license agreements. See the NOTICE file distributed with + * this work for additional information regarding copyright ownership. + * The ASF licenses this file to You under the Apache License, Version 2.0 + * (the "License"); you may not use this file except in compliance with + * the License. You may obtain a copy of the License at + * + *http://www.apache.org/licenses/LICENSE-2.0 + * + * Unless required by applicable law or agreed to in writing, software + * distributed under the License is distributed on an "AS IS" BASIS, + * WITHOUT WARRANTIES OR CONDITIONS OF ANY KIND, either express or implied. + * See the License for the specific language governing permissions and + * limitations under the License. + */ + +package org.apache.mxnet + +import org.slf4j.LoggerFactory + +import scala.annotation.varargs +import scala.collection.mutable + +/** + * A collector to store NDArrays. + * It provides a scope, NDArrays allocated in the scope can either + * - be disposed automatically when the code block finishes, or + * - simply be collected for future usage. + * + * If the return type of scope is NDArray or NDArrayFuncReturn, + * the collector is smart enough NOT to collect or dispose the returned NDArray. + * However in other cases, it is users' responsibility NOT to leak allocated NDArrays outside, + * (e.g., store to a global variable and use later, pass to another thread, etc.) + * Usage Example: + * + * val a = NDArray.array(Array(-1f, 0f, 1f, 2f, 3f, 4f), shape = Shape(2, 3)) + * val res = NDArrayCollector.auto().withScope { + *(NDArray.relu(a) + a).toArray + * } + * + * In the case above, the intermediate NDArrays + * (created by NDArray.relu and +) will be disposed automatically. + * User can also decide to dispose the collected NDArrays later: + * + * val collector = NDArrayCollector.manual() + * val res = collector.withScope { + *(NDArray.relu(a) + a).toArray + * } + * collector.foreach(_.dispose()) + * + * For Java users: + * + * NDArray a = NDArray.array(new float[]{-1f, 0f, 1f, 2f, 3f, 4f}, + *Shape.create(2, 3), Context.cpu(0)); + * float[] sliced = NDArrayCollector.auto().withScope( + *new scala.runtime.AbstractFunction0() { + *@Override + *public float[] apply() { + * a.slice(0, 1).toArray(); + *} + * }); + * + */ +object NDArrayCollector { + private val logger = LoggerFactory.getLogger(classOf[NDArrayCollector]) + + private val currCollector = new ThreadLocal[NDArrayCollector] { +override def initialValue = new NDArrayCollector(false, false) + } + + /** + * Create a collector which will dispose the collected NDArrays automatically. + * @return an auto-disposable collector. + */ + def auto(): NDArrayCollector = new NDArrayCollector(true) + + /** + * Create a collector allows users to later dispose the collected NDArray manually. + * @return a manually-disposable collector. + */ + def manual(): NDArrayCollector = new NDArrayCollector(false) + + /** + * Collect the NDArrays into the collector of the current thread. + * @param ndArray NDArrays need to be collected. + */ + @varargs def collect(ndArray: NDArray*): Unit = { +currCollector.get().add(ndArray: _*) + } +} + +class NDArrayCollector private(private val autoDispose: Boolean = true, + private val doCollect: Boolean = true) { + private val arrays = mutable.HashMap.empty[Long, NDArray] + + private def add(nd: NDArray*): Unit = { +if (doCollect) nd.foreach(arr => arrays.put(arr.handle, arr)) + } + + /** + * Clear the collector. + */ + def clear(): Unit = { Review comment: When using manual(), user may want to re-use one collector: ```scala val c = NDArrayCollector.manual() c.withScope { ... } ... c.clear() c.withScope { ... } ``` This is an automated message from the Apache Git Service. To respond to the message, please log on GitHub and use the URL above to go to the specific comment. For queries about this service, please contact Infrastructure at: us...@infra.apache.org With regards, Apache Git Services
[GitHub] yzhliu commented on a change in pull request #11751: [MXNET-600][Scala] NDArray auto-collector
yzhliu commented on a change in pull request #11751: [MXNET-600][Scala] NDArray auto-collector URL: https://github.com/apache/incubator-mxnet/pull/11751#discussion_r202891007 ## File path: scala-package/core/src/main/scala/org/apache/mxnet/NDArrayCollector.scala ## @@ -0,0 +1,155 @@ +/* + * Licensed to the Apache Software Foundation (ASF) under one or more + * contributor license agreements. See the NOTICE file distributed with + * this work for additional information regarding copyright ownership. + * The ASF licenses this file to You under the Apache License, Version 2.0 + * (the "License"); you may not use this file except in compliance with + * the License. You may obtain a copy of the License at + * + *http://www.apache.org/licenses/LICENSE-2.0 + * + * Unless required by applicable law or agreed to in writing, software + * distributed under the License is distributed on an "AS IS" BASIS, + * WITHOUT WARRANTIES OR CONDITIONS OF ANY KIND, either express or implied. + * See the License for the specific language governing permissions and + * limitations under the License. + */ + +package org.apache.mxnet + +import org.slf4j.LoggerFactory + +import scala.annotation.varargs +import scala.collection.mutable + +/** + * A collector to store NDArrays. + * It provides a scope, NDArrays allocated in the scope can either + * - be disposed automatically when the code block finishes, or + * - simply be collected for future usage. + * + * If the return type of scope is NDArray or NDArrayFuncReturn, + * the collector is smart enough NOT to collect or dispose the returned NDArray. + * However in other cases, it is users' responsibility NOT to leak allocated NDArrays outside, + * (e.g., store to a global variable and use later, pass to another thread, etc.) + * Usage Example: + * + * val a = NDArray.array(Array(-1f, 0f, 1f, 2f, 3f, 4f), shape = Shape(2, 3)) + * val res = NDArrayCollector.auto().withScope { + *(NDArray.relu(a) + a).toArray + * } + * + * In the case above, the intermediate NDArrays + * (created by NDArray.relu and +) will be disposed automatically. + * User can also decide to dispose the collected NDArrays later: + * + * val collector = NDArrayCollector.manual() + * val res = collector.withScope { + *(NDArray.relu(a) + a).toArray + * } + * collector.foreach(_.dispose()) + * + * For Java users: + * + * NDArray a = NDArray.array(new float[]{-1f, 0f, 1f, 2f, 3f, 4f}, + *Shape.create(2, 3), Context.cpu(0)); + * float[] sliced = NDArrayCollector.auto().withScope( + *new scala.runtime.AbstractFunction0() { + *@Override + *public float[] apply() { + * a.slice(0, 1).toArray(); + *} + * }); + * + */ +object NDArrayCollector { + private val logger = LoggerFactory.getLogger(classOf[NDArrayCollector]) + + private val currCollector = new ThreadLocal[NDArrayCollector] { +override def initialValue = new NDArrayCollector(false, false) + } + + /** + * Create a collector which will dispose the collected NDArrays automatically. + * @return an auto-disposable collector. + */ + def auto(): NDArrayCollector = new NDArrayCollector(true) + + /** + * Create a collector allows users to later dispose the collected NDArray manually. + * @return a manually-disposable collector. + */ + def manual(): NDArrayCollector = new NDArrayCollector(false) + + /** + * Collect the NDArrays into the collector of the current thread. + * @param ndArray NDArrays need to be collected. + */ + @varargs def collect(ndArray: NDArray*): Unit = { +currCollector.get().add(ndArray: _*) + } +} + +class NDArrayCollector private(private val autoDispose: Boolean = true, + private val doCollect: Boolean = true) { + private val arrays = mutable.HashMap.empty[Long, NDArray] Review comment: this is exactly what I intended to avoid. Two NDArrays with same ptr must be disposed only once. This is an automated message from the Apache Git Service. To respond to the message, please log on GitHub and use the URL above to go to the specific comment. For queries about this service, please contact Infrastructure at: us...@infra.apache.org With regards, Apache Git Services
[GitHub] ZhennanQin commented on issue #10724: Flaky test MKLDNN test_bucket_module
ZhennanQin commented on issue #10724: Flaky test MKLDNN test_bucket_module URL: https://github.com/apache/incubator-mxnet/issues/10724#issuecomment-405451800 `for i in {1..1000}; do python tests/python/train/test_bucketing.py; done` In this way, I still can't reproduce this bug. As in first way you can't reproduce either, I assume the original bug has been fixed. For the segfault you saw in second way, maybe it's another issue. This is an automated message from the Apache Git Service. To respond to the message, please log on GitHub and use the URL above to go to the specific comment. For queries about this service, please contact Infrastructure at: us...@infra.apache.org With regards, Apache Git Services
[GitHub] szha opened a new pull request #11776: fix rnn layer save
szha opened a new pull request #11776: fix rnn layer save URL: https://github.com/apache/incubator-mxnet/pull/11776 ## Description ## Resolves #11772 ## Checklist ## ### Essentials ### Please feel free to remove inapplicable items for your PR. - [x] Changes are complete (i.e. I finished coding on this PR) - [x] All changes have test coverage: - Unit tests are added for small changes to verify correctness (e.g. adding a new operator) - [x] To the my best knowledge, examples are either not affected by this change, or have been fixed to be compatible with this change ### Changes ### - [x] Fix RNN layer save This is an automated message from the Apache Git Service. To respond to the message, please log on GitHub and use the URL above to go to the specific comment. For queries about this service, please contact Infrastructure at: us...@infra.apache.org With regards, Apache Git Services
[GitHub] apeforest commented on a change in pull request #11773: Update PyPI version number
apeforest commented on a change in pull request #11773: Update PyPI version number URL: https://github.com/apache/incubator-mxnet/pull/11773#discussion_r202886104 ## File path: tools/coreml/pip_package/setup.py ## @@ -40,7 +40,7 @@ def readme(): return f.read() setup(name='mxnet-to-coreml', - version='0.1.0', + version='0.1.1', Review comment: Updated This is an automated message from the Apache Git Service. To respond to the message, please log on GitHub and use the URL above to go to the specific comment. For queries about this service, please contact Infrastructure at: us...@infra.apache.org With regards, Apache Git Services
[GitHub] lanking520 edited a comment on issue #11681: [MXNET-531] improvement of adding source.jar to assembly
lanking520 edited a comment on issue #11681: [MXNET-531] improvement of adding source.jar to assembly URL: https://github.com/apache/incubator-mxnet/pull/11681#issuecomment-405448665 @gigasquid I tried to reproduce the issue in CI but failed on my Ubuntu Machine ``` lein test + lein test Could not find artifact org.apache.mxnet:mxnet-full_2.11-linux-x86_64-cpu:jar:1.3.0-SNAPSHOT in clojars (https://repo.clojars.org/) Could not find artifact org.apache.mxnet:mxnet-full_2.11-linux-x86_64-cpu:jar:1.3.0-SNAPSHOT in staging (https://repository.apache.org/content/repositories/staging) This could be due to a typo in :dependencies, file system permissions, or network issues. If you are behind a proxy, try setting the 'http_proxy' environment variable. ``` Could you please help with the `Clojure` package build failure, I have `.so` generated here: ``` /home/ubuntu/incubator-mxnet/scala-package/native/linux-x86_64-cpu/target/libmxnet-scala-linux-x86_64-cpu.so ``` `-Djava.library.path=[lib path]` Step to reproduce `Ubuntu 16.04`: ``` sudo bash ./ubuntu_core.sh sudo .bash /ubuntu_scala.sh make -j $(nprocs) make scalapkg make scalainstall sudo bash ubuntu_clojure.sh lein test ``` This is an automated message from the Apache Git Service. To respond to the message, please log on GitHub and use the URL above to go to the specific comment. For queries about this service, please contact Infrastructure at: us...@infra.apache.org With regards, Apache Git Services
[GitHub] lanking520 commented on issue #11681: [MXNET-531] improvement of adding source.jar to assembly
lanking520 commented on issue #11681: [MXNET-531] improvement of adding source.jar to assembly URL: https://github.com/apache/incubator-mxnet/pull/11681#issuecomment-405448665 @gigasquid I tried to reproduce the issue in CI but failed on my Ubuntu Machine ``` lein test + lein test Could not find artifact org.apache.mxnet:mxnet-full_2.11-linux-x86_64-cpu:jar:1.3.0-SNAPSHOT in clojars (https://repo.clojars.org/) Could not find artifact org.apache.mxnet:mxnet-full_2.11-linux-x86_64-cpu:jar:1.3.0-SNAPSHOT in staging (https://repository.apache.org/content/repositories/staging) This could be due to a typo in :dependencies, file system permissions, or network issues. If you are behind a proxy, try setting the 'http_proxy' environment variable. ``` Could you please help with the `Clojure` package build failure, I have `.so` generated here: ``` /home/ubuntu/incubator-mxnet/scala-package/native/linux-x86_64-cpu/target/libmxnet-scala-linux-x86_64-cpu.so ``` `-Djava.library.path=[lib path]` This is an automated message from the Apache Git Service. To respond to the message, please log on GitHub and use the URL above to go to the specific comment. For queries about this service, please contact Infrastructure at: us...@infra.apache.org With regards, Apache Git Services
[GitHub] safrooze commented on issue #11772: load_parameters expecting parameters that don't exist
safrooze commented on issue #11772: load_parameters expecting parameters that don't exist URL: https://github.com/apache/incubator-mxnet/issues/11772#issuecomment-405446821 Looks like the change in #10989 introduces this regression. This change is not included in 1.2.0 or 1.2.1. This is an automated message from the Apache Git Service. To respond to the message, please log on GitHub and use the URL above to go to the specific comment. For queries about this service, please contact Infrastructure at: us...@infra.apache.org With regards, Apache Git Services
[GitHub] safrooze commented on issue #10989: add gluon model summary
safrooze commented on issue #10989: add gluon model summary URL: https://github.com/apache/incubator-mxnet/pull/10989#issuecomment-405446404 @szha I think this change causes a regression reported in [issue #11772](https://github.com/apache/incubator-mxnet/issues/11772). This is an automated message from the Apache Git Service. To respond to the message, please log on GitHub and use the URL above to go to the specific comment. For queries about this service, please contact Infrastructure at: us...@infra.apache.org With regards, Apache Git Services
[GitHub] frankfliu commented on a change in pull request #11749: [MXNET-8230] test_operator_gpu.test_rms fails
frankfliu commented on a change in pull request #11749: [MXNET-8230] test_operator_gpu.test_rms fails URL: https://github.com/apache/incubator-mxnet/pull/11749#discussion_r202879363 ## File path: tests/python/unittest/test_optimizer.py ## @@ -848,6 +848,13 @@ def test_rms(): wd_options = [{}, {'wd': 0.03}, {'wd': 0.05}, {'wd': 0.07}] mp_options = [{}, {'multi_precision': False}, {'multi_precision': True}] for dtype in [np.float16, np.float32]: +if (dtype == np.float16): +rtol=1e-1 +atol=1e-1 +else: +rtol=1e-2 +atol=1e-2 Review comment: done This is an automated message from the Apache Git Service. To respond to the message, please log on GitHub and use the URL above to go to the specific comment. For queries about this service, please contact Infrastructure at: us...@infra.apache.org With regards, Apache Git Services
[GitHub] frankfliu commented on a change in pull request #11749: [MXNET-8230] test_operator_gpu.test_rms fails
frankfliu commented on a change in pull request #11749: [MXNET-8230] test_operator_gpu.test_rms fails URL: https://github.com/apache/incubator-mxnet/pull/11749#discussion_r202879342 ## File path: tests/python/unittest/test_optimizer.py ## @@ -835,8 +835,8 @@ def update(self, index, weight, grad, state): if self.clip_weights: mx.ndarray.clip(weight, -self.clip_weights, self.clip_weights, out=weight) -@unittest.skip("Test fails intermittently. Temporarily disabled until fixed. Tracked at https://github.com/apache/incubator-mxnet/issues/8230;) -@with_seed(0) +# @unittest.skip("Test fails intermittently. Temporarily disabled until fixed. Tracked at https://github.com/apache/incubator-mxnet/issues/8230;) Review comment: done This is an automated message from the Apache Git Service. To respond to the message, please log on GitHub and use the URL above to go to the specific comment. For queries about this service, please contact Infrastructure at: us...@infra.apache.org With regards, Apache Git Services
[GitHub] hetong007 commented on a change in pull request #11374: [MXNET-563] Refactor R optimizers to fix memory leak
hetong007 commented on a change in pull request #11374: [MXNET-563] Refactor R optimizers to fix memory leak URL: https://github.com/apache/incubator-mxnet/pull/11374#discussion_r202875960 ## File path: R-package/R/optimizer.R ## @@ -159,41 +209,60 @@ mx.opt.rmsprop <- function(learning.rate=0.002, #' L2 regularization coefficient add to all the weights. #' @param rescale.grad float, default=1.0 #' rescaling factor of gradient. -#' @param clip_gradient float, optional +#' @param clip_gradient float, optional, default=-1 #' clip gradient in range [-clip_gradient, clip_gradient]. #' @param lr_scheduler function, optional #' The learning rate scheduler. #' -mx.opt.adam <- function(learning.rate=0.001, -beta1=0.9, -beta2=0.999, -epsilon=1e-8, -wd=0, -rescale.grad=1, -clip_gradient = NULL, +mx.opt.adam <- function(learning.rate = 1e-3, +beta1 = 0.9, +beta2 = 0.999, +epsilon = 1e-8, +wd = 0, +rescale.grad = 1, +clip_gradient = -1, Review comment: Please add that to the docstring, otherwise people may feel confused without looking at the code. This is an automated message from the Apache Git Service. To respond to the message, please log on GitHub and use the URL above to go to the specific comment. For queries about this service, please contact Infrastructure at: us...@infra.apache.org With regards, Apache Git Services
[GitHub] szha commented on a change in pull request #11528: New ecosystem website page
szha commented on a change in pull request #11528: New ecosystem website page URL: https://github.com/apache/incubator-mxnet/pull/11528#discussion_r202875781 ## File path: docs/community/ecosystem.md ## @@ -0,0 +1,72 @@ +# MXNet's Ecosystem + +Community contributions to MXNet have added many new valuable features and functionality to support use cases such as model serving & portability, easy and flexible APIs, and educational material like crash courses and online books. This ecosystem page lists the projects that use MXNet, teach MXNet, or augment MXNet in some way. + + +## Highlighted Project + +[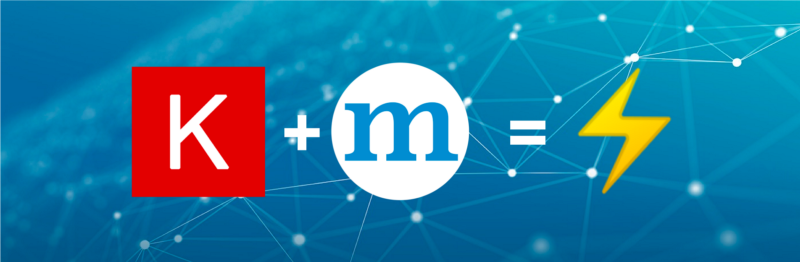](https://medium.com/apache-mxnet/announcing-keras-mxnet-v2-2-4b8404568e75) + [Keras-MXNet v2.2 released!](https://medium.com/apache-mxnet/announcing-keras-mxnet-v2-2-4b8404568e75) + + +## Contents + +* [Learning MXNet and other Educational Resources](#learning-mxnet-and-other-educational-resources) +* [MXNet APIs](#mxnet-apis) +* [Toolkits to Extend MXNet](#toolkits-to-extend-mxnet) +* [Debugging and Visualization](#debugging-and-visualization) +* [Model Serving](#model-serving) +* [Model Zoos](#model-zoos) +* [Contributions](#contributions) + + +## Learning MXNet and other Educational Resources + +* [Gluon 60 Minute Crash Course](https://gluon-crash-course.mxnet.io/) - deep learning practitioners can learn Gluon quickly with these six 10-minute tutorials. +- [YouTube Series](https://www.youtube.com/playlist?list=PLkEvNnRk8uVmVKRDgznk3o3LxmjFRaW7s) +* [The Straight Dope](https://gluon.mxnet.io/) - a series of notebooks designed to teach deep learning using the Gluon Python API for MXNet. + + +## MXNet APIs + +* [Clojure API](https://github.com/apache/incubator-mxnet/tree/master/contrib/clojure-package) - use MXNet with Clojure. +* [C++ API](../api/c++/index.html) - not be confused with the C++ backend, this API allows C++ programmers to train networks in C++. +* [Gluon Python Interface](../gluon/index.html) - train complex models imperatively and then deploy with a symbolic graph. +* [Julia API](../api/julia/index.html) *(Community Supported)* - train models with multiple GPUs using Julia. +* [Keras-MXNet](https://github.com/awslabs/keras-apache-mxnet) - design with Keras2 and train with MXNet as the backend for 2x or more speed improvement. +* [MinPy](https://github.com/dmlc/minpy) - Pure numpy practice with third party operator integration and MXNet as backend for GPU computing +* [Module Python API](../api/python/index.html) - backed by the Symbol API, you can define your network in a declarative fashion. +* [ONNX-MXnet API](../api/python/contrib/onnx.html) - train and use Open Neural Network eXchange (ONNX) model files. +* [Perl API](../api/perl/index.html) *(Community Supported)* - train models with multiple GPUs using Perl. +* [R API](https://mxnet.incubator.apache.org/api/r/index.html) *(Community Supported)* - train models with multiple GPUs using R. +* [Scala Infer API](../api/scala/infer.html) - model loading and inference functionality. +* [TensorFuse](https://github.com/dementrock/tensorfuse) - Common interface for Theano, CGT, TensorFlow, and MXNet (experimental) by [dementrock](https://github.com/dementrock) + + +## Toolkits to Extend MXNet + +* [NVIDIA DALI](https://github.com/NVIDIA/DALI/tree/master/examples/mxnet) - a collection of highly optimized building blocks and an execution engine to accelerate input data pre-processing for deep learning applications. +* [Gluon CV](https://gluon-cv.mxnet.io/) - state-of-the-art deep learning algorithms in computer vision. +* [Gluon NLP](https://gluon-nlp.mxnet.io/) - state-of-the-art deep learning models in natural language processing. +* [Sockeye](https://github.com/awslabs/sockeye) - a sequence-to-sequence framework for Neural Machine Translation + + +## Debugging and Visualization + +* [MXBoard](https://github.com/awslabs/mxboard) - lets you to visually inspect and interpret your MXNet runs and graphs using the TensorBoard software. + + +## Model Serving + +* [MXNet Model Server (MMS)](https://github.com/apache/incubator-mxnet/tree/master/example/model-server/mms.md) - simple yet scalable solution for model inference. + + +## Model Zoos + +* [Gluon Model Zoo](https://mxnet.incubator.apache.org/api/python/gluon/model_zoo.html) - models trained in Gluon and available through Gluon's model zoo API. +* [ONNX Model Zoo](https://github.com/onnx/model-zoo) - ONNX models from a variety of ONNX-supported frameworks. Review comment: This link redirects to a personal repo and doesn't seem to be the official model zoo. The one in onnx org is https://github.com/onnx/models This is an automated message from the Apache Git Service. To respond to the message, please log on GitHub and use the URL above to go to the specific comment. For
[GitHub] jeremiedb commented on a change in pull request #11374: [MXNET-563] Refactor R optimizers to fix memory leak
jeremiedb commented on a change in pull request #11374: [MXNET-563] Refactor R optimizers to fix memory leak URL: https://github.com/apache/incubator-mxnet/pull/11374#discussion_r202873909 ## File path: R-package/R/optimizer.R ## @@ -159,41 +209,60 @@ mx.opt.rmsprop <- function(learning.rate=0.002, #' L2 regularization coefficient add to all the weights. #' @param rescale.grad float, default=1.0 #' rescaling factor of gradient. -#' @param clip_gradient float, optional +#' @param clip_gradient float, optional, default=-1 #' clip gradient in range [-clip_gradient, clip_gradient]. #' @param lr_scheduler function, optional #' The learning rate scheduler. #' -mx.opt.adam <- function(learning.rate=0.001, -beta1=0.9, -beta2=0.999, -epsilon=1e-8, -wd=0, -rescale.grad=1, -clip_gradient = NULL, +mx.opt.adam <- function(learning.rate = 1e-3, +beta1 = 0.9, +beta2 = 0.999, +epsilon = 1e-8, +wd = 0, +rescale.grad = 1, +clip_gradient = -1, Review comment: When `clip_gradient` is < 0, no clipping is applied. This is an automated message from the Apache Git Service. To respond to the message, please log on GitHub and use the URL above to go to the specific comment. For queries about this service, please contact Infrastructure at: us...@infra.apache.org With regards, Apache Git Services
[GitHub] szha commented on a change in pull request #11528: New ecosystem website page
szha commented on a change in pull request #11528: New ecosystem website page URL: https://github.com/apache/incubator-mxnet/pull/11528#discussion_r202873424 ## File path: docs/community/ecosystem.md ## @@ -0,0 +1,72 @@ +# MXNet's Ecosystem + +Community contributions to MXNet have added many new valuable features and functionality to support use cases such as model serving & portability, easy and flexible APIs, and educational material like crash courses and online books. This ecosystem page lists the projects that use MXNet, teach MXNet, or augment MXNet in some way. + + +## Highlighted Project + +[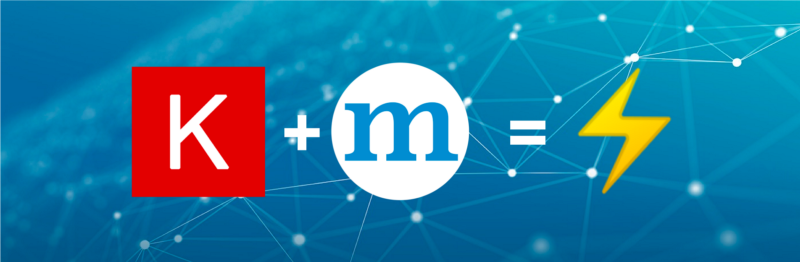](https://medium.com/apache-mxnet/announcing-keras-mxnet-v2-2-4b8404568e75) + [Keras-MXNet v2.2 released!](https://medium.com/apache-mxnet/announcing-keras-mxnet-v2-2-4b8404568e75) + + +## Contents + +* [Learning MXNet and other Educational Resources](#learning-mxnet-and-other-educational-resources) +* [MXNet APIs](#mxnet-apis) +* [Toolkits to Extend MXNet](#toolkits-to-extend-mxnet) +* [Debugging and Visualization](#debugging-and-visualization) +* [Model Serving](#model-serving) +* [Model Zoos](#model-zoos) +* [Contributions](#contributions) + + +## Learning MXNet and other Educational Resources + +* [Gluon 60 Minute Crash Course](https://gluon-crash-course.mxnet.io/) - deep learning practitioners can learn Gluon quickly with these six 10-minute tutorials. +- [YouTube Series](https://www.youtube.com/playlist?list=PLkEvNnRk8uVmVKRDgznk3o3LxmjFRaW7s) +* [The Straight Dope](https://gluon.mxnet.io/) - a series of notebooks designed to teach deep learning using the Gluon Python API for MXNet. + + +## MXNet APIs + +* [Clojure API](https://github.com/apache/incubator-mxnet/tree/master/contrib/clojure-package) - use MXNet with Clojure. +* [C++ API](../api/c++/index.html) - not be confused with the C++ backend, this API allows C++ programmers to train networks in C++. +* [Gluon Python Interface](../gluon/index.html) - train complex models imperatively and then deploy with a symbolic graph. +* [Julia API](../api/julia/index.html) *(Community Supported)* - train models with multiple GPUs using Julia. +* [Keras-MXNet](https://github.com/awslabs/keras-apache-mxnet) - design with Keras2 and train with MXNet as the backend for 2x or more speed improvement. +* [MinPy](https://github.com/dmlc/minpy) - Pure numpy practice with third party operator integration and MXNet as backend for GPU computing +* [Module Python API](../api/python/index.html) - backed by the Symbol API, you can define your network in a declarative fashion. +* [ONNX-MXnet API](../api/python/contrib/onnx.html) - train and use Open Neural Network eXchange (ONNX) model files. +* [Perl API](../api/perl/index.html) *(Community Supported)* - train models with multiple GPUs using Perl. +* [R API](https://mxnet.incubator.apache.org/api/r/index.html) *(Community Supported)* - train models with multiple GPUs using R. +* [Scala Infer API](../api/scala/infer.html) - model loading and inference functionality. +* [TensorFuse](https://github.com/dementrock/tensorfuse) - Common interface for Theano, CGT, TensorFlow, and MXNet (experimental) by [dementrock](https://github.com/dementrock) + + +## Toolkits to Extend MXNet + +* [NVIDIA DALI](https://github.com/NVIDIA/DALI/tree/master/examples/mxnet) - a collection of highly optimized building blocks and an execution engine to accelerate input data pre-processing for deep learning applications. Review comment: delete This is an automated message from the Apache Git Service. To respond to the message, please log on GitHub and use the URL above to go to the specific comment. For queries about this service, please contact Infrastructure at: us...@infra.apache.org With regards, Apache Git Services
[GitHub] szha commented on a change in pull request #11528: New ecosystem website page
szha commented on a change in pull request #11528: New ecosystem website page URL: https://github.com/apache/incubator-mxnet/pull/11528#discussion_r202873847 ## File path: example/model-server/mms.md ## @@ -0,0 +1,115 @@ +# Model Server for Apache MXNet (incubating) Review comment: Delete. This is MMS code from AWSLabs, and its documentation shouldn't reside in MXNet. This is an automated message from the Apache Git Service. To respond to the message, please log on GitHub and use the URL above to go to the specific comment. For queries about this service, please contact Infrastructure at: us...@infra.apache.org With regards, Apache Git Services
[GitHub] anirudh2290 commented on issue #11772: load_parameters expecting parameters that don't exist
anirudh2290 commented on issue #11772: load_parameters expecting parameters that don't exist URL: https://github.com/apache/incubator-mxnet/issues/11772#issuecomment-405429230 tested that on 1.2.1 this works fine. This is an automated message from the Apache Git Service. To respond to the message, please log on GitHub and use the URL above to go to the specific comment. For queries about this service, please contact Infrastructure at: us...@infra.apache.org With regards, Apache Git Services
[GitHub] milandesai opened a new issue #11775: NPE in BucketingModule when providedLabel of DataBatch is not set
milandesai opened a new issue #11775: NPE in BucketingModule when providedLabel of DataBatch is not set URL: https://github.com/apache/incubator-mxnet/issues/11775 We create a DataBatch without setting the `providedLabel` field. We then call `BucketingModule.forward(...)` with the data batch and get a NullPointerException: ``` java.lang.NullPointerException was thrown. java.lang.NullPointerException at ml.dmlc.mxnet.DataDesc$.ListMap2Descs(IO.scala:252) at ml.dmlc.mxnet.module.BucketingModule.forward(BucketingModule.scala:313) ``` This happens because the implicit method `ListMap2Descs` tries to convert `ListMap[String, Shape]` into `IndexedSeq[DataDesc]` but fails because the former is null by default. This is an automated message from the Apache Git Service. To respond to the message, please log on GitHub and use the URL above to go to the specific comment. For queries about this service, please contact Infrastructure at: us...@infra.apache.org With regards, Apache Git Services
[incubator-mxnet-site] branch asf-site updated: Bump the publish timestamp.
This is an automated email from the ASF dual-hosted git repository. zhasheng pushed a commit to branch asf-site in repository https://gitbox.apache.org/repos/asf/incubator-mxnet-site.git The following commit(s) were added to refs/heads/asf-site by this push: new 0ed87b0 Bump the publish timestamp. 0ed87b0 is described below commit 0ed87b0da25768f779c0eedce1f75ae236b0f4e4 Author: mxnet-ci AuthorDate: Tue Jul 17 00:46:39 2018 + Bump the publish timestamp. --- date.txt | 1 + 1 file changed, 1 insertion(+) diff --git a/date.txt b/date.txt new file mode 100644 index 000..4f52c26 --- /dev/null +++ b/date.txt @@ -0,0 +1 @@ +Tue Jul 17 00:46:39 UTC 2018
[GitHub] pengzhao-intel commented on issue #11756: [MXNET-677] int8 quantization does not work on toy mnist dataset
pengzhao-intel commented on issue #11756: [MXNET-677] int8 quantization does not work on toy mnist dataset URL: https://github.com/apache/incubator-mxnet/issues/11756#issuecomment-405422167 @OneRaynyDay we have successfully run MNIST in our local with the quantization flow. @xinyu-intel will help to take a look for your scripts. This is an automated message from the Apache Git Service. To respond to the message, please log on GitHub and use the URL above to go to the specific comment. For queries about this service, please contact Infrastructure at: us...@infra.apache.org With regards, Apache Git Services
[GitHub] lanking520 commented on issue #10753: Scala Module MNIST MLP example not working
lanking520 commented on issue #10753: Scala Module MNIST MLP example not working URL: https://github.com/apache/incubator-mxnet/issues/10753#issuecomment-405419911 Hi @gigasquid , I am also facing the same issues when I try to deal with RNN example, I have add some fix here: https://github.com/apache/incubator-mxnet/pull/11753. Please see if this helps this problem as well This is an automated message from the Apache Git Service. To respond to the message, please log on GitHub and use the URL above to go to the specific comment. For queries about this service, please contact Infrastructure at: us...@infra.apache.org With regards, Apache Git Services
[GitHub] OneRaynyDay commented on issue #11756: [MXNET-677] int8 quantization does not work on toy mnist dataset
OneRaynyDay commented on issue #11756: [MXNET-677] int8 quantization does not work on toy mnist dataset URL: https://github.com/apache/incubator-mxnet/issues/11756#issuecomment-405416065 Thank you. I have also found out the reason for the failure, and it is not related to CPU quantization. We should discuss more about this in the slack channel. It is a very strange issue. This is an automated message from the Apache Git Service. To respond to the message, please log on GitHub and use the URL above to go to the specific comment. For queries about this service, please contact Infrastructure at: us...@infra.apache.org With regards, Apache Git Services
[GitHub] roywei commented on issue #11774: Gluon BatchNorm beta=False not working properly
roywei commented on issue #11774: Gluon BatchNorm beta=False not working properly URL: https://github.com/apache/incubator-mxnet/issues/11774#issuecomment-405414431 @sandeep-krishnamurthy could you help label Gluon, Bug? Thanks This is an automated message from the Apache Git Service. To respond to the message, please log on GitHub and use the URL above to go to the specific comment. For queries about this service, please contact Infrastructure at: us...@infra.apache.org With regards, Apache Git Services
[GitHub] roywei opened a new issue #11774: Gluon BatchNorm beta=False not working properly
roywei opened a new issue #11774: Gluon BatchNorm beta=False not working properly URL: https://github.com/apache/incubator-mxnet/issues/11774 **Description** I came from #10401 In that issue, there is no reproducible code, so not sure whats the use case there. When I was developing mxnet backend for keras, I have similar issue. So I used this [unit test](https://github.com/keras-team/keras/blob/6489c457d03e5fd5d605ed6197932f3b769ff951/tests/keras/layers/normalization_test.py#L144) and was able to reproduce: **Config** machine: mac mxnet: latest master (pip installed mxnet --pre) keras: 2.2.0 with TensorFlow backend **The purpose of the test:** Create a random normalized data with size size=(1000, 3, 4, 4), centered on 5.0, variance 10.0. Use batchnorm layer to normalize it to have mean close to 0, and std close to 1. **Reproducible code:** First part of the code shows Keras implementation with TensorFlow backend, you can see mean is close to 0 and std is close to 1. Second Part of the code shows Gluon implementation, When scale and center is False, it throws error ``` import mxnet as mx import numpy as np from keras.layers import normalization from keras.models import Sequential from mxnet import autograd from mxnet import gluon from mxnet import nd from numpy.testing import assert_allclose """ Keras test """ model = Sequential() norm = normalization.BatchNormalization(center=False, scale=False, input_shape=(3, 4, 4)) model.add(norm) model.compile(loss='mse', optimizer='sgd') # centered on 5.0, variance 10.0 x = np.random.normal(loc=5.0, scale=10.0, size=(1000, 3, 4, 4)) model.fit(x, x, epochs=4, verbose=0, batch_size=32) out = model.predict(x) print("Results from keras:") print( "Mean: %s" % np.mean(out, axis=(0, 2, 3))) print( "Std: %s" % np.std(out, axis=(0, 2, 3))) assert_allclose(np.mean(out, axis=(0, 2, 3)), 0.0, atol=1e-1) assert_allclose(np.std(out, axis=(0, 2, 3)), 1.0, atol=1e-1) """ Gluon test """ print(mx.__version__) x = np.random.normal(loc=5.0, scale=10.0, size=(1000, 3, 4, 4)) x_nd = nd.array(x) net = gluon.nn.Sequential() ctx = mx.cpu() with net.name_scope(): net.add(gluon.nn.BatchNorm(scale=False, center=False)) net.collect_params().initialize(mx.init.Normal(sigma=.1), ctx=ctx) mse = gluon.loss.L2Loss() trainer = gluon.Trainer(net.collect_params(), 'sgd') epochs = 4 for e in range(epochs): cumulative_loss = 0 for i in range(1000): data = x_nd[i, :] label = x_nd[i, :] with autograd.record(): output = net(data) loss = mse(output, label) loss.backward(retain_graph=True) trainer.step(32) cumulative_loss += nd.sum(loss).asscalar() out = net(x_nd).asnumpy() print("Results from gluon:") print( "Mean: %s" % np.mean(out, axis=(0, 2, 3))) print( "Std: %s" % np.std(out, axis=(0, 2, 3))) assert_allclose(np.mean(out, axis=(0, 2, 3)), 0.0, atol=1e-1) assert_allclose(np.std(out, axis=(0, 2, 3)), 1.0, atol=1e-1) ``` **Error Message:** ``` Traceback (most recent call last): File "/Users/lawei/Documents/Notebooks/keras/gluon_batchnorm.py", line 56, in loss.backward(retain_graph=True) File "/Users/lawei/anaconda3/lib/python3.6/site-packages/mxnet/ndarray/ndarray.py", line 2130, in backward ctypes.c_void_p(0))) File "/Users/lawei/anaconda3/lib/python3.6/site-packages/mxnet/base.py", line 210, in check_call raise MXNetError(py_str(_LIB.MXGetLastError())) mxnet.base.MXNetError: [16:31:24] src/imperative/imperative.cc:285: Check failed: !AGInfo::IsNone(*i) Cannot differentiate node because it is not in a computational graph. You need to set is_recording to true or use autograd.record() to save computational graphs for backward. If you want to differentiate the same graph twice, you need to pass retain_graph=True to backward. Stack trace returned 6 entries: [bt] (0) 0 libmxnet.so 0x000111dfdeb4 libmxnet.so + 20148 [bt] (1) 1 libmxnet.so 0x000111dfdc6f libmxnet.so + 19567 [bt] (2) 2 libmxnet.so 0x000112f69389 MXNDListFree + 548393 [bt] (3) 3 libmxnet.so 0x000112ebe1fd MXAutogradBackwardEx + 893 [bt] (4) 4 libffi.6.dylib 0x000110670884 ffi_call_unix64 + 76 [bt] (5) 5 ??? 0x7fff4fcabf40 0x0 + 140734532075328 ``` **My Questions:** 1. gluon.nn.BatchNorm is not working with scale and beta both to False, need to fix 2. When changing scale=False, center=True, I was able to get std close to 1, but mean is not close
[GitHub] KellenSunderland commented on issue #11579: Fix arm64 docker build
KellenSunderland commented on issue #11579: Fix arm64 docker build URL: https://github.com/apache/incubator-mxnet/pull/11579#issuecomment-405412790 @larroy Did you address the comments? I see a few fields with a 'done' comment that don't look like they're updated. Is the PR missing a push? This is an automated message from the Apache Git Service. To respond to the message, please log on GitHub and use the URL above to go to the specific comment. For queries about this service, please contact Infrastructure at: us...@infra.apache.org With regards, Apache Git Services
[GitHub] ddavydenko edited a comment on issue #9724: undefined symbol: MXSymbolGetNumOutputs
ddavydenko edited a comment on issue #9724: undefined symbol: MXSymbolGetNumOutputs URL: https://github.com/apache/incubator-mxnet/issues/9724#issuecomment-404983772 This seems to be related to C API, not to front end C++ API. @sandeep-krishnamurthy , could you please update labels on this issue: remove "C++" and add "C API". This is an automated message from the Apache Git Service. To respond to the message, please log on GitHub and use the URL above to go to the specific comment. For queries about this service, please contact Infrastructure at: us...@infra.apache.org With regards, Apache Git Services
[GitHub] Roshrini edited a comment on issue #11555: Wrong conversion of `Split` operator of ONNX
Roshrini edited a comment on issue #11555: Wrong conversion of `Split` operator of ONNX URL: https://github.com/apache/incubator-mxnet/issues/11555#issuecomment-405406207 @marcoabreu @sandeep-krishnamurthy https://github.com/apache/incubator-mxnet/pull/11593 merged which solves this issue. Can you please close this issue? Thanks! This is an automated message from the Apache Git Service. To respond to the message, please log on GitHub and use the URL above to go to the specific comment. For queries about this service, please contact Infrastructure at: us...@infra.apache.org With regards, Apache Git Services
[GitHub] andrewfayres commented on a change in pull request #11605: Updating MultiTask example to use new infer api and adding test for CI
andrewfayres commented on a change in pull request #11605: Updating MultiTask example to use new infer api and adding test for CI URL: https://github.com/apache/incubator-mxnet/pull/11605#discussion_r202852156 ## File path: scala-package/examples/src/test/scala/org/apache/mxnetexamples/multitask/MultiTaskTest.scala ## @@ -0,0 +1,191 @@ +/* + * Licensed to the Apache Software Foundation (ASF) under one or more + * contributor license agreements. See the NOTICE file distributed with + * this work for additional information regarding copyright ownership. + * The ASF licenses this file to You under the Apache License, Version 2.0 + * (the "License"); you may not use this file except in compliance with + * the License. You may obtain a copy of the License at + * + *http://www.apache.org/licenses/LICENSE-2.0 + * + * Unless required by applicable law or agreed to in writing, software + * distributed under the License is distributed on an "AS IS" BASIS, + * WITHOUT WARRANTIES OR CONDITIONS OF ANY KIND, either express or implied. + * See the License for the specific language governing permissions and + * limitations under the License. + */ + +package org.apache.mxnetexamples.multitask + +import org.apache.commons.io.FileUtils +import org.apache.mxnet.Context +import org.scalatest.{BeforeAndAfterAll, FunSuite} +import org.slf4j.LoggerFactory +import org.apache.mxnet.Symbol +import org.apache.mxnet.DataIter +import org.apache.mxnet.DataBatch +import org.apache.mxnet.NDArray +import org.apache.mxnet.Shape +import org.apache.mxnet.EvalMetric +import org.apache.mxnet.Context +import org.apache.mxnet.Xavier +import org.apache.mxnet.optimizer.RMSProp + +import java.io.File +import java.net.URL + +import scala.sys.process.Process +import scala.collection.immutable.ListMap +import scala.collection.immutable.IndexedSeq + +import scala.collection.mutable.{ArrayBuffer, ListBuffer} + + +/** + * Integration test for imageClassifier example. + * This will run as a part of "make scalatest" + */ +class MultiTaskTest extends FunSuite with BeforeAndAfterAll { + + def getListOfFiles(dir: File): List[File] = dir.listFiles.filter(_.isFile).toList + + private val logger = LoggerFactory.getLogger(classOf[MultiTaskTest]) + + logger.info("Multitask Test...") + + val baseUrl = "https://s3.us-east-2.amazonaws.com/mxnet-scala/scala-example-ci; + val tempDirPath = System.getProperty("java.io.tmpdir") + val modelDirPath = tempDirPath + File.separator + "multitask/" + val tmpFile = new File(tempDirPath + "/multitask/mnist.zip") + if (!tmpFile.exists()) { +FileUtils.copyURLToFile(new URL(baseUrl + "/mnist/mnist.zip"), + tmpFile) + } + + // TODO: Need to confirm with Windows + + + Process("unzip " + tempDirPath + "/multitask/mnist.zip -d " ++ tempDirPath + "/multitask/") ! + + val batchSize = 100 + val numEpoch = 10 + val ctx = Context.cpu() Review comment: I did CPU on purpose because this is training on MNIST data. It trains on my macbook in just a few minutes. This is consistent with the other example that we have that uses mnist [here](https://github.com/apache/incubator-mxnet/blob/master/scala-package/examples/src/test/scala/org/apache/mxnetexamples/imclassification/MNISTExampleSuite.scala#L53). This is an automated message from the Apache Git Service. To respond to the message, please log on GitHub and use the URL above to go to the specific comment. For queries about this service, please contact Infrastructure at: us...@infra.apache.org With regards, Apache Git Services
[GitHub] ifeherva commented on issue #11056: [Feature Request] broadcast_like operator
ifeherva commented on issue #11056: [Feature Request] broadcast_like operator URL: https://github.com/apache/incubator-mxnet/issues/11056#issuecomment-405408044 Happy to do this next. This is an automated message from the Apache Git Service. To respond to the message, please log on GitHub and use the URL above to go to the specific comment. For queries about this service, please contact Infrastructure at: us...@infra.apache.org With regards, Apache Git Services
[GitHub] pracheer commented on a change in pull request #11773: Update PyPI version number
pracheer commented on a change in pull request #11773: Update PyPI version number URL: https://github.com/apache/incubator-mxnet/pull/11773#discussion_r202851221 ## File path: tools/coreml/pip_package/setup.py ## @@ -40,7 +40,7 @@ def readme(): return f.read() setup(name='mxnet-to-coreml', - version='0.1.0', + version='0.1.1', Review comment: https://pypi.org/project/mxnet-to-coreml/ seems to indicate we are already at 0.1.2. Update this to 0.1.3 instead? This is an automated message from the Apache Git Service. To respond to the message, please log on GitHub and use the URL above to go to the specific comment. For queries about this service, please contact Infrastructure at: us...@infra.apache.org With regards, Apache Git Services
[GitHub] Roshrini commented on issue #11555: Wrong conversion of `Split` operator of ONNX
Roshrini commented on issue #11555: Wrong conversion of `Split` operator of ONNX URL: https://github.com/apache/incubator-mxnet/issues/11555#issuecomment-405406207 @sandeep-krishnamurthy https://github.com/apache/incubator-mxnet/pull/11593 merged which solves this issue. Can you please close this issue? Thanks! This is an automated message from the Apache Git Service. To respond to the message, please log on GitHub and use the URL above to go to the specific comment. For queries about this service, please contact Infrastructure at: us...@infra.apache.org With regards, Apache Git Services
[GitHub] apeforest commented on issue #10349: Converting MXNet model to CoreML fails in param = node['attr']
apeforest commented on issue #10349: Converting MXNet model to CoreML fails in param = node['attr'] URL: https://github.com/apache/incubator-mxnet/issues/10349#issuecomment-405403180 @pracheer Please review and approve my PR for the PyPI update: https://github.com/apache/incubator-mxnet/pull/11773 This is an automated message from the Apache Git Service. To respond to the message, please log on GitHub and use the URL above to go to the specific comment. For queries about this service, please contact Infrastructure at: us...@infra.apache.org With regards, Apache Git Services
[GitHub] piyushghai commented on a change in pull request #11626: [MXNET-651] MXNet Model Backwards Compatibility Checker
piyushghai commented on a change in pull request #11626: [MXNET-651] MXNet Model Backwards Compatibility Checker URL: https://github.com/apache/incubator-mxnet/pull/11626#discussion_r202846947 ## File path: tests/nightly/model_backwards_compatibility_check/train_mxnet_legacy_models.sh ## @@ -0,0 +1,51 @@ +#!/usr/bin/env bash + +# Licensed to the Apache Software Foundation (ASF) under one +# or more contributor license agreements. See the NOTICE file +# distributed with this work for additional information +# regarding copyright ownership. The ASF licenses this file +# to you under the Apache License, Version 2.0 (the +# "License"); you may not use this file except in compliance +# with the License. You may obtain a copy of the License at +# +# http://www.apache.org/licenses/LICENSE-2.0 +# +# Unless required by applicable law or agreed to in writing, +# software distributed under the License is distributed on an +# "AS IS" BASIS, WITHOUT WARRANTIES OR CONDITIONS OF ANY +# KIND, either express or implied. See the License for the +# specific language governing permissions and limitations +# under the License. + +#Author: Piyush Ghai + +run_models() { + echo '==' + echo "Running training files and preparing models" + echo '==' + python model_backwards_compat_train.py + echo '==' +} + +install_mxnet() { + version=$1 + echo "Installing MXNet "$version + pip install mxnet==$version +} + +install_boto3(){ + echo "Intalling boto3" + pip install boto3 +} + +echo `pwd` +cd tests/nightly/model_backwards_compatibility_check +echo `pwd` + +install_boto3 + +install_mxnet 1.1.0 Review comment: Done. e764d5a2898d42f263688685bf575234a492a650 This is an automated message from the Apache Git Service. To respond to the message, please log on GitHub and use the URL above to go to the specific comment. For queries about this service, please contact Infrastructure at: us...@infra.apache.org With regards, Apache Git Services
[GitHub] piyushghai commented on a change in pull request #11626: [MXNET-651] MXNet Model Backwards Compatibility Checker
piyushghai commented on a change in pull request #11626: [MXNET-651] MXNet Model Backwards Compatibility Checker URL: https://github.com/apache/incubator-mxnet/pull/11626#discussion_r202846856 ## File path: tests/nightly/model_backwards_compatibility_check/common.py ## @@ -0,0 +1,199 @@ +#!/usr/bin/env python + +# Licensed to the Apache Software Foundation (ASF) under one +# or more contributor license agreements. See the NOTICE file +# distributed with this work for additional information +# regarding copyright ownership. The ASF licenses this file +# to you under the Apache License, Version 2.0 (the +# "License"); you may not use this file except in compliance +# with the License. You may obtain a copy of the License at +# +# http://www.apache.org/licenses/LICENSE-2.0 +# +# Unless required by applicable law or agreed to in writing, +# software distributed under the License is distributed on an +# "AS IS" BASIS, WITHOUT WARRANTIES OR CONDITIONS OF ANY +# KIND, either express or implied. See the License for the +# specific language governing permissions and limitations +# under the License. + + +import boto3 +import mxnet as mx +import json +import os +import numpy as np +import logging +from mxnet import nd, autograd, gluon +import mxnet.ndarray as nd +from mxnet.gluon.data.vision import transforms, datasets +from mxnet import autograd as ag +import mxnet.ndarray as F +from mxnet.gluon import nn, rnn +import re +import time +import sys +from mxnet.test_utils import assert_almost_equal + +# Set fixed random seeds. +mx.random.seed(7, ctx = mx.cpu()) +np.random.seed(7) +logging.getLogger().setLevel(logging.DEBUG) + +# get the current mxnet version we are running on +mxnet_version = mx.__version__ +model_bucket_name = 'mxnet-model-backwards-compatibility-models' +data_bucket_name = 'mxnet-model-backwards-compatibility-data' Review comment: Done. c80ee31 This is an automated message from the Apache Git Service. To respond to the message, please log on GitHub and use the URL above to go to the specific comment. For queries about this service, please contact Infrastructure at: us...@infra.apache.org With regards, Apache Git Services
[GitHub] sandeep-krishnamurthy closed pull request #11593: onnx split op import
sandeep-krishnamurthy closed pull request #11593: onnx split op import URL: https://github.com/apache/incubator-mxnet/pull/11593 This is a PR merged from a forked repository. As GitHub hides the original diff on merge, it is displayed below for the sake of provenance: As this is a foreign pull request (from a fork), the diff is supplied below (as it won't show otherwise due to GitHub magic): diff --git a/python/mxnet/contrib/onnx/onnx2mx/_op_translations.py b/python/mxnet/contrib/onnx/onnx2mx/_op_translations.py index 2b98aa08feb..a014f2d7f31 100644 --- a/python/mxnet/contrib/onnx/onnx2mx/_op_translations.py +++ b/python/mxnet/contrib/onnx/onnx2mx/_op_translations.py @@ -407,8 +407,22 @@ def cast(attrs, inputs, proto_obj): def split(attrs, inputs, proto_obj): """Splits an array along a particular axis into multiple sub-arrays.""" +split_list = attrs.get('split') if 'split' in attrs else [] new_attrs = translation_utils._fix_attribute_names(attrs, {'split' : 'num_outputs'}) +if 'axis' not in attrs: +new_attrs = translation_utils._add_extra_attributes(new_attrs, {'axis': 0}) + +if not split_list: +num_outputs = len(proto_obj.model_metadata.get('output_tensor_data')) +else: +raise NotImplementedError("Operator {} in MXNet does not support variable splits." + "Tracking the issue to support variable split here: " + "https://github.com/apache/incubator-mxnet/issues/11594; + .format('split')) + +new_attrs['num_outputs'] = num_outputs + return 'split', new_attrs, inputs def _slice(attrs, inputs, proto_obj): diff --git a/tests/python-pytest/onnx/import/mxnet_backend_rep.py b/tests/python-pytest/onnx/import/mxnet_backend_rep.py index 067ef156830..31a1935b187 100644 --- a/tests/python-pytest/onnx/import/mxnet_backend_rep.py +++ b/tests/python-pytest/onnx/import/mxnet_backend_rep.py @@ -82,4 +82,7 @@ def run(self, inputs, **kwargs): # run inference mod.forward(mx.io.DataBatch(data_forward)) result = mod.get_outputs()[0].asnumpy() +# split operator inference returns 1 less dimension +if self.symbol.name.startswith('split'): +return [i.asnumpy() for i in mod.get_outputs()] return [result] diff --git a/tests/python-pytest/onnx/import/test_cases.py b/tests/python-pytest/onnx/import/test_cases.py index f7addbb29b3..8af9500f29d 100644 --- a/tests/python-pytest/onnx/import/test_cases.py +++ b/tests/python-pytest/onnx/import/test_cases.py @@ -18,6 +18,7 @@ """Test Cases to be run for the import module""" IMPLEMENTED_OPERATORS_TEST = [ +'test_split_equal' 'test_random_uniform', 'test_random_normal', 'test_add', This is an automated message from the Apache Git Service. To respond to the message, please log on GitHub and use the URL above to go to the specific comment. For queries about this service, please contact Infrastructure at: us...@infra.apache.org With regards, Apache Git Services
[incubator-mxnet] branch master updated: onnx split op import (#11593)
This is an automated email from the ASF dual-hosted git repository. skm pushed a commit to branch master in repository https://gitbox.apache.org/repos/asf/incubator-mxnet.git The following commit(s) were added to refs/heads/master by this push: new e70f6ff onnx split op import (#11593) e70f6ff is described below commit e70f6ff51a30afd4b8d4afa6f281e9f95453bb4f Author: Roshani Nagmote AuthorDate: Mon Jul 16 15:38:14 2018 -0700 onnx split op import (#11593) * split operator import modified * split test cases partially enabled * lint fix * issue linked from error message --- python/mxnet/contrib/onnx/onnx2mx/_op_translations.py | 14 ++ tests/python-pytest/onnx/import/mxnet_backend_rep.py | 3 +++ tests/python-pytest/onnx/import/test_cases.py | 1 + 3 files changed, 18 insertions(+) diff --git a/python/mxnet/contrib/onnx/onnx2mx/_op_translations.py b/python/mxnet/contrib/onnx/onnx2mx/_op_translations.py index 61f342a..aa37856 100644 --- a/python/mxnet/contrib/onnx/onnx2mx/_op_translations.py +++ b/python/mxnet/contrib/onnx/onnx2mx/_op_translations.py @@ -409,8 +409,22 @@ def cast(attrs, inputs, proto_obj): def split(attrs, inputs, proto_obj): """Splits an array along a particular axis into multiple sub-arrays.""" +split_list = attrs.get('split') if 'split' in attrs else [] new_attrs = translation_utils._fix_attribute_names(attrs, {'split' : 'num_outputs'}) +if 'axis' not in attrs: +new_attrs = translation_utils._add_extra_attributes(new_attrs, {'axis': 0}) + +if not split_list: +num_outputs = len(proto_obj.model_metadata.get('output_tensor_data')) +else: +raise NotImplementedError("Operator {} in MXNet does not support variable splits." + "Tracking the issue to support variable split here: " + "https://github.com/apache/incubator-mxnet/issues/11594; + .format('split')) + +new_attrs['num_outputs'] = num_outputs + return 'split', new_attrs, inputs def _slice(attrs, inputs, proto_obj): diff --git a/tests/python-pytest/onnx/import/mxnet_backend_rep.py b/tests/python-pytest/onnx/import/mxnet_backend_rep.py index 067ef15..31a1935 100644 --- a/tests/python-pytest/onnx/import/mxnet_backend_rep.py +++ b/tests/python-pytest/onnx/import/mxnet_backend_rep.py @@ -82,4 +82,7 @@ class MXNetBackendRep(BackendRep): # run inference mod.forward(mx.io.DataBatch(data_forward)) result = mod.get_outputs()[0].asnumpy() +# split operator inference returns 1 less dimension +if self.symbol.name.startswith('split'): +return [i.asnumpy() for i in mod.get_outputs()] return [result] diff --git a/tests/python-pytest/onnx/import/test_cases.py b/tests/python-pytest/onnx/import/test_cases.py index 24265bd..d2574db 100644 --- a/tests/python-pytest/onnx/import/test_cases.py +++ b/tests/python-pytest/onnx/import/test_cases.py @@ -18,6 +18,7 @@ """Test Cases to be run for the import module""" IMPLEMENTED_OPERATORS_TEST = [ +'test_split_equal' 'test_random_uniform', 'test_random_normal', 'test_add',
[GitHub] lebeg commented on issue #11148: [MXNET-679] Refactor handling BLAS libraries with cmake
lebeg commented on issue #11148: [MXNET-679] Refactor handling BLAS libraries with cmake URL: https://github.com/apache/incubator-mxnet/pull/11148#issuecomment-405402956 Thanks! Yes, probably building from source section would be the right place, what do you think? This is an automated message from the Apache Git Service. To respond to the message, please log on GitHub and use the URL above to go to the specific comment. For queries about this service, please contact Infrastructure at: us...@infra.apache.org With regards, Apache Git Services
[GitHub] zhreshold commented on issue #11421: Can't iterate custom dataset by dataloader with 'num_workers > 1' param on Linux
zhreshold commented on issue #11421: Can't iterate custom dataset by dataloader with 'num_workers > 1' param on Linux URL: https://github.com/apache/incubator-mxnet/issues/11421#issuecomment-405401234 As far as I know, opencv seems to be not fork friendly, and therefore will cause all kinds of bug in multiprocessing. This is an automated message from the Apache Git Service. To respond to the message, please log on GitHub and use the URL above to go to the specific comment. For queries about this service, please contact Infrastructure at: us...@infra.apache.org With regards, Apache Git Services
[GitHub] reminisce commented on issue #11756: [MXNET-677] int8 quantization does not work on toy mnist dataset
reminisce commented on issue #11756: [MXNET-677] int8 quantization does not work on toy mnist dataset URL: https://github.com/apache/incubator-mxnet/issues/11756#issuecomment-405400288 CPU quantization was implemented by Intel engineers. I've already informed them offline and they will take this forward. You are right, the invitation to the slack channel has not been sent out yet. You should be able to receive that soon. This is an automated message from the Apache Git Service. To respond to the message, please log on GitHub and use the URL above to go to the specific comment. For queries about this service, please contact Infrastructure at: us...@infra.apache.org With regards, Apache Git Services
[GitHub] aaronmarkham commented on a change in pull request #11148: [MXNET-679] Refactor handling BLAS libraries with cmake
aaronmarkham commented on a change in pull request #11148: [MXNET-679] Refactor handling BLAS libraries with cmake URL: https://github.com/apache/incubator-mxnet/pull/11148#discussion_r202841317 ## File path: cmake/Modules/FindAccelerate.cmake ## @@ -42,6 +66,6 @@ if(Accelerate_FOUND) set(Accelerate_LIBRARIES "-framework Accelerate") mark_as_advanced(${LOOKED_FOR}) - message(STATUS "Found Accelerate (include: ${Accelerate_CBLAS_INCLUDE_DIR}, library: ${Accelerate_BLAS_LIBRARY})") + message(STATUS "Found Accelerate (include: ${Accelerate_CBLAS_INCLUDE_DIR}, library: ${Accelerate_LIBRARIES})") Review comment: Apple Accelerate This is an automated message from the Apache Git Service. To respond to the message, please log on GitHub and use the URL above to go to the specific comment. For queries about this service, please contact Infrastructure at: us...@infra.apache.org With regards, Apache Git Services
[GitHub] aaronmarkham commented on a change in pull request #11148: [MXNET-679] Refactor handling BLAS libraries with cmake
aaronmarkham commented on a change in pull request #11148: [MXNET-679] Refactor handling BLAS libraries with cmake URL: https://github.com/apache/incubator-mxnet/pull/11148#discussion_r202841266 ## File path: cmake/Modules/FindAccelerate.cmake ## @@ -19,20 +19,44 @@ # # The following are set after configuration is done: # Accelerate_FOUND -# Accelerate_INCLUDE_DIRS +# Accelerate_LAPACK_FOUND +# Accelerate_INCLUDE_DIR # Accelerate_LIBRARIES file(TO_CMAKE_PATH "$ENV{Accelerate_HOME}" Accelerate_HOME) + set(Accelerate_INCLUDE_SEARCH_PATHS - /System/Library/Frameworks/Accelerate.framework/Versions/Current/Frameworks/vecLib.framework/Versions/Current - ${Accelerate_HOME} -) + /System/Library/Frameworks/Accelerate.framework/Versions/Current/Frameworks/vecLib.framework/Versions/Current +${Accelerate_HOME} +) -find_path(Accelerate_CBLAS_INCLUDE_DIR NAMES cblas.h PATHS ${Accelerate_INCLUDE_SEARCH_PATHS} PATH_SUFFIXES Headers) +find_path(Accelerate_CBLAS_INCLUDE_DIR + NAMES cblas.h + PATHS ${Accelerate_INCLUDE_SEARCH_PATHS} + PATH_SUFFIXES Headers) set(LOOKED_FOR Accelerate_CBLAS_INCLUDE_DIR -) +) + +if(Accelerate_NEED_LAPACK) + message(STATUS "Looking for lapack support...") + + # Apples vecLib should contain lapack functionalities included in the Accelerate framework, but we will double check + # https://developer.apple.com/documentation/accelerate/veclib?changes=_2 + include(CheckFunctionExists) + set(CMAKE_REQUIRED_LIBRARIES "-framework Accelerate") + check_function_exists("cgees_" LAPACK_FOUND) + + if(LAPACK_FOUND) +set(Accelerate_LAPACK_FOUND True) +message(STATUS "Lapack found") + else() +set(Accelerate_LAPACK_FOUND False) +message(WARNING "Accelerate lapack support could not be identified, lapack functionality will not be available") Review comment: Apple Accelerate's LAPACK LAPACK functionality... This is an automated message from the Apache Git Service. To respond to the message, please log on GitHub and use the URL above to go to the specific comment. For queries about this service, please contact Infrastructure at: us...@infra.apache.org With regards, Apache Git Services
[GitHub] aaronmarkham commented on a change in pull request #11148: [MXNET-679] Refactor handling BLAS libraries with cmake
aaronmarkham commented on a change in pull request #11148: [MXNET-679] Refactor handling BLAS libraries with cmake URL: https://github.com/apache/incubator-mxnet/pull/11148#discussion_r202841694 ## File path: cmake/Modules/FindOpenBLAS.cmake ## @@ -15,77 +15,133 @@ # specific language governing permissions and limitations # under the License. +# Find the OpenBLAS libraries +# +# The following variables are optionally searched for defaults +# +# OpenBLAS_HOME:Base directory where all OpenBLAS components are found +# OpenBLAS_NEED_LAPACK: Whether need lapack libraries +# +# The following are set after configuration is done: +# +# OpenBLAS_FOUND +# OpenBLAS_LAPACK_FOUND +# OpenBLAS_INCLUDE_DIRS +# OpenBLAS_LIBRARIES + file(TO_CMAKE_PATH "$ENV{OpenBLAS_HOME}" OpenBLAS_HOME) file(TO_CMAKE_PATH "$ENV{OpenBLAS}" OpenBLAS_DIR) +file(TO_CMAKE_PATH "$ENV{CROSS_ROOT}" CROSS_ROOT) + +if(CMAKE_CROSSCOMPILING) + set(OpenBLAS_INCLUDE_SEARCH_PATHS + ${CROSS_ROOT} + ${CROSS_ROOT}/include + ) +endif() + +set(OpenBLAS_INCLUDE_SEARCH_PATHS +${OpenBLAS_INCLUDE_SEARCH_PATHS} + +${OpenBLAS_HOME} +${OpenBLAS_HOME}/include + +/usr/include +/usr/include/openblas +/usr/include/openblas-base +/usr/local/include +/usr/local/include/openblas +/usr/local/include/openblas-base +/opt/OpenBLAS/include +/usr/local/opt/openblas/include + +${PROJECT_SOURCE_DIR}/3rdparty/OpenBLAS/include +${PROJECT_SOURCE_DIR}/thirdparty/OpenBLAS/include +) + +if(CMAKE_CROSSCOMPILING) + set(Open_BLAS_LIB_SEARCH_PATHS + ${CROSS_ROOT} + ${CROSS_ROOT}/include + ) +endif() + +set(OpenBLAS_LIB_SEARCH_PATHS +${OpenBLAS_LIB_SEARCH_PATHS} + +${OpenBLAS_DIR} +${OpenBLAS_DIR}/lib +${OpenBLAS_DIR}/lib64 +${OpenBLAS_HOME} +${OpenBLAS_HOME}/lib +${OpenBLAS_HOME}/lib64 -SET(Open_BLAS_INCLUDE_SEARCH_PATHS - /usr/include - /usr/include/openblas - /usr/include/openblas-base - /usr/local/include - /usr/local/include/openblas - /usr/local/include/openblas-base - /opt/OpenBLAS/include - /usr/local/opt/openblas/include - ${PROJECT_SOURCE_DIR}/3rdparty/OpenBLAS/include - ${PROJECT_SOURCE_DIR}/thirdparty/OpenBLAS/include - ${OpenBLAS_HOME} - ${OpenBLAS_HOME}/include -) - -SET(Open_BLAS_LIB_SEARCH_PATHS -/lib/ -/lib/openblas-base -/lib64/ -/usr/lib -/usr/lib/openblas-base -/usr/lib64 -/usr/local/lib -/usr/local/lib64 -/opt/OpenBLAS/lib -/usr/local/opt/openblas/lib -${PROJECT_SOURCE_DIR}/3rdparty/OpenBLAS/lib -${PROJECT_SOURCE_DIR}/thirdparty/OpenBLAS/lib - ${OpenBLAS_DIR} - ${OpenBLAS_DIR}/lib -${OpenBLAS_HOME} -${OpenBLAS_HOME}/lib - ) - -FIND_PATH(OpenBLAS_INCLUDE_DIR NAMES cblas.h PATHS ${Open_BLAS_INCLUDE_SEARCH_PATHS}) -FIND_LIBRARY(OpenBLAS_LIB NAMES openblas PATHS ${Open_BLAS_LIB_SEARCH_PATHS}) -IF(NOT OpenBLAS_LIB) - FIND_FILE(OpenBLAS_LIB NAMES libopenblas.dll.a PATHS ${Open_BLAS_LIB_SEARCH_PATHS}) -ENDIF() - -SET(OpenBLAS_FOUND ON) - -#Check include files -IF(NOT OpenBLAS_INCLUDE_DIR) -SET(OpenBLAS_FOUND OFF) -MESSAGE(STATUS "Could not find OpenBLAS include. Turning OpenBLAS_FOUND off") -ENDIF() - -#Check libraries -IF(NOT OpenBLAS_LIB) -SET(OpenBLAS_FOUND OFF) -MESSAGE(STATUS "Could not find OpenBLAS lib. Turning OpenBLAS_FOUND off") -ENDIF() - -IF (OpenBLAS_FOUND) - IF (NOT OpenBLAS_FIND_QUIETLY) -MESSAGE(STATUS "Found OpenBLAS libraries: ${OpenBLAS_LIB}") -MESSAGE(STATUS "Found OpenBLAS include: ${OpenBLAS_INCLUDE_DIR}") - ENDIF (NOT OpenBLAS_FIND_QUIETLY) -ELSE (OpenBLAS_FOUND) - IF (OpenBLAS_FIND_REQUIRED) -MESSAGE(FATAL_ERROR "Could not find OpenBLAS") - ENDIF (OpenBLAS_FIND_REQUIRED) -ENDIF (OpenBLAS_FOUND) - -MARK_AS_ADVANCED( +/lib/ +/lib/openblas-base +/lib64/ +/usr/lib +/usr/lib/openblas-base +/usr/lib64 +/usr/local/lib +/usr/local/lib64 +/opt/OpenBLAS/lib +/usr/local/opt/openblas/lib + +${PROJECT_SOURCE_DIR}/3rdparty/OpenBLAS/lib +${PROJECT_SOURCE_DIR}/thirdparty/OpenBLAS/lib +) + +find_path(OpenBLAS_INCLUDE_DIR + NAMES cblas.h + PATHS ${OpenBLAS_INCLUDE_SEARCH_PATHS}) +find_library(OpenBLAS_LIBRARY + NAMES libopenblas.a openblas libopenblas.dll.a libopenblas.dll + PATHS ${OpenBLAS_LIB_SEARCH_PATHS}) + +set(LOOKED_FOR OpenBLAS_INCLUDE_DIR -OpenBLAS_LIB -OpenBLAS -) +OpenBLAS_LIBRARY +) + +if(OpenBLAS_NEED_LAPACK) + message(STATUS "Looking for lapack support...") + + find_path(OpenBLAS_LAPACK_INCLUDE_DIR +NAMES lapacke.h +PATHS ${OpenBLAS_INCLUDE_SEARCH_PATHS}) + + # OpenBLAS does not have a separate lapack library: https://github.com/xianyi/OpenBLAS/issues/296 + # lapack if present in OpenBLAS build is included into libopenblas + set(OpenBLAS_LAPACK_LIBRARY ${OpenBLAS_LIBRARY}) + +
[GitHub] aaronmarkham commented on a change in pull request #11148: [MXNET-679] Refactor handling BLAS libraries with cmake
aaronmarkham commented on a change in pull request #11148: [MXNET-679] Refactor handling BLAS libraries with cmake URL: https://github.com/apache/incubator-mxnet/pull/11148#discussion_r202840076 ## File path: cmake/ChooseBlas.cmake ## @@ -16,43 +16,199 @@ # under the License. set(BLAS "Open" CACHE STRING "Selected BLAS library") -set_property(CACHE BLAS PROPERTY STRINGS "Atlas;Open;MKL") +set_property(CACHE BLAS PROPERTY STRINGS "Atlas;Open;MKL;Apple") -if(USE_MKL_IF_AVAILABLE) - if(NOT MKL_FOUND) +function(switch_lapack enable) + if(${enable}) +message(STATUS "Enabling lapack functionality") +add_definitions(-DMXNET_USE_LAPACK=1) + else() +message(WARNING "Lapack functionality not available") + endif() +endfunction() + +function(try_mkldnn) + + if(NOT ${USE_MKLDNN}) +return() + endif() + + if(${CMAKE_CROSSCOMPILING}) +message(WARNING "MKLDNN with cross compilation is not supported, MKLDNN will not be available") +return() + endif() + + if(NOT ${MKL_FOUND}) +message(WARNING "MKLDNN requires either MKL or MKLML, MKLDNN will not be available") +return() + endif() + + message(STATUS "Adding MKLDNN to the build") + + # CPU architecture (e.g., C5) can't run on another architecture (e.g., g3). + if(NOT MSVC) +set(ARCH_OPT_FLAGS ${ARCH_OPT_FLAGS} "-mtune=generic" PARENT_SCOPE) + endif() + + set(WITH_TEST OFF) + set(WITH_EXAMPLE OFF) + add_subdirectory(3rdparty/mkldnn) + + include_directories(3rdparty/mkldnn/include) + set(mxnet_LINKER_LIBS ${mxnet_LINKER_LIBS} mkldnn PARENT_SCOPE) + + add_definitions(-DUSE_MKL=1) + add_definitions(-DCUB_MKL=1) + add_definitions(-DMXNET_USE_MKLDNN=1) + +endfunction() + +function(try_mkl) + if(${USE_MKL_IF_AVAILABLE} AND NOT ${USE_MKLML}) +message(STATUS "Trying to enable mkl framework due to USE_MKL_IF_AVAILABLE") find_package(MKL) +if(${MKL_FOUND}) + message(STATUS "MKL framework found") + + set(MKL_FOUND ${MKL_FOUND} PARENT_SCOPE) + set(MKLROOT ${MKLROOT} PARENT_SCOPE) + + set(BLAS MKL PARENT_SCOPE) +else() + message(STATUS "MKL framework not found") +endif() endif() - if(MKL_FOUND) - if(USE_MKLDNN) - set(BLAS "open") +endfunction() + +function(try_mklml) + if(NOT ${USE_MKLML}) +return() + endif() + + if(${MKL_FOUND}) +return() + endif() + + message(STATUS "Trying to enable mklml framework due to USE_MKLML") + + include(${CMAKE_CURRENT_LIST_DIR}/DownloadMKLML.cmake) + find_package(MKLML REQUIRED) + include_directories(SYSTEM ${MKLML_INCLUDE_DIRS}) + set(mxnet_LINKER_LIBS ${mxnet_LINKER_LIBS} ${MKLML_LIBRARIES} PARENT_SCOPE) + + set(MKL_FOUND ${MKL_FOUND} PARENT_SCOPE) + set(MKLROOT ${MKLROOT} PARENT_SCOPE) + + set(BLAS MKL PARENT_SCOPE) + +endfunction() + +function(try_accelerate) + if(NOT APPLE) +return() + endif() + + if(BLAS MATCHES "[Mm][Kk][Ll]") +return() + endif() + + if(${USE_ACCELERATE_IF_AVAILABLE}) +message(STATUS "Trying to enable accelerate framework due to USE_ACCELERATE_IF_AVAILABLE") +find_package(Accelerate) +if(${Accelerate_FOUND}) + message(STATUS "Accelerate framework found") Review comment: Apple Accelerate This is an automated message from the Apache Git Service. To respond to the message, please log on GitHub and use the URL above to go to the specific comment. For queries about this service, please contact Infrastructure at: us...@infra.apache.org With regards, Apache Git Services
[GitHub] aaronmarkham commented on a change in pull request #11148: [MXNET-679] Refactor handling BLAS libraries with cmake
aaronmarkham commented on a change in pull request #11148: [MXNET-679] Refactor handling BLAS libraries with cmake URL: https://github.com/apache/incubator-mxnet/pull/11148#discussion_r202841058 ## File path: cmake/Modules/FindAccelerate.cmake ## @@ -19,20 +19,44 @@ # # The following are set after configuration is done: # Accelerate_FOUND -# Accelerate_INCLUDE_DIRS +# Accelerate_LAPACK_FOUND +# Accelerate_INCLUDE_DIR # Accelerate_LIBRARIES file(TO_CMAKE_PATH "$ENV{Accelerate_HOME}" Accelerate_HOME) + set(Accelerate_INCLUDE_SEARCH_PATHS - /System/Library/Frameworks/Accelerate.framework/Versions/Current/Frameworks/vecLib.framework/Versions/Current - ${Accelerate_HOME} -) + /System/Library/Frameworks/Accelerate.framework/Versions/Current/Frameworks/vecLib.framework/Versions/Current +${Accelerate_HOME} +) -find_path(Accelerate_CBLAS_INCLUDE_DIR NAMES cblas.h PATHS ${Accelerate_INCLUDE_SEARCH_PATHS} PATH_SUFFIXES Headers) +find_path(Accelerate_CBLAS_INCLUDE_DIR + NAMES cblas.h + PATHS ${Accelerate_INCLUDE_SEARCH_PATHS} + PATH_SUFFIXES Headers) set(LOOKED_FOR Accelerate_CBLAS_INCLUDE_DIR -) +) + +if(Accelerate_NEED_LAPACK) + message(STATUS "Looking for lapack support...") Review comment: LAPACK This is an automated message from the Apache Git Service. To respond to the message, please log on GitHub and use the URL above to go to the specific comment. For queries about this service, please contact Infrastructure at: us...@infra.apache.org With regards, Apache Git Services
[GitHub] zheng-da commented on issue #10724: Flaky test MKLDNN test_bucket_module
zheng-da commented on issue #10724: Flaky test MKLDNN test_bucket_module URL: https://github.com/apache/incubator-mxnet/issues/10724#issuecomment-405399856 how did you test it? I tried putting a loop in the test code and run it 1000 times. ```Python for i in range(1000): test_bucket_module() ``` I don't see segfault either. Originally, I can reproduce the bug in this way. It seems https://github.com/apache/incubator-mxnet/pull/11577 fixed the bug. But if I run ``` for i in {1..1000}; do python tests/python/train/test_bucketing.py; done ``` I can see a segfault occasionally. I'm not sure if this segfault is the same segfault. This is an automated message from the Apache Git Service. To respond to the message, please log on GitHub and use the URL above to go to the specific comment. For queries about this service, please contact Infrastructure at: us...@infra.apache.org With regards, Apache Git Services
[GitHub] eric-haibin-lin removed a comment on issue #11218: Feature request: randint operator
eric-haibin-lin removed a comment on issue #11218: Feature request: randint operator URL: https://github.com/apache/incubator-mxnet/issues/11218#issuecomment-405398805 @mseth25 This is an automated message from the Apache Git Service. To respond to the message, please log on GitHub and use the URL above to go to the specific comment. For queries about this service, please contact Infrastructure at: us...@infra.apache.org With regards, Apache Git Services
[GitHub] christ1ne closed issue #11770: [quantization] unexpected keyword argument 'quantized_dtype'
christ1ne closed issue #11770: [quantization] unexpected keyword argument 'quantized_dtype' URL: https://github.com/apache/incubator-mxnet/issues/11770 This is an automated message from the Apache Git Service. To respond to the message, please log on GitHub and use the URL above to go to the specific comment. For queries about this service, please contact Infrastructure at: us...@infra.apache.org With regards, Apache Git Services
[GitHub] aaronmarkham commented on a change in pull request #11148: [MXNET-679] Refactor handling BLAS libraries with cmake
aaronmarkham commented on a change in pull request #11148: [MXNET-679] Refactor handling BLAS libraries with cmake URL: https://github.com/apache/incubator-mxnet/pull/11148#discussion_r202839878 ## File path: cmake/ChooseBlas.cmake ## @@ -16,43 +16,199 @@ # under the License. set(BLAS "Open" CACHE STRING "Selected BLAS library") -set_property(CACHE BLAS PROPERTY STRINGS "Atlas;Open;MKL") +set_property(CACHE BLAS PROPERTY STRINGS "Atlas;Open;MKL;Apple") -if(USE_MKL_IF_AVAILABLE) - if(NOT MKL_FOUND) +function(switch_lapack enable) + if(${enable}) +message(STATUS "Enabling lapack functionality") +add_definitions(-DMXNET_USE_LAPACK=1) + else() +message(WARNING "Lapack functionality not available") + endif() +endfunction() + +function(try_mkldnn) + + if(NOT ${USE_MKLDNN}) +return() + endif() + + if(${CMAKE_CROSSCOMPILING}) +message(WARNING "MKLDNN with cross compilation is not supported, MKLDNN will not be available") +return() + endif() + + if(NOT ${MKL_FOUND}) +message(WARNING "MKLDNN requires either MKL or MKLML, MKLDNN will not be available") +return() + endif() + + message(STATUS "Adding MKLDNN to the build") + + # CPU architecture (e.g., C5) can't run on another architecture (e.g., g3). + if(NOT MSVC) +set(ARCH_OPT_FLAGS ${ARCH_OPT_FLAGS} "-mtune=generic" PARENT_SCOPE) + endif() + + set(WITH_TEST OFF) + set(WITH_EXAMPLE OFF) + add_subdirectory(3rdparty/mkldnn) + + include_directories(3rdparty/mkldnn/include) + set(mxnet_LINKER_LIBS ${mxnet_LINKER_LIBS} mkldnn PARENT_SCOPE) + + add_definitions(-DUSE_MKL=1) + add_definitions(-DCUB_MKL=1) + add_definitions(-DMXNET_USE_MKLDNN=1) + +endfunction() + +function(try_mkl) + if(${USE_MKL_IF_AVAILABLE} AND NOT ${USE_MKLML}) +message(STATUS "Trying to enable mkl framework due to USE_MKL_IF_AVAILABLE") Review comment: MKL This is an automated message from the Apache Git Service. To respond to the message, please log on GitHub and use the URL above to go to the specific comment. For queries about this service, please contact Infrastructure at: us...@infra.apache.org With regards, Apache Git Services
[GitHub] aaronmarkham commented on a change in pull request #11148: [MXNET-679] Refactor handling BLAS libraries with cmake
aaronmarkham commented on a change in pull request #11148: [MXNET-679] Refactor handling BLAS libraries with cmake URL: https://github.com/apache/incubator-mxnet/pull/11148#discussion_r202840871 ## File path: cmake/ChooseBlas.cmake ## @@ -16,43 +16,199 @@ # under the License. set(BLAS "Open" CACHE STRING "Selected BLAS library") -set_property(CACHE BLAS PROPERTY STRINGS "Atlas;Open;MKL") +set_property(CACHE BLAS PROPERTY STRINGS "Atlas;Open;MKL;Apple") -if(USE_MKL_IF_AVAILABLE) - if(NOT MKL_FOUND) +function(switch_lapack enable) + if(${enable}) +message(STATUS "Enabling lapack functionality") +add_definitions(-DMXNET_USE_LAPACK=1) + else() +message(WARNING "Lapack functionality not available") + endif() +endfunction() + +function(try_mkldnn) + + if(NOT ${USE_MKLDNN}) +return() + endif() + + if(${CMAKE_CROSSCOMPILING}) +message(WARNING "MKLDNN with cross compilation is not supported, MKLDNN will not be available") +return() + endif() + + if(NOT ${MKL_FOUND}) +message(WARNING "MKLDNN requires either MKL or MKLML, MKLDNN will not be available") +return() + endif() + + message(STATUS "Adding MKLDNN to the build") + + # CPU architecture (e.g., C5) can't run on another architecture (e.g., g3). + if(NOT MSVC) +set(ARCH_OPT_FLAGS ${ARCH_OPT_FLAGS} "-mtune=generic" PARENT_SCOPE) + endif() + + set(WITH_TEST OFF) + set(WITH_EXAMPLE OFF) + add_subdirectory(3rdparty/mkldnn) + + include_directories(3rdparty/mkldnn/include) + set(mxnet_LINKER_LIBS ${mxnet_LINKER_LIBS} mkldnn PARENT_SCOPE) + + add_definitions(-DUSE_MKL=1) + add_definitions(-DCUB_MKL=1) + add_definitions(-DMXNET_USE_MKLDNN=1) + +endfunction() + +function(try_mkl) + if(${USE_MKL_IF_AVAILABLE} AND NOT ${USE_MKLML}) +message(STATUS "Trying to enable mkl framework due to USE_MKL_IF_AVAILABLE") find_package(MKL) +if(${MKL_FOUND}) + message(STATUS "MKL framework found") + + set(MKL_FOUND ${MKL_FOUND} PARENT_SCOPE) + set(MKLROOT ${MKLROOT} PARENT_SCOPE) + + set(BLAS MKL PARENT_SCOPE) +else() + message(STATUS "MKL framework not found") +endif() endif() - if(MKL_FOUND) - if(USE_MKLDNN) - set(BLAS "open") +endfunction() + +function(try_mklml) + if(NOT ${USE_MKLML}) +return() + endif() + + if(${MKL_FOUND}) +return() + endif() + + message(STATUS "Trying to enable mklml framework due to USE_MKLML") + + include(${CMAKE_CURRENT_LIST_DIR}/DownloadMKLML.cmake) + find_package(MKLML REQUIRED) + include_directories(SYSTEM ${MKLML_INCLUDE_DIRS}) + set(mxnet_LINKER_LIBS ${mxnet_LINKER_LIBS} ${MKLML_LIBRARIES} PARENT_SCOPE) + + set(MKL_FOUND ${MKL_FOUND} PARENT_SCOPE) + set(MKLROOT ${MKLROOT} PARENT_SCOPE) + + set(BLAS MKL PARENT_SCOPE) + +endfunction() + +function(try_accelerate) + if(NOT APPLE) +return() + endif() + + if(BLAS MATCHES "[Mm][Kk][Ll]") +return() + endif() + + if(${USE_ACCELERATE_IF_AVAILABLE}) +message(STATUS "Trying to enable accelerate framework due to USE_ACCELERATE_IF_AVAILABLE") +find_package(Accelerate) +if(${Accelerate_FOUND}) + message(STATUS "Accelerate framework found") + set(BLAS Accelerate PARENT_SCOPE) else() - set(BLAS "MKL") + message(STATUS "Accelerate framework not found") endif() endif() -endif() +endfunction() -if(BLAS STREQUAL "Atlas" OR BLAS STREQUAL "atlas") +try_mkl() +try_mklml() +try_mkldnn() +try_accelerate() + +# cmake regexp does not support case insensitive match (?i) or //i +if(BLAS MATCHES "[Aa][Tt][Ll][Aa][Ss]") + message(STATUS "Using Atlas for BLAS") + + set(Atlas_NEED_LAPACK ${USE_LAPACK}) find_package(Atlas REQUIRED) - include_directories(SYSTEM ${Atlas_INCLUDE_DIR}) - list(APPEND mshadow_LINKER_LIBS ${Atlas_LIBRARIES}) + + include_directories(SYSTEM ${Atlas_INCLUDE_DIRS}) + set(mxnet_LINKER_LIBS ${mxnet_LINKER_LIBS} ${Atlas_LIBRARIES}) + add_definitions(-DMSHADOW_USE_CBLAS=1) add_definitions(-DMSHADOW_USE_MKL=0) -elseif(BLAS STREQUAL "Open" OR BLAS STREQUAL "open") + + switch_lapack(${USE_LAPACK} AND ${Atlas_LAPACK_FOUND}) + + return() +endif() + +if(BLAS MATCHES "[Oo][Pp][Ee][Nn]") + message(STATUS "Using OpenBLAS for BLAS") + + set(OpenBLAS_NEED_LAPACK ${USE_LAPACK}) + find_package(OpenBLAS REQUIRED) - include_directories(SYSTEM ${OpenBLAS_INCLUDE_DIR}) - list(APPEND mshadow_LINKER_LIBS ${OpenBLAS_LIB}) + + include_directories(SYSTEM ${OpenBLAS_INCLUDE_DIRS}) + set(mxnet_LINKER_LIBS ${mxnet_LINKER_LIBS} ${OpenBLAS_LIBRARIES}) + add_definitions(-DMSHADOW_USE_CBLAS=1) add_definitions(-DMSHADOW_USE_MKL=0) -elseif(BLAS STREQUAL "MKL" OR BLAS STREQUAL "mkl") + + switch_lapack(${USE_LAPACK} AND ${OpenBLAS_LAPACK_FOUND}) + + return() +endif() + +if(BLAS MATCHES "[Mm][Kk][Ll]") + message(STATUS "Using MKL for BLAS") + find_package(MKL REQUIRED) + include_directories(SYSTEM ${MKL_INCLUDE_DIR}) - list(APPEND mshadow_LINKER_LIBS ${MKL_LIBRARIES}) +
[GitHub] aaronmarkham commented on a change in pull request #11148: [MXNET-679] Refactor handling BLAS libraries with cmake
aaronmarkham commented on a change in pull request #11148: [MXNET-679] Refactor handling BLAS libraries with cmake URL: https://github.com/apache/incubator-mxnet/pull/11148#discussion_r202839993 ## File path: cmake/ChooseBlas.cmake ## @@ -16,43 +16,199 @@ # under the License. set(BLAS "Open" CACHE STRING "Selected BLAS library") -set_property(CACHE BLAS PROPERTY STRINGS "Atlas;Open;MKL") +set_property(CACHE BLAS PROPERTY STRINGS "Atlas;Open;MKL;Apple") -if(USE_MKL_IF_AVAILABLE) - if(NOT MKL_FOUND) +function(switch_lapack enable) + if(${enable}) +message(STATUS "Enabling lapack functionality") +add_definitions(-DMXNET_USE_LAPACK=1) + else() +message(WARNING "Lapack functionality not available") + endif() +endfunction() + +function(try_mkldnn) + + if(NOT ${USE_MKLDNN}) +return() + endif() + + if(${CMAKE_CROSSCOMPILING}) +message(WARNING "MKLDNN with cross compilation is not supported, MKLDNN will not be available") +return() + endif() + + if(NOT ${MKL_FOUND}) +message(WARNING "MKLDNN requires either MKL or MKLML, MKLDNN will not be available") +return() + endif() + + message(STATUS "Adding MKLDNN to the build") + + # CPU architecture (e.g., C5) can't run on another architecture (e.g., g3). + if(NOT MSVC) +set(ARCH_OPT_FLAGS ${ARCH_OPT_FLAGS} "-mtune=generic" PARENT_SCOPE) + endif() + + set(WITH_TEST OFF) + set(WITH_EXAMPLE OFF) + add_subdirectory(3rdparty/mkldnn) + + include_directories(3rdparty/mkldnn/include) + set(mxnet_LINKER_LIBS ${mxnet_LINKER_LIBS} mkldnn PARENT_SCOPE) + + add_definitions(-DUSE_MKL=1) + add_definitions(-DCUB_MKL=1) + add_definitions(-DMXNET_USE_MKLDNN=1) + +endfunction() + +function(try_mkl) + if(${USE_MKL_IF_AVAILABLE} AND NOT ${USE_MKLML}) +message(STATUS "Trying to enable mkl framework due to USE_MKL_IF_AVAILABLE") find_package(MKL) +if(${MKL_FOUND}) + message(STATUS "MKL framework found") + + set(MKL_FOUND ${MKL_FOUND} PARENT_SCOPE) + set(MKLROOT ${MKLROOT} PARENT_SCOPE) + + set(BLAS MKL PARENT_SCOPE) +else() + message(STATUS "MKL framework not found") +endif() endif() - if(MKL_FOUND) - if(USE_MKLDNN) - set(BLAS "open") +endfunction() + +function(try_mklml) + if(NOT ${USE_MKLML}) +return() + endif() + + if(${MKL_FOUND}) +return() + endif() + + message(STATUS "Trying to enable mklml framework due to USE_MKLML") + + include(${CMAKE_CURRENT_LIST_DIR}/DownloadMKLML.cmake) + find_package(MKLML REQUIRED) + include_directories(SYSTEM ${MKLML_INCLUDE_DIRS}) + set(mxnet_LINKER_LIBS ${mxnet_LINKER_LIBS} ${MKLML_LIBRARIES} PARENT_SCOPE) + + set(MKL_FOUND ${MKL_FOUND} PARENT_SCOPE) + set(MKLROOT ${MKLROOT} PARENT_SCOPE) + + set(BLAS MKL PARENT_SCOPE) + +endfunction() + +function(try_accelerate) + if(NOT APPLE) +return() + endif() + + if(BLAS MATCHES "[Mm][Kk][Ll]") +return() + endif() + + if(${USE_ACCELERATE_IF_AVAILABLE}) +message(STATUS "Trying to enable accelerate framework due to USE_ACCELERATE_IF_AVAILABLE") Review comment: Apple Accelerate This is an automated message from the Apache Git Service. To respond to the message, please log on GitHub and use the URL above to go to the specific comment. For queries about this service, please contact Infrastructure at: us...@infra.apache.org With regards, Apache Git Services
[GitHub] aaronmarkham commented on a change in pull request #11148: [MXNET-679] Refactor handling BLAS libraries with cmake
aaronmarkham commented on a change in pull request #11148: [MXNET-679] Refactor handling BLAS libraries with cmake URL: https://github.com/apache/incubator-mxnet/pull/11148#discussion_r202837545 ## File path: CMakeLists.txt ## @@ -38,7 +34,73 @@ mxnet_option(BUILD_CPP_EXAMPLES "Build cpp examples" ON) mxnet_option(INSTALL_EXAMPLES "Install the example source files." OFF) mxnet_option(USE_SIGNAL_HANDLER "Print stack traces on segfaults." OFF) +# Choose BLAS (Basic Linear Algebra Subprograms) computation libraries + +# MXNet supports multiple mathematical backends for computations on the CPU: +# +# * Atlas +# * OpenBLAS +# * MKL (MKL, MKLML, MKLDNN) +# * Apple Accelerate +# +# The default order of choice for the libraries if found follows the path from probably the most +# (recommended) to less performant backends. The order is as follows: +# +# 1. MKLDNN (requires and checks for MKL or MKLML) +# 2. MKL Review comment: (must register, download, and install separately) link to it? This is an automated message from the Apache Git Service. To respond to the message, please log on GitHub and use the URL above to go to the specific comment. For queries about this service, please contact Infrastructure at: us...@infra.apache.org With regards, Apache Git Services
[GitHub] aaronmarkham commented on a change in pull request #11148: [MXNET-679] Refactor handling BLAS libraries with cmake
aaronmarkham commented on a change in pull request #11148: [MXNET-679] Refactor handling BLAS libraries with cmake URL: https://github.com/apache/incubator-mxnet/pull/11148#discussion_r202841125 ## File path: cmake/Modules/FindAccelerate.cmake ## @@ -19,20 +19,44 @@ # # The following are set after configuration is done: # Accelerate_FOUND -# Accelerate_INCLUDE_DIRS +# Accelerate_LAPACK_FOUND +# Accelerate_INCLUDE_DIR # Accelerate_LIBRARIES file(TO_CMAKE_PATH "$ENV{Accelerate_HOME}" Accelerate_HOME) + set(Accelerate_INCLUDE_SEARCH_PATHS - /System/Library/Frameworks/Accelerate.framework/Versions/Current/Frameworks/vecLib.framework/Versions/Current - ${Accelerate_HOME} -) + /System/Library/Frameworks/Accelerate.framework/Versions/Current/Frameworks/vecLib.framework/Versions/Current +${Accelerate_HOME} +) -find_path(Accelerate_CBLAS_INCLUDE_DIR NAMES cblas.h PATHS ${Accelerate_INCLUDE_SEARCH_PATHS} PATH_SUFFIXES Headers) +find_path(Accelerate_CBLAS_INCLUDE_DIR + NAMES cblas.h + PATHS ${Accelerate_INCLUDE_SEARCH_PATHS} + PATH_SUFFIXES Headers) set(LOOKED_FOR Accelerate_CBLAS_INCLUDE_DIR -) +) + +if(Accelerate_NEED_LAPACK) + message(STATUS "Looking for lapack support...") + + # Apples vecLib should contain lapack functionalities included in the Accelerate framework, but we will double check Review comment: Apple's LAPACK This is an automated message from the Apache Git Service. To respond to the message, please log on GitHub and use the URL above to go to the specific comment. For queries about this service, please contact Infrastructure at: us...@infra.apache.org With regards, Apache Git Services
[GitHub] aaronmarkham commented on a change in pull request #11148: [MXNET-679] Refactor handling BLAS libraries with cmake
aaronmarkham commented on a change in pull request #11148: [MXNET-679] Refactor handling BLAS libraries with cmake URL: https://github.com/apache/incubator-mxnet/pull/11148#discussion_r202837409 ## File path: CMakeLists.txt ## @@ -38,7 +34,73 @@ mxnet_option(BUILD_CPP_EXAMPLES "Build cpp examples" ON) mxnet_option(INSTALL_EXAMPLES "Install the example source files." OFF) mxnet_option(USE_SIGNAL_HANDLER "Print stack traces on segfaults." OFF) +# Choose BLAS (Basic Linear Algebra Subprograms) computation libraries + +# MXNet supports multiple mathematical backends for computations on the CPU: +# +# * Atlas +# * OpenBLAS +# * MKL (MKL, MKLML, MKLDNN) +# * Apple Accelerate +# +# The default order of choice for the libraries if found follows the path from probably the most +# (recommended) to less performant backends. The order is as follows: +# +# 1. MKLDNN (requires and checks for MKL or MKLML) +# 2. MKL +# 3. MKLML (downloaded) +# 4. Accelerate (on mac) Review comment: triggered by `apple` correct? Maybe worth putting in each one's param value, so when reading this you're associating the correct values... This is an automated message from the Apache Git Service. To respond to the message, please log on GitHub and use the URL above to go to the specific comment. For queries about this service, please contact Infrastructure at: us...@infra.apache.org With regards, Apache Git Services
[GitHub] aaronmarkham commented on a change in pull request #11148: [MXNET-679] Refactor handling BLAS libraries with cmake
aaronmarkham commented on a change in pull request #11148: [MXNET-679] Refactor handling BLAS libraries with cmake URL: https://github.com/apache/incubator-mxnet/pull/11148#discussion_r202841381 ## File path: cmake/Modules/FindAtlas.cmake ## @@ -18,54 +18,113 @@ # Find the Atlas (and Lapack) libraries # # The following variables are optionally searched for defaults -# Atlas_ROOT_DIR:Base directory where all Atlas components are found -# Atlas_NEED_LAPACK: Whether need lapack libraries +# +# Atlas_ROOT_DIR:Base directory where all Atlas components are found +# Atlas_NEED_LAPACK: Whether need lapack libraries # # The following are set after configuration is done: -# Atlas_FOUND -# Atlas_INCLUDE_DIRS -# Atlas_LIBRARIES -# Atlas_LIBRARYRARY_DIRS +# +# Atlas_FOUND +# Atlas_LAPACK_FOUND +# Atlas_INCLUDE_DIRS +# Atlas_LIBRARIES + +file(TO_CMAKE_PATH "$ENV{CROSS_ROOT}" CROSS_ROOT) + +if(CMAKE_CROSSCOMPILING) + set(Atlas_INCLUDE_SEARCH_PATHS + ${CROSS_ROOT} + ${CROSS_ROOT}/include + ) +endif() set(Atlas_INCLUDE_SEARCH_PATHS - /usr/include/atlas - /usr/include/atlas-base - $ENV{Atlas_ROOT_DIR} - $ENV{Atlas_ROOT_DIR}/include - $ENV{Atlas_ROOT_DIR}/include/atlas -) +$ENV{Atlas_INCLUDE_SEARCH_PATHS} + +$ENV{Atlas_ROOT_DIR} +$ENV{Atlas_ROOT_DIR}/include +$ENV{Atlas_ROOT_DIR}/include/atlas + +/usr/include/atlas +/usr/include/atlas-base +) + +if(CMAKE_CROSSCOMPILING) + set(Atlas_LIB_SEARCH_PATHS + ${CROSS_ROOT} + ${CROSS_ROOT}/lib + ${CROSS_ROOT}/lib64 + ) +endif() set(Atlas_LIB_SEARCH_PATHS - /usr/lib/atlas - /usr/lib/atlas-base - $ENV{Atlas_ROOT_DIR} - $ENV{Atlas_ROOT_DIR}/lib -) +$ENV{Atlas_LIB_SEARCH_PATHS} + +$ENV{Atlas_ROOT_DIR} +$ENV{Atlas_ROOT_DIR}/lib + +/usr/lib/atlas +/usr/lib/atlas-base +) -find_path(Atlas_CBLAS_INCLUDE_DIR NAMES cblas.h PATHS ${Atlas_INCLUDE_SEARCH_PATHS}) -find_library(Atlas_CBLAS_LIBRARY NAMES ptcblas_r ptcblas cblas_r cblas PATHS ${Atlas_LIB_SEARCH_PATHS}) -find_library(Atlas_BLAS_LIBRARY NAMES atlas_r atlas PATHS ${Atlas_LIB_SEARCH_PATHS}) +find_path(Atlas_CBLAS_INCLUDE_DIR + NAMES cblas.h + PATHS ${Atlas_INCLUDE_SEARCH_PATHS}) + +find_library(Atlas_CBLAS_LIBRARY + NAMES ptcblas_r ptcblas cblas_r cblas + PATHS ${Atlas_LIB_SEARCH_PATHS}) +find_library(Atlas_BLAS_LIBRARY + NAMES atlas_r atlas + PATHS ${Atlas_LIB_SEARCH_PATHS}) set(LOOKED_FOR - Atlas_CBLAS_INCLUDE_DIR +Atlas_CBLAS_INCLUDE_DIR - Atlas_CBLAS_LIBRARY - Atlas_BLAS_LIBRARY -) +Atlas_CBLAS_LIBRARY +Atlas_BLAS_LIBRARY +) if(Atlas_NEED_LAPACK) - find_path(Atlas_CLAPACK_INCLUDE_DIR NAMES clapack.h PATHS ${Atlas_INCLUDE_SEARCH_PATHS}) - find_library(Atlas_LAPACK_LIBRARY NAMES alapack_r alapack lapack_atlas PATHS ${Atlas_LIB_SEARCH_PATHS}) - set(LOOKED_FOR ${LOOKED_FOR} Atlas_CLAPACK_INCLUDE_DIR Atlas_LAPACK_LIBRARY) -endif(Atlas_NEED_LAPACK) + message(STATUS "Looking for lapack support...") Review comment: LAPACK This is an automated message from the Apache Git Service. To respond to the message, please log on GitHub and use the URL above to go to the specific comment. For queries about this service, please contact Infrastructure at: us...@infra.apache.org With regards, Apache Git Services
[GitHub] aaronmarkham commented on a change in pull request #11148: [MXNET-679] Refactor handling BLAS libraries with cmake
aaronmarkham commented on a change in pull request #11148: [MXNET-679] Refactor handling BLAS libraries with cmake URL: https://github.com/apache/incubator-mxnet/pull/11148#discussion_r202838327 ## File path: CMakeLists.txt ## @@ -38,7 +34,73 @@ mxnet_option(BUILD_CPP_EXAMPLES "Build cpp examples" ON) mxnet_option(INSTALL_EXAMPLES "Install the example source files." OFF) mxnet_option(USE_SIGNAL_HANDLER "Print stack traces on segfaults." OFF) +# Choose BLAS (Basic Linear Algebra Subprograms) computation libraries + +# MXNet supports multiple mathematical backends for computations on the CPU: +# +# * Atlas +# * OpenBLAS +# * MKL (MKL, MKLML, MKLDNN) +# * Apple Accelerate +# +# The default order of choice for the libraries if found follows the path from probably the most +# (recommended) to less performant backends. The order is as follows: +# +# 1. MKLDNN (requires and checks for MKL or MKLML) +# 2. MKL +# 3. MKLML (downloaded) +# 4. Accelerate (on mac) +# 5. OpenBLAS +# +# Intel's MKL (Math Kernel Library) is one of the most powerful math libraries +# https://software.intel.com/en-us/mkl +# +# It has following flavours: + +# * MKL is a full library, containing all the functionality. It is free under +# community support licensing (https://software.intel.com/en-us/articles/free-mkl), +# but needs to be downloaded and installed manually. +# +# * MKLML is a subset of MKL. It contains smaller number of functions to reduce the +# size of the download and reduce the number of dynamic libraries user needs. This +# is the most effective option since it can be downloaded and installed automatically +# by the cmake script (see cmake/DownloadMKLML.cmake). +# +# * MKLDNN is a separate open-source library, but it requires MKL or MKLML. It is +# shipped as a subrepo with MXNet source code (see 3rdparty/mkldnn). +# +# Since the full MKL library is almost always faster than any other it's turned on by default, +# however it needs to be downloaded and installed manually before doing cmake configuration. +mxnet_option(USE_MKL_IF_AVAILABLE "Use MKL if found" ON) + +# If the full MKL library could not be found the thinner subset MKLML will be downloaded +# unless switched off explicitly. +mxnet_option(USE_MKLML "Use MKLML subset of MKL instead of mkl, will be downloaded" ON) + +# If either MKL of MKLML is present MKLDNN can be utilised from the 3rdparty/mkldnn subrepo. +# See more information here: https://github.com/intel/mkl-dnn +mxnet_option(USE_MKLDNN "Use MKLDNN (requires MKLML or MKL)" ON) + +# Apple's mathematical framework, probably the best choice on a Mac if MKL/MKLML/MKLDNN +# are not available. +# https://developer.apple.com/documentation/accelerate +mxnet_option(USE_ACCELERATE_IF_AVAILABLE "Use Apple Accelerate framework if found, \ Review comment: I get it now, after digging in, but on first glance, I would confuse this with a generic acceleration option, and say, "YEAH! Turn that one on for sure!", and not realize it is for Apple. What if you called it USE_APPLE_ACCELERATE_IF_AVAILABLE? This is an automated message from the Apache Git Service. To respond to the message, please log on GitHub and use the URL above to go to the specific comment. For queries about this service, please contact Infrastructure at: us...@infra.apache.org With regards, Apache Git Services
[GitHub] aaronmarkham commented on a change in pull request #11148: [MXNET-679] Refactor handling BLAS libraries with cmake
aaronmarkham commented on a change in pull request #11148: [MXNET-679] Refactor handling BLAS libraries with cmake URL: https://github.com/apache/incubator-mxnet/pull/11148#discussion_r202839704 ## File path: cmake/ChooseBlas.cmake ## @@ -16,43 +16,199 @@ # under the License. set(BLAS "Open" CACHE STRING "Selected BLAS library") -set_property(CACHE BLAS PROPERTY STRINGS "Atlas;Open;MKL") +set_property(CACHE BLAS PROPERTY STRINGS "Atlas;Open;MKL;Apple") -if(USE_MKL_IF_AVAILABLE) - if(NOT MKL_FOUND) +function(switch_lapack enable) + if(${enable}) +message(STATUS "Enabling lapack functionality") Review comment: LAPACK This is an automated message from the Apache Git Service. To respond to the message, please log on GitHub and use the URL above to go to the specific comment. For queries about this service, please contact Infrastructure at: us...@infra.apache.org With regards, Apache Git Services
[GitHub] aaronmarkham commented on a change in pull request #11148: [MXNET-679] Refactor handling BLAS libraries with cmake
aaronmarkham commented on a change in pull request #11148: [MXNET-679] Refactor handling BLAS libraries with cmake URL: https://github.com/apache/incubator-mxnet/pull/11148#discussion_r202841654 ## File path: cmake/Modules/FindOpenBLAS.cmake ## @@ -15,77 +15,133 @@ # specific language governing permissions and limitations # under the License. +# Find the OpenBLAS libraries +# +# The following variables are optionally searched for defaults +# +# OpenBLAS_HOME:Base directory where all OpenBLAS components are found +# OpenBLAS_NEED_LAPACK: Whether need lapack libraries +# +# The following are set after configuration is done: +# +# OpenBLAS_FOUND +# OpenBLAS_LAPACK_FOUND +# OpenBLAS_INCLUDE_DIRS +# OpenBLAS_LIBRARIES + file(TO_CMAKE_PATH "$ENV{OpenBLAS_HOME}" OpenBLAS_HOME) file(TO_CMAKE_PATH "$ENV{OpenBLAS}" OpenBLAS_DIR) +file(TO_CMAKE_PATH "$ENV{CROSS_ROOT}" CROSS_ROOT) + +if(CMAKE_CROSSCOMPILING) + set(OpenBLAS_INCLUDE_SEARCH_PATHS + ${CROSS_ROOT} + ${CROSS_ROOT}/include + ) +endif() + +set(OpenBLAS_INCLUDE_SEARCH_PATHS +${OpenBLAS_INCLUDE_SEARCH_PATHS} + +${OpenBLAS_HOME} +${OpenBLAS_HOME}/include + +/usr/include +/usr/include/openblas +/usr/include/openblas-base +/usr/local/include +/usr/local/include/openblas +/usr/local/include/openblas-base +/opt/OpenBLAS/include +/usr/local/opt/openblas/include + +${PROJECT_SOURCE_DIR}/3rdparty/OpenBLAS/include +${PROJECT_SOURCE_DIR}/thirdparty/OpenBLAS/include +) + +if(CMAKE_CROSSCOMPILING) + set(Open_BLAS_LIB_SEARCH_PATHS + ${CROSS_ROOT} + ${CROSS_ROOT}/include + ) +endif() + +set(OpenBLAS_LIB_SEARCH_PATHS +${OpenBLAS_LIB_SEARCH_PATHS} + +${OpenBLAS_DIR} +${OpenBLAS_DIR}/lib +${OpenBLAS_DIR}/lib64 +${OpenBLAS_HOME} +${OpenBLAS_HOME}/lib +${OpenBLAS_HOME}/lib64 -SET(Open_BLAS_INCLUDE_SEARCH_PATHS - /usr/include - /usr/include/openblas - /usr/include/openblas-base - /usr/local/include - /usr/local/include/openblas - /usr/local/include/openblas-base - /opt/OpenBLAS/include - /usr/local/opt/openblas/include - ${PROJECT_SOURCE_DIR}/3rdparty/OpenBLAS/include - ${PROJECT_SOURCE_DIR}/thirdparty/OpenBLAS/include - ${OpenBLAS_HOME} - ${OpenBLAS_HOME}/include -) - -SET(Open_BLAS_LIB_SEARCH_PATHS -/lib/ -/lib/openblas-base -/lib64/ -/usr/lib -/usr/lib/openblas-base -/usr/lib64 -/usr/local/lib -/usr/local/lib64 -/opt/OpenBLAS/lib -/usr/local/opt/openblas/lib -${PROJECT_SOURCE_DIR}/3rdparty/OpenBLAS/lib -${PROJECT_SOURCE_DIR}/thirdparty/OpenBLAS/lib - ${OpenBLAS_DIR} - ${OpenBLAS_DIR}/lib -${OpenBLAS_HOME} -${OpenBLAS_HOME}/lib - ) - -FIND_PATH(OpenBLAS_INCLUDE_DIR NAMES cblas.h PATHS ${Open_BLAS_INCLUDE_SEARCH_PATHS}) -FIND_LIBRARY(OpenBLAS_LIB NAMES openblas PATHS ${Open_BLAS_LIB_SEARCH_PATHS}) -IF(NOT OpenBLAS_LIB) - FIND_FILE(OpenBLAS_LIB NAMES libopenblas.dll.a PATHS ${Open_BLAS_LIB_SEARCH_PATHS}) -ENDIF() - -SET(OpenBLAS_FOUND ON) - -#Check include files -IF(NOT OpenBLAS_INCLUDE_DIR) -SET(OpenBLAS_FOUND OFF) -MESSAGE(STATUS "Could not find OpenBLAS include. Turning OpenBLAS_FOUND off") -ENDIF() - -#Check libraries -IF(NOT OpenBLAS_LIB) -SET(OpenBLAS_FOUND OFF) -MESSAGE(STATUS "Could not find OpenBLAS lib. Turning OpenBLAS_FOUND off") -ENDIF() - -IF (OpenBLAS_FOUND) - IF (NOT OpenBLAS_FIND_QUIETLY) -MESSAGE(STATUS "Found OpenBLAS libraries: ${OpenBLAS_LIB}") -MESSAGE(STATUS "Found OpenBLAS include: ${OpenBLAS_INCLUDE_DIR}") - ENDIF (NOT OpenBLAS_FIND_QUIETLY) -ELSE (OpenBLAS_FOUND) - IF (OpenBLAS_FIND_REQUIRED) -MESSAGE(FATAL_ERROR "Could not find OpenBLAS") - ENDIF (OpenBLAS_FIND_REQUIRED) -ENDIF (OpenBLAS_FOUND) - -MARK_AS_ADVANCED( +/lib/ +/lib/openblas-base +/lib64/ +/usr/lib +/usr/lib/openblas-base +/usr/lib64 +/usr/local/lib +/usr/local/lib64 +/opt/OpenBLAS/lib +/usr/local/opt/openblas/lib + +${PROJECT_SOURCE_DIR}/3rdparty/OpenBLAS/lib +${PROJECT_SOURCE_DIR}/thirdparty/OpenBLAS/lib +) + +find_path(OpenBLAS_INCLUDE_DIR + NAMES cblas.h + PATHS ${OpenBLAS_INCLUDE_SEARCH_PATHS}) +find_library(OpenBLAS_LIBRARY + NAMES libopenblas.a openblas libopenblas.dll.a libopenblas.dll + PATHS ${OpenBLAS_LIB_SEARCH_PATHS}) + +set(LOOKED_FOR OpenBLAS_INCLUDE_DIR -OpenBLAS_LIB -OpenBLAS -) +OpenBLAS_LIBRARY +) + +if(OpenBLAS_NEED_LAPACK) + message(STATUS "Looking for lapack support...") Review comment: LAPACK This is an automated message from the Apache Git Service. To respond to the message, please log on GitHub and use the URL above to go to the specific comment. For queries about this service, please contact Infrastructure at: us...@infra.apache.org With
[GitHub] aaronmarkham commented on a change in pull request #11148: [MXNET-679] Refactor handling BLAS libraries with cmake
aaronmarkham commented on a change in pull request #11148: [MXNET-679] Refactor handling BLAS libraries with cmake URL: https://github.com/apache/incubator-mxnet/pull/11148#discussion_r202837162 ## File path: CMakeLists.txt ## @@ -38,7 +34,73 @@ mxnet_option(BUILD_CPP_EXAMPLES "Build cpp examples" ON) mxnet_option(INSTALL_EXAMPLES "Install the example source files." OFF) mxnet_option(USE_SIGNAL_HANDLER "Print stack traces on segfaults." OFF) +# Choose BLAS (Basic Linear Algebra Subprograms) computation libraries + +# MXNet supports multiple mathematical backends for computations on the CPU: +# +# * Atlas +# * OpenBLAS +# * MKL (MKL, MKLML, MKLDNN) +# * Apple Accelerate +# +# The default order of choice for the libraries if found follows the path from probably the most Review comment: probably? This is an automated message from the Apache Git Service. To respond to the message, please log on GitHub and use the URL above to go to the specific comment. For queries about this service, please contact Infrastructure at: us...@infra.apache.org With regards, Apache Git Services
[GitHub] eric-haibin-lin commented on issue #11218: Feature request: randint operator
eric-haibin-lin commented on issue #11218: Feature request: randint operator URL: https://github.com/apache/incubator-mxnet/issues/11218#issuecomment-405398805 @mseth25 This is an automated message from the Apache Git Service. To respond to the message, please log on GitHub and use the URL above to go to the specific comment. For queries about this service, please contact Infrastructure at: us...@infra.apache.org With regards, Apache Git Services
[GitHub] aaronmarkham commented on a change in pull request #11148: [MXNET-679] Refactor handling BLAS libraries with cmake
aaronmarkham commented on a change in pull request #11148: [MXNET-679] Refactor handling BLAS libraries with cmake URL: https://github.com/apache/incubator-mxnet/pull/11148#discussion_r202841167 ## File path: cmake/Modules/FindAccelerate.cmake ## @@ -19,20 +19,44 @@ # # The following are set after configuration is done: # Accelerate_FOUND -# Accelerate_INCLUDE_DIRS +# Accelerate_LAPACK_FOUND +# Accelerate_INCLUDE_DIR # Accelerate_LIBRARIES file(TO_CMAKE_PATH "$ENV{Accelerate_HOME}" Accelerate_HOME) + set(Accelerate_INCLUDE_SEARCH_PATHS - /System/Library/Frameworks/Accelerate.framework/Versions/Current/Frameworks/vecLib.framework/Versions/Current - ${Accelerate_HOME} -) + /System/Library/Frameworks/Accelerate.framework/Versions/Current/Frameworks/vecLib.framework/Versions/Current +${Accelerate_HOME} +) -find_path(Accelerate_CBLAS_INCLUDE_DIR NAMES cblas.h PATHS ${Accelerate_INCLUDE_SEARCH_PATHS} PATH_SUFFIXES Headers) +find_path(Accelerate_CBLAS_INCLUDE_DIR + NAMES cblas.h + PATHS ${Accelerate_INCLUDE_SEARCH_PATHS} + PATH_SUFFIXES Headers) set(LOOKED_FOR Accelerate_CBLAS_INCLUDE_DIR -) +) + +if(Accelerate_NEED_LAPACK) + message(STATUS "Looking for lapack support...") + + # Apples vecLib should contain lapack functionalities included in the Accelerate framework, but we will double check + # https://developer.apple.com/documentation/accelerate/veclib?changes=_2 + include(CheckFunctionExists) + set(CMAKE_REQUIRED_LIBRARIES "-framework Accelerate") + check_function_exists("cgees_" LAPACK_FOUND) + + if(LAPACK_FOUND) +set(Accelerate_LAPACK_FOUND True) +message(STATUS "Lapack found") Review comment: LAPACK This is an automated message from the Apache Git Service. To respond to the message, please log on GitHub and use the URL above to go to the specific comment. For queries about this service, please contact Infrastructure at: us...@infra.apache.org With regards, Apache Git Services